Bridging the Gap Between Academia and Industry In The Energy Sector
Data science innovation is often lost between Industry and Academia. A new report offers suggestions for improving the process.
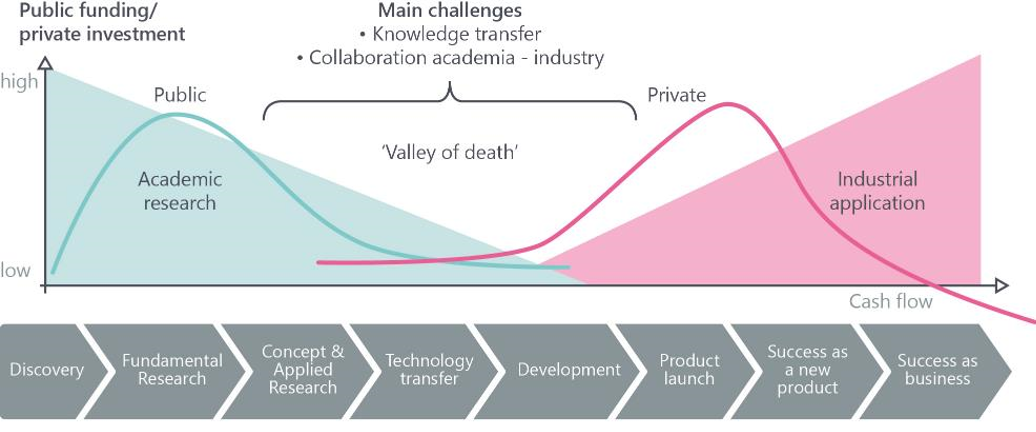
Despite the wealth of cutting-edge research produced in academia, it is difficult to extract these learnings and embed them into the operational, day-to-day activities of industry. This innovation chasm between academia and industry is known as the “Valley of Death,” where many good ideas are lost.
Data science innovation within the energy sector loses many great ideas in the valley of death. In this post, we provide insights from a recent report which investigates the challenges and opportunities in sharing knowledge and skills from academia to industry.
The challenges and opportunities of the valley of death were highlighted to Energy Systems Catapult, a UK not-for-profit technology and innovation centre set up to support innovation in the energy sector, after a meeting with the innovative flexibility providers Arenko Ltd. Arenko, an operator of flexible assets in the UK energy market that focuses on algorithms and automation, had struggled with accessing and reproducing the valuable research contained within academic journals, despite their need to keep up with the latest state-of-the-art models.
Identifying the Valley of Death
To understand the landscape of academic impact in the energy industry, especially within the fast-moving data science domain, we conducted desktop research and interviewed over 30 professionals from both academia and industry. These professionals provided insight into their difficulties in collaborating and transferring knowledge. They also shared insights about what worked well, or poorly, in their interactions across the valley of death.
A common theme in our interviews was disappointment with collaborations between academia and industry. A driving factor in this disappointment is that the two sectors’ timelines and objectives are misaligned. Industry expects relatively short turnover (months), whereas academic projects are usually much longer (years). Additionally, their success measures and criteria are often very different. For example, in academia a common objective is a large number of citations on publications in high-impact journals, whereas industry focuses on other KPIs such as financial targets or levels of engagement.
When developing projects together, academia and industry often don’t fully understand the differences in work culture and end up choosing suboptimal ways of working together. As a result, objectives and outputs deviate from the original proposal, with academics often focusing on more theoretical outputs, leading to less application to the original business case. This creates many missed opportunities, not just for innovation, but for identifying and recruiting the best academic talent.
To supplement the interviews, we also reviewed the literature on a specific area of data science research in energy systems: electricity price forecasting. Arenko’s domain expertise allowed us to understand what parts of these academic publications could be used in industry settings. The literature review showed a disconnect between industry needs and academic practices. The methodologies outlined in most papers are often not clear enough to enable reproduction, and without open data and/or code, it is often virtually impossible to verify the results and methods. For industry practitioners with limited time and resources, these instant roadblocks make it far less likely that a company will apply academic research.
