Using Machine Learning to Increase Durability and Reduce Returns for Sports and Fashion Goods
Contextualizing Environmental Impacts of Short Life Cycles and High Returns on the Industry
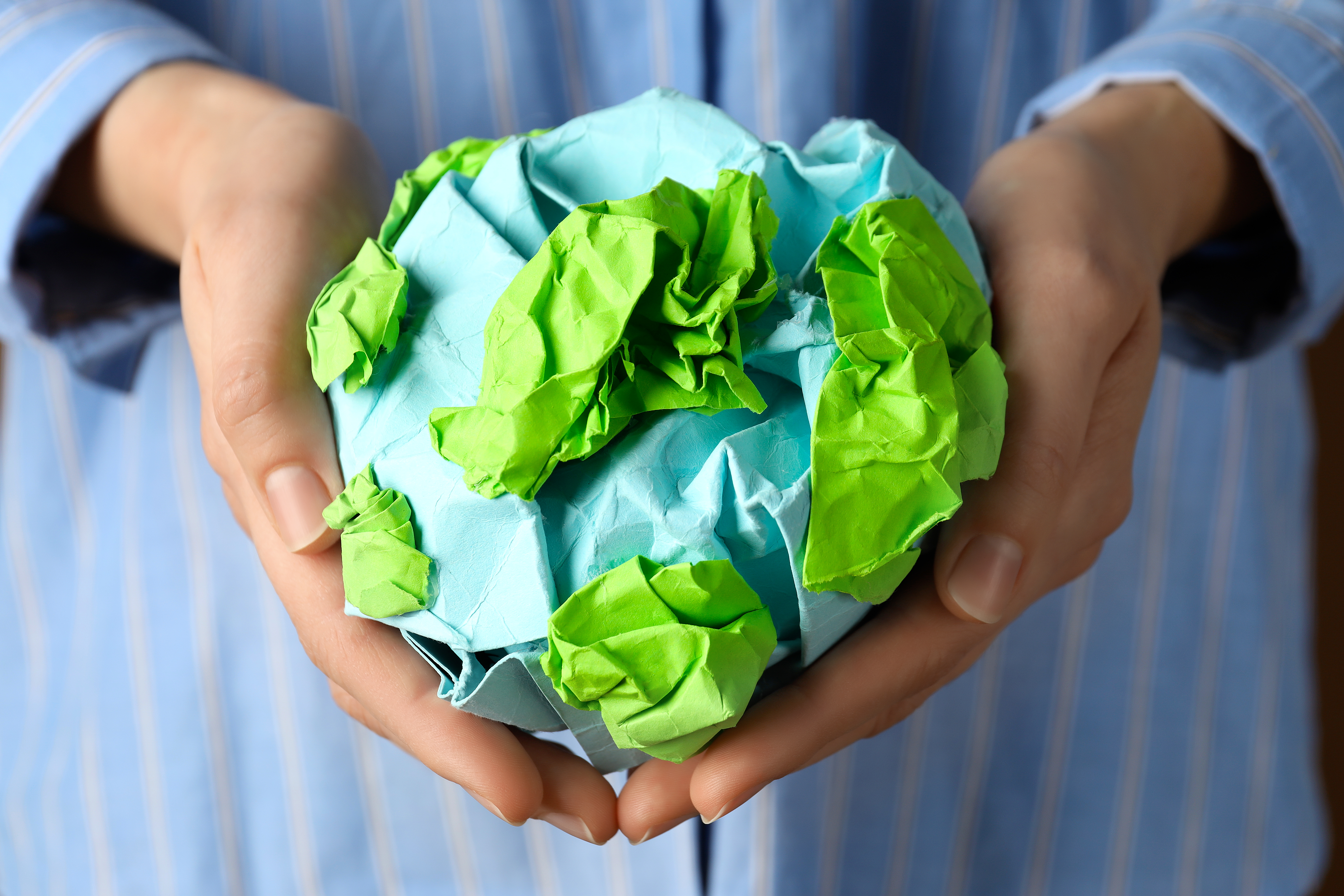
Fashion shapes the way we express ourselves and reflects personal values. However, from haute couture (handmade) to fast fashion, the cost of fashion extends beyond the price tag. This article delves into the far-reaching consequences of fashion products and how overlooked factors such as quality can be combined to significantly reduce their impact on the planet.
