Data Extraction and Modelling from Plant Trait Literature
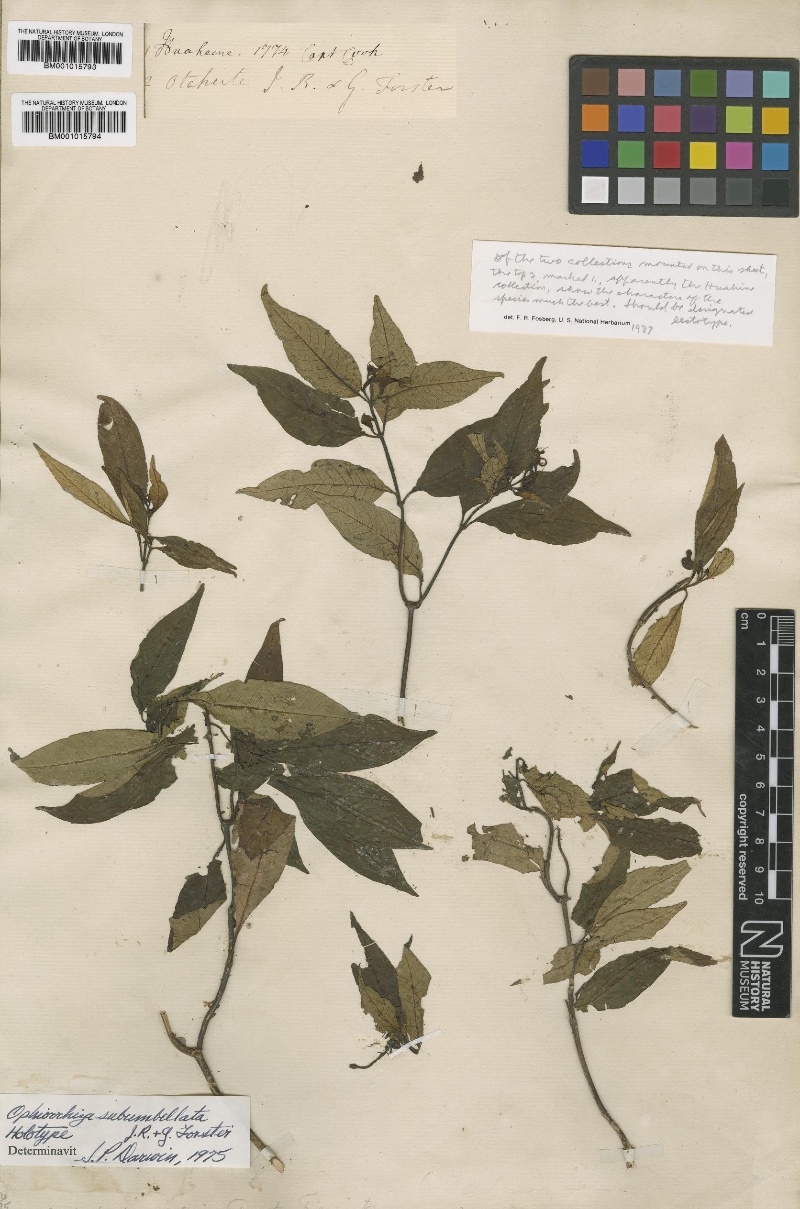
PI and co-PIs: Richard Reeve (University of Glasgow); Neil A. Brummitt (Natural History Museum); Claire L. Harris (Biomathematics and Statistics Scotland); Ana Claudia Araujo (Natural History Museum); Ben Scott (Natural History Museum); Christina Cobbold (University of Glasgow); Glenn Marion (Biomathematics & Statistics Scotland)
Funding amount: $117,500
Project overview: Climate change is significantly affecting plant biodiversity, but it is challenging to monitor climate effects across a multitude of species. This project aims to create a comprehensive global database of plant traits by utilizing computer vision and natural language processing to parse data from both museum collections and the ecology literature, linked to climate and habitat. Data for missing species will also be inferred using taxonomic relationships. This database will provide essential information to improve global biodiversity-climate models, in turn contributing to policy efforts to preserve biodiversity in the face of changing climatic conditions.
Full abstract:
Click to expand
Plants are at the heart of our ecosystems and their complex two-way interactions with climate are an important factor in our ability to predict and mitigate future climate change. We propose to build a framework for extracting and imputing a comprehensive plant trait dataset, which would underpin the next generation of biodiversity-climate models. The big data revolution in ecology has resulted in an enormous amount of information on the distribution and evolutionary history of plant species, which can be combined with historical climate reconstructions to identify their global climate niches. However, the geographic scope of data has been limited and key quantitative traits missing. A wealth of information on plant functional traits is locked away in natural history collections but is now becoming accessible through digitisation efforts. This information is necessary for both global and local predictive models of species distributions that are crucial both to predict the impact of climate change on biodiversity, and to explore the feedback between global vegetation patterns and climate. With the aid of Machine Learning (ML) and these novel botanical datasets we will produce a comprehensive global database of qualitative and quantitative plant functional traits related to climate and habitat. This will involve two concurrent approaches: firstly, deriving quantitative functional trait information from ecological literature and imaged specimens, using Natural Language Processing (NLP) and Image Recognition software and, secondly, exploiting different approaches for trait imputation and gap filling in mined plant traits, using both ML and traditional phylogenetic techniques.
Ecosystems & Biodiversity Climate Science & Modeling Data Mining Natural Language Processing