Using Machine Learning to Track International Climate Finance
Researchers used natural language processing to track international climate finance based on textual descriptions of development projects.
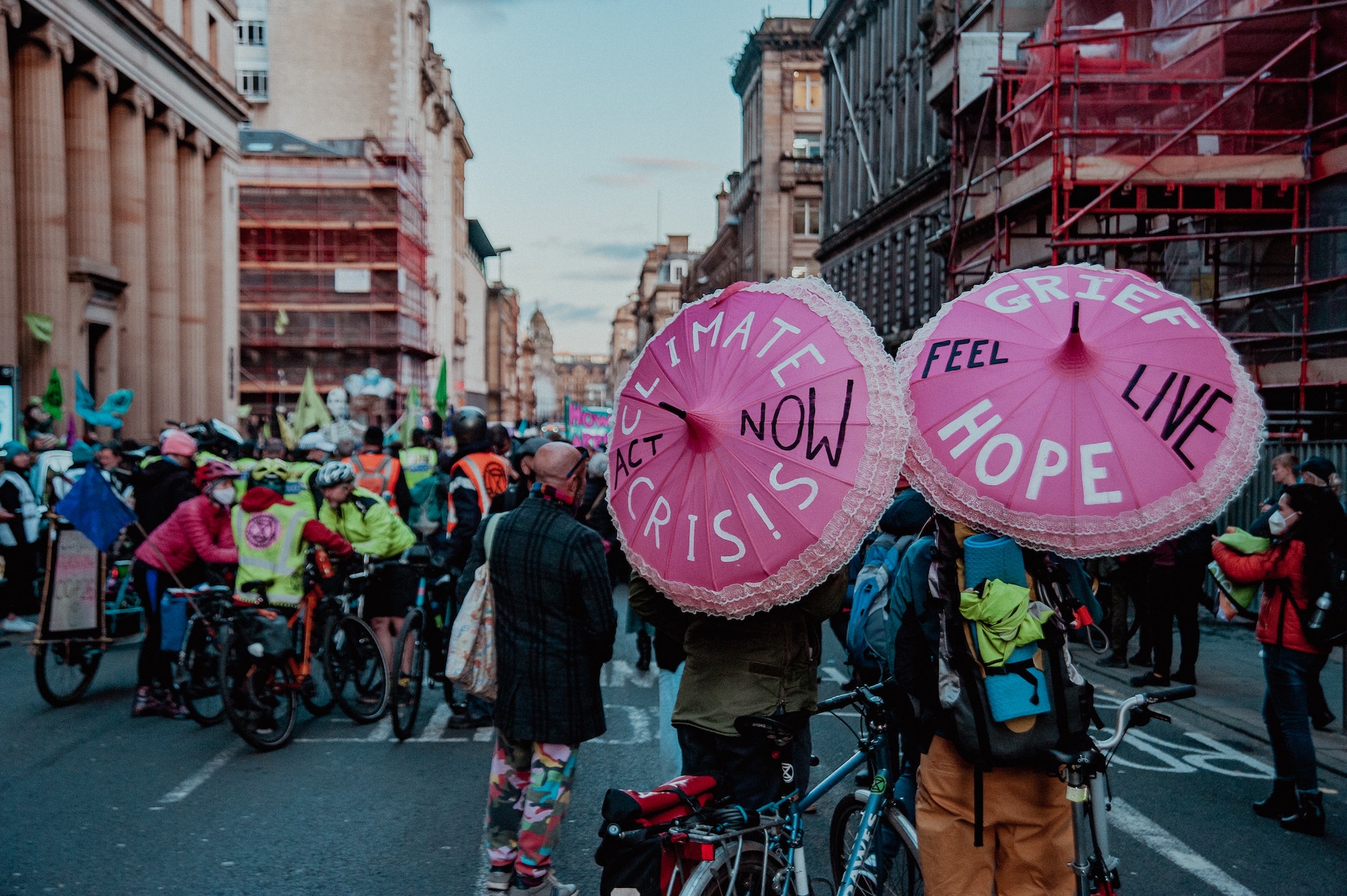
International climate finance is a key ingredient for global action to curb global temperature rises and prepare societies for the already irreversible effects of climate change. At the 2009 Copenhagen summit, high-income countries committed to mobilize US$ 100 billion annually from 2020 onwards to support mitigation and adaptation in developing countries. This promise was a crucial step to get lower and middle income countries to define national emissions reduction targets and led to the Paris Agreement where, for the first time, all countries committed to climate action.
The latest report by the OECD shows that, in 2020, contributor countries failed to meet the US$ 100 billion climate finance target. This weakens trust of recipient countries and complicates the current climate negotiations in Egypt (COP27), where countries are starting to define a new climate finance target for the period after 2025—the “new collective quantified goal.”
Tracking global flows of climate finance is difficult. Countries and institutions self-report the projects and corresponding finances which contribute to the US$ 100 billion target. However, these contributors have different methods for assessing what counts as climate finance, and the reporting process is not transparent. Moreover, researchers and NGOs have found that many projects are misreported and should not be counted as climate finance.