A product that breaks quickly or fails to meet consumer expectations has a staggering impact. Durability issues give rise to short lifecycles, resource squandering, and increased landfill waste. Poor designs create unwanted products. Sizing mismatches generate unnecessary emissions through returns. The prevalence of poor quality not only undermines sustainable consumption but also accelerates environmental damage. A report from the European Parliament quantifies the existing impacts of textile production on the environment.
The fashion industry accounts for 4 percent of global GHG emissions, according to McKinsey. According to the World Bank, every year the fashion industry uses 93 billion cubic meters of water; about 20 percent of wastewater worldwide comes from fabric dyeing and treatment, and 87 percent of the fabric is incinerated or disposed of in a landfill. Overall, the textile industry contributes about 10% to global emissions, which, at the current pace, may rise to 50% (according to the World Bank) by 2030.
With the aim of reducing the impact of our industry, a team of data scientists and machine learning engineers gathered together to discover the information which can improve product durability and reduce returns using available data.
Product Reviews to Measure Product Life Cycle and Quality
In this context, we looked at different data sources to gather information on the quality and durability of the product, focusing on aspects that matter most to consumers and capture circularity performance. Circularity metrics at the product level were not available to us, and to our knowledge, there are still many challenges with assessing holistic footprint and circularity performance.
Since we did not have circularity metrics, we focused on proxies such as durability and quality-led returns (understood as the match of consumer expectations and product claims). Durability is key, as the most sustainable product is the one that is not produced, and if we produce products that last two times longer, we are directly reducing our footprint by 50%. Given that many returned products are incinerated or piling up in warehouses, we also sought data sources that could help us understand the reasons for product returns so we can minimize them.
Our available data to capture information about prodcut returns included product test data, expert reviews, and product reviews. Product test data provides valuable insights into the internal testing done on a product under lab conditions; however, the problem is that it is not systematically stored and does not give us visibility into the reasons for returns. Expert reviews provide feedback on the product across all relevant dimensions (versatility, durability, fit, etc.) but rarely cover most products (only key franchises), and it does not necessarily match what actual consumers report about the products. Our preferred option was, therefore, the product reviews from actual consumers of the products, as it gives us a good understanding of the positive and negative features of the product, as well as the reasons for return or a short life cycle. In our analysis, we were able to cover more than 80% of the revenue of the products we want to analyze with English language product reviews, and expanding the language scope would lead to more than 95% product coverage. For this reason, we chose product reviews as the data source to measure product aspect sentiment.
This post proposes a novel approach using public product review data. By using Natural Language Processing, consumer feedback can be extracted, analyzed, and grouped by key topics or aspects to gain remarkable insights.
How Aspect-Based Sentiment Analysis Can Improve Product Design and Consumption Decisions
In particular, we will use Aspect-Based Sentiment Analysis (ABSA) to extract consumer sentiments from reviews. The framework leverages pretrained or fine-tuned language models to detect word relationships in the text, which is later used to map aspects and sentiments. We were interested in analysing dimensions(from now on referred to as ‘aspects’) that truly matter both for product footprint and consumer satisfaction.
Although ABSA is able to find most of the aspects available in the reviews without customization, we choose to limit the aspects to a predefined list due to the diversity of consumer experiences with a product. Based on product expertise, six key categories, or quality aspects, were defined. They cover most of the topics reported by consumers found in our data.
The Quality Aspects are:
- Fit
- Design
- Material
- Comfort
- Durability
- Color
A score is calculated for each of the above aspects. This score is calculated by analyzing the sentiment associated with every predefined keyword using the pyabsa (Python Aspect-Based Sentiment Analysis) framework. These sentiments are then amalgamated to create a score for each aspect. With this information, we can detect products that have been underperforming and understand why. These underperforming products need immediate attention by either fixing the underlying problems or improving the product in the next release.
The spider plot below gives a visual comparison of two products using the Quality Aspects.
