Estimating the Ice Volume of All Glaciers in High Mountain Asia With Deep Learning
With the support of the Innovation Grants Program, the ICENET project combines remote sensing and deep learning to assess the ice volume of all glaciers in High Mountain Asia.
Estimating the ice volume of the Earth’s glaciers is a grand challenge of Earth system science. Besides being a critical parameter to model glacier evolution, knowledge of glacier volumes is fundamental to forecasting global sea level rise as well as available freshwater resources.
Glaciers are very sensitive to climate change. Under atmospheric warming induced by human forcing, with few exceptions glaciers have been retreating worldwide at unprecedented rates. Glacier melting is estimated to be responsible for 22% of the sea level rise observed during 1971-2018, with far reaching implications for the human population living in coastal areas.
Ice mass loss from glacier shrinkage has impacts on water availability for an estimated global population of 1.9 billion people living in or depending on glacier sourced freshwater .
In the Himalayan region in particular, melting glaciers poses a threat to water security for more than 1.4 billion people who depend on glacial basins for water , in addition to exacerbating the risk of floods that are wrecking the region, driven by increasingly more powerful monsoon rainfall. Overall, the future pathways for freshwater availability are uncertain and heavily depend on the upcoming climate trajectories, making improved glacier ice volume estimates in this region an urgent priority.
In a recent study, a group of researchers reported that the total glacial ice volume in High Mountain Asia is 37% higher than the previous consensus value, highlighting the challenges of traditional glacier modeling approaches and the need for more accurate and less uncertain ice volume reconstructions. With ICENET we explore the potential of the immense pool of satellite images and recent advances in deep neural networks to advance the knowledge of glacier ice volume in High Mountain Asia.
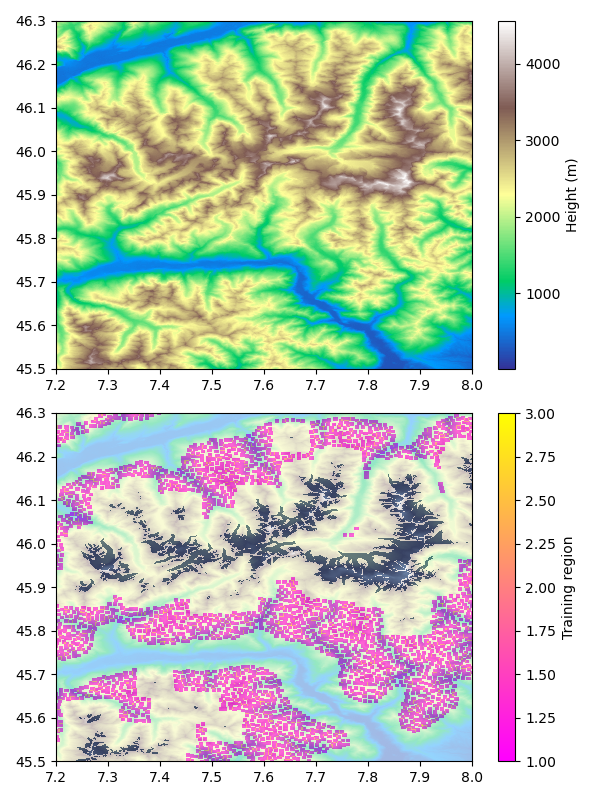
Remote sensors mounted on satellites have been silently monitoring the extent and changes of the glaciated surfaces of the Earth for decades. The accumulated vast amount of data produced by these instruments are difficult to exploit by traditional data-analysis pipelines, and at the same time the perfect playground for neural networks. Among the products delivered by satellite sensors is data of the Earth’s surface elevation, including glaciers. To calculate their ice volume, however, the missing piece of information is the bedrock topography, i.e. the spatial distribution of the elevation of the Earth’s crust beneath glaciated regions. Our model will attempt to infer such glacier bedrock topographies. As an elevation satellite product, we use 1-dimensional digital elevation maps of the Earth surface.
We approach the problem leveraging generative deep inpainting architectures trained on extensive portions of de-glaciated mountain regions. Inpainting networks are used to fill missing patches within an image. In our case the training images are such elevation maps of mountain regions, while the missing patches, i.e. the targets of the network, are represented by the glaciated areas. The network will thus be trained to reconstruct glacier underlying topographies, and in turn their volume by the difference with the surface elevation.
With the generative models developed over the course of the ICENET project, we hope to reduce the current uncertainties of ice volumes in High Mountain Asia, and at the same time advance knowledge of glacier modeling with the help of AI techniques.
The Team
The Institute of Polar Sciences (CNR-ISP, Venice, Italy) is part of the Italian National Research Council. The mission of the ISP is to improve our understanding of the climatic changes taking place in the Arctic and Antarctic environments and possible future developments at both polar and global levels. Our studies address research issues related to both the chemical/geochemical and physical aspects of the poles using a multidisciplinary approach to protect these vulnerable extreme environments. ISP also has a long standing record of research on mid-latitude glaciers, especially in the European alpine region.
The Department of Earth System Science (ESS) at the University of California, Irvine focuses on how the atmosphere, land, and oceans interact as a system, and how the Earth will change over a human lifetime. At ESS, researchers combine observations from remote sensing platforms and field data with numerical modeling to understand the physical processes controlling the response of the ice sheets to climate change, and to reduce the uncertainties of projections of the future contributions of the Greenland and Antarctic Ice Sheets to sea level rise regionally and globally over the coming centuries.