Analyzing project descriptions with natural language processing
In our new study, Florian Egli, Anna Stünzi, and I developed a natural language processing model (ClimateFinanceBERT) that identifies climate finance projects based on their textual descriptions and classifies them into granular climate finance categories (e.g., solar energy or energy efficiency). Using ClimateFinanceBERT, we analyzed 2.7 million descriptions of bilateral development projects from 2000 to 2019. The model classified 80,023 of these projects as climate finance (52% adaptation and 48% mitigation projects), totalling US$ 80 billion.
For the period after the Paris Agreement (2016–2019), our estimates are about 64% lower than the officially reported project assessments. These findings show a great disparity between promised and delivered climate finance and support claims that contributor-reported numbers may be inflated.
The study also provides important take-aways on how machine learning could help address major challenges in climate finance accounting. The machine learning based approach offers three benefits: flexibility regarding the scope of climate finance, consistency in the evaluations of textual descriptions, and scalability that enables cost- and time-effective analysis regardless of the number of documents.
- Flexible scope: ClimateFinanceBERT allows for a flexible definition of climate finance, because its assessment is based on a set of granular categories, like solar energy or energy efficiency. In the paper, we focused on a strict conception of climate finance where we only counted projects with a primary focus on adaptation or mitigation as climate finance. However, our model also categorizes other types of projects with co-benefits for the climate, such as biodiversity or sustainable land use projects. This “bottom-up” approach—using a collection of granular categories instead of a single yes/no classification—offers the flexibility to extend the scope of climate finance by including more categories with co-benefits for the climate.
- Consistent evaluation: ClimateFinanceBERT provides a consistent evaluation of climate finance that can easily be replicated. The current reporting of climate finance lacks such consistency, on the one hand because reporting organizations differ in how they assess climate relevance, and on the other hand because human annotators vary considerably in interpretation and individual judgment. The lack of consistent reporting makes it difficult to compare numbers between different contributors and to provide a transparent and comprehensive picture on global flows in international climate finance.
- Scalable classification: Replacing human evaluations with machine learning classification could open a major bottleneck in climate finance accounting: time and cost. Classifying individual projects via human annotators takes considerable time, which means that evaluation is done only once and, most likely, by only one person. Changing decision paradigms ex-post or double checking individual evaluations is simply too time consuming and expensive. In contrast, machine learning algorithms are scalable. Depending on the hardware, it takes between several minutes and a few hours to classify millions of textual project descriptions with ClimateFinanceBERT.
What role can machine learning play in climate finance accounting?
Despite its benefits, a machine learning-based approach cannot substitute entirely for human evaluations.
Climate finance is complex, and so is the task of classifying it. Projects related to climate change adaptation are highly context-specific. They may require expert knowledge of other external factors, which are not provided in the project descriptions, to classify them correctly. For example, water and sanitation projects are more relevant for climate adaptation in regions affected by droughts.
Furthermore, the complexity of climate finance means machine learning classifiers may misclassify some infrequent and underrepresented types of projects. This risk is intrinsic to supervised classification: a classifier trained on one set of project descriptions must generalize to new descriptions it hasn’t seen during training.
In our study, we trained our classifier on 1,500 climate finance projects and then classified 2.7 million project descriptions. To mitigate blind spots, we:
- used a stratified approach to create our training set by sampling project descriptions from different sectors (e.g., energy, agriculture) and with different climate finance tags by the contributors (e.g., mitigation, adaptation);
- used a model that was already pre-trained on three million climate related texts before training it on project descriptions from international climate finance; and
- conducted extensive validation checks, testing our trained model against existing manually annotated data sets from other climate finance studies and against expert judgment in user studies.
While machine learning will not be able to replace human evaluators, it is a helpful tool that can complement human assessment by double-checking reported projects, simulating outcomes for different climate finance scopes, and analyzing how climate finance is distributed over different sub-categories.
-
Double-checking reported projects: Machine learning models could double-check reported projects and highlight potential misclassifications or relevant projects that have been overlooked by reporting contributors. In our study, we found both substantial over- and underreporting of climate finance projects. Alternative suggestions by the machine learning tool could be manually checked through an expert verification process. Contributors could use the tools to cross-check their own efforts. Overall, algorithmic double-checking could improve trust in the current reporting at minimal cost and effort.
-
Simulating outcomes for different climate finance scopes: Machine learning models could be used to analyze how different scopes of climate finance accounting affect overall financial contributions and relate to climate finance targets. This would create a more nuanced picture of international climate finance, informing negotiations and nurturing climate ambitions.
-
Analyzing climate finance distribution: The bottom-up approach of the machine learning model could help analyze how climate finance is distributed over different sub-categories, such as extreme weather, renewables, or energy efficiency. This would allow contributors, recipient countries, and other stakeholders to identify categories that are under- or overrepresented in international climate finance, helping them coordinate between projects and effectively allocate financial resources.
While machine learning is not a silver bullet, it allows parties such as contributors, recipients, and NGOs to review climate finance contributions based on consistent criteria and a flexible scope. This would enable all parties to discuss targets for climate finance and climate action at eye level. Ultimately, tracking international climate finance with machine learning could help restore trust in the global community, which is essential for agreeing on credible climate finance targets and translating financial support into effective climate action.