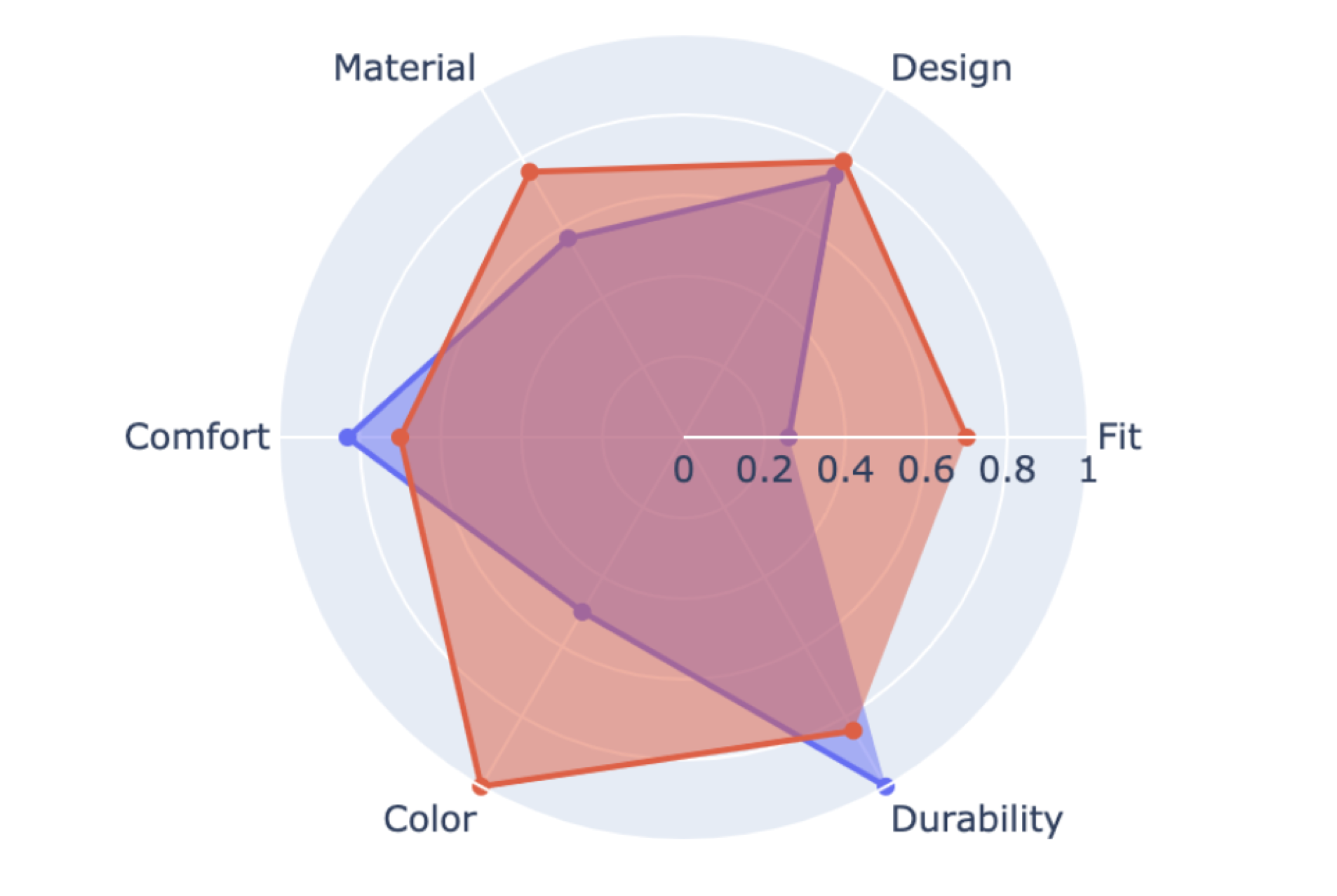
In this example, the product in red is superior in almost every dimension, particularly fit and color, while not substantially different in the others. One can also see that the blue product design cycle should focus on fit and color problems.
These scores for each metric can be used in a number of ways to tackle environmental challenges.
Fit:
Helps by reducing returns and minimizing the need for shipping back and forth due to sizing issues, decreasing the carbon footprint associated with transportation and packaging materials.
Accurate sizing reduces the need for extra production and inventory, which, in turn, lowers manufacturing emissions and resource consumption.
Design:
Designing products that have timeless appeal and can withstand changing trends reduces the frequency of product turnover, resulting in fewer items becoming obsolete and contributing to landfill waste.
Longer-lasting designs mean fewer resources are used for constant redesign and production, ultimately reducing emissions linked to manufacturing processes.
Material:
Material score helps with choosing durable and sustainable materials that can extend the product’s lifespan, reducing the need for frequent replacements.
Sustainable material replacements can also have a smaller footprint during production, as they might require less energy or fewer chemicals in their manufacturing processes.
Comfort:
Comfortable products lead to longer usage and reduced turnover. Consumers are less likely to discard a comfortable item, decreasing the frequency of purchases and waste.
Longer product lifespans mean fewer products need to be manufactured, cutting down on resource consumption and associated emissions.
Color:
Offering classic colors that stand the test of time can reduce the “fast fashion” cycle, where colors