The Green Last Mile Project: Accelerating Cargo-Bike Logistics in Cities
As part of the CCAI Innovation Grants Program, a team from Denmark, India, the US and the UK is working on simulations of vehicle performance across urban micro-regions to accelerate the transition to cargo-bike logistics.
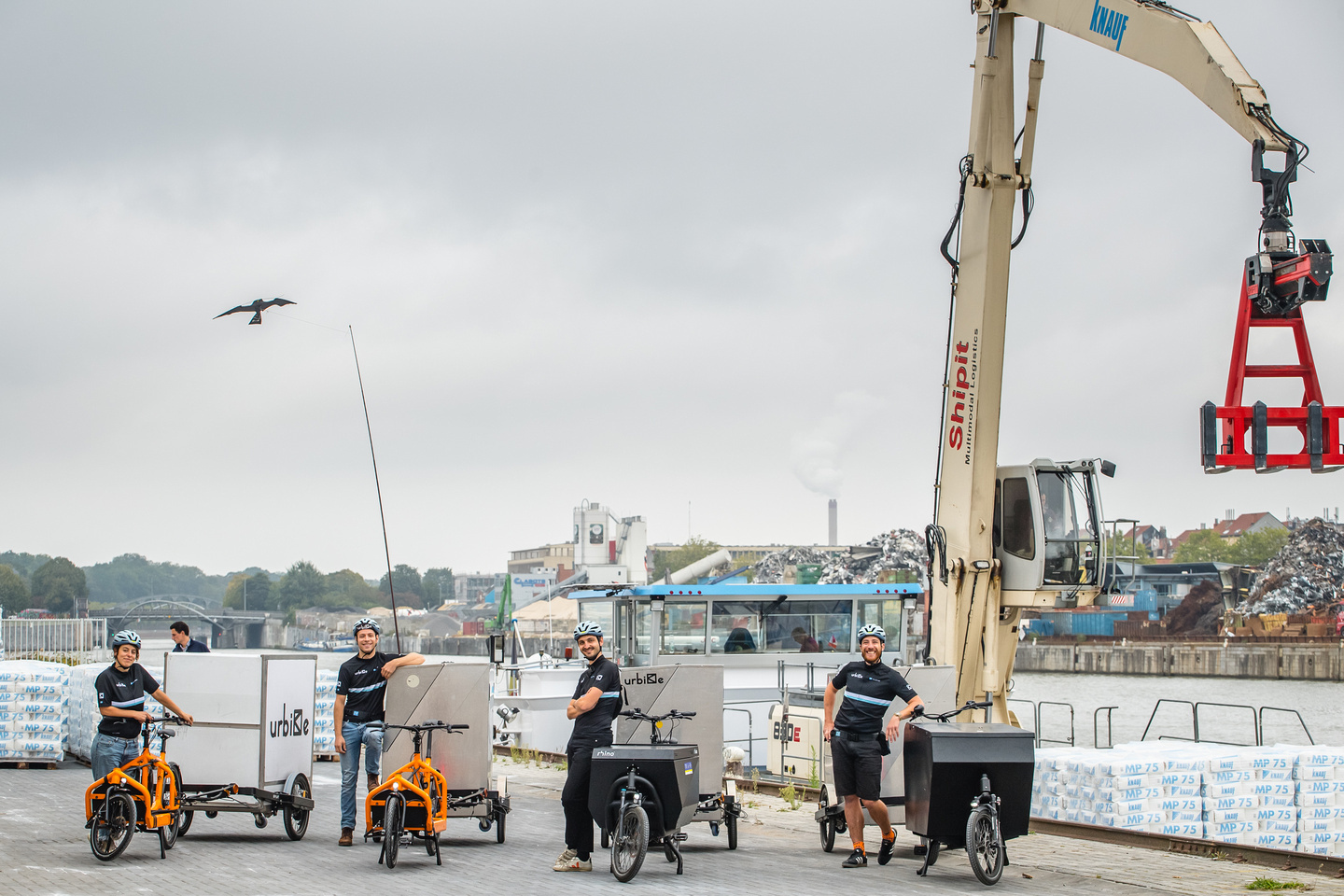
Light goods vehicles (LGV) are one of the leading polluters in cities due to their extensive use in the last mile of delivery. Recently, cargo bike logistics has been put forward as a competitive, zero-emission candidate for replacing LGVs in urban areas, with experts usually estimating that cargo bikes can replace 25-50% of van deliveries. Today the percentage of last mile deliveries made by cargo-bike remains anecdotal, mainly pioneered by a number of smaller logistics operators (our partners Pedal Me and Urbike being prime examples of that), but is gaining increasing attention from the logistics industry.
Cargo-bikes are a multi-solution on the path to sustainable and humane cities. They cut pollution, decongest cities, emit a tenth of the CO2 emitted by an electric van, and take much less space on the roads, all this while moving significantly faster in dense urban areas. Due to their faster speeds, shorter parking times, and more efficient routes across cities, they can out-compete traditional van logistics when operated effectively.
Despite these competitive advantages in dense urban areas, their widespread adoption by logistics operators is limited. This is primarily due to the lack of inexpensive ways of accurately evaluating their impact on the cost of business. By modelling the relative delivery performance of different vehicle types across urban micro-regions, machine learning can help operators evaluate the business and environmental impact of adding cargo-bikes to their fleets.
Our project is developing accurate models of navigation time and service time (e.g. cruising for parking, unloading, walking) for different vehicle types during urban delivery runs. Using Uber’s H3 index to divide the cities into hexagonal cells, and using OpenStreetMap tag data, we are studying how urban context affects delivery performance for different vehicles. Our models will enable key stakeholders to run feasibility studies for optimising and diversifying their fleet composition in a cost-effective manner.
Cycle-logistics as a sustainable solution to urban transport has not been a focus of the machine learning community, where solutions such as autonomous vehicles, delivery drones, and robots get attention and funding, despite their uncertain feasibility and impact.
A significant uptick in cycle logistics would also make transport cycling much safer and thus encourage people to move away from cars through 1) removing vans from the roads, 2) providing commercial incentives for cycling infrastructure and 3) fostering a phenomenon known as ”safety in numbers” (the more people cycle, the safer it is, thus the more people cycle). In cities, 50% of car trips are shorter than a 20min cycle. Shifting away from short car trips could have wider repercussions on transport GHG emissions.
.jpg)
About the Partners
The Green Last Mile Research Group
Our research team is composed of Navish Kumar (IIT, Kharagpur 🇮🇳), Max Schrader (University of Alabama 🇺🇸), Maria Astefanoaei (Dasya Lab, ITU, Copenhagen 🇩🇰), Akash Srivastava (MIT-IBM AI Research Lab, Cambridge, MA, 🇺🇸), Kai Xu (Edinburgh University / Amazon Research, NYC 🇺🇸), Esben Sørig, Soonmyeong Yoon and Nicolas Collignon (Kale Collective, London 🇬🇧). The research is being conducted in partnership with two expert cargo-bike logistics operators, Pedal Me in London 🇬🇧 and Urbike in Brussels 🇧🇪, as well as Larry vs Harry, a leading cargo-bike manufacturer in Copenhagen 🇩🇰.