The interviews and the literature review showed an important training gap in coding, a skill set that is desperately needed given the rapid digitalisation of the energy sector. Although data science courses develop rigorous skills in modeling and advanced algorithms there is very little content on applying the latest programming approaches, e.g., code review, structure, or version control. Of ten data science-focused master’s programs from major universities in the UK, we found only two that had dedicated introductory courses to programming and none of them had any dedicated intermediate or advanced courses. In other words, unless self-taught, such graduates entering the workforce will be lacking in the skills to develop operational code in industry teams. Discussions with those in the energy sector have highlighted this as a major difficulty when recruiting new data science talent.
Making Impact
These are just some of the issues we discovered through our research. To better address the long-standing misalignment between academia and industry our reports collate many tools and approaches to support more fruitful and fulfilling academic impact in industry.
One of the most effective, but perhaps difficult, ways to improve collaboration is better understanding of each other’s cultures, businesses, and objectives. Is it more effective to collaborate through master’s projects, PhDs, or postdocs? Can the project be designed to achieve satisfactory outcomes for both sides: publications for academics; useful tools and insight for the company?
One approach to improved collaboration is to move away from short-term projects and develop longer-term strategic relationships between business leaders and academics. This can give clearer objectives for collaboration and support postdoctoral researchers, a valuable and often overlooked academic partner. All too often, postdocs, who are often the most versatile and effective researchers, are given short-term contracts with extremely large workloads. Longer-term contracts will not only attract the best academics but also help maintain continuity and quality in the relationship.
Another recommendation is for academic research to focus on open and reproducible research. All models should be trained on open data sets and verified using common, simple benchmarks. Code should be treated as a valuable research output to incentivise code sharing within academia. This can improve coding skills, serve as an advertisement to prospective employers, and help support reproducible research and innovation. Platforms like Hugging Face which share trained code and data are becoming more popular and should be adopted by the energy data science community.
Data Science Competitions co-organised with industry have proven to be incredibly useful platforms for supporting open science and the development of common benchmarks to give innovators a head start. They can also help identify some of the most powerful methods and input features, helping crowdsource solutions for some of the biggest challenges facing the energy sector. By having participants share repositories, these competitions also encourage the sharing of new and novel data sets.
Finally, applied academic research depends on the real-world challenges and problems facing the industry. Industry plays a key supporting role for new innovative research. Companies must share their experience, challenges, and data if they want research to solve their relevant issues and make a difference in the real world. Industry needs to engage proactively with universities to make sure they are solving the actual problems they are facing and can utilise their domain knowledge and experience.
Similarly, academia must better utilise and listen to industrial experience and knowledge in shaping their courses. Industry can play an important role in developing the coding skills of the future graduates. Software development moves at a faster pace outside of academia, so their input is vital to ensure training that is fit for purpose!
Finding More Information
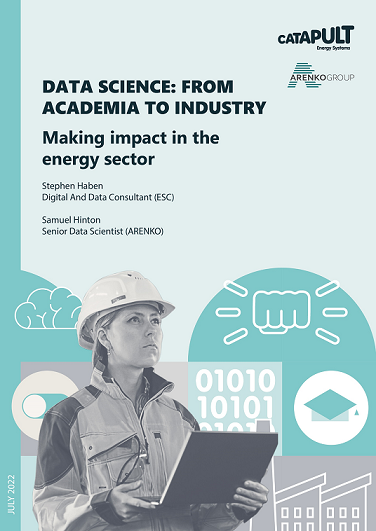
The learnings and challenges we outlined above and more can be found in the series of reports we developed. The main report considers the core aspects and challenges of bringing innovative ideas from academia to industry in data science. Four supplementary reports describe mechanisms which can support this impact: Collaboration, Accessible and Reproducible Research, Code Development for Academics, and Industrial Support for Academia.
We also invited a number of experts in these topics to share their views and insights in a recorded launch event.
We do hope you enjoy these resources and if you are interested in further discussions, or have ideas to extend or enhance this work, we’d love to hear from you! Contact us at Energy Systems Catapult: https://es.catapult.org.uk/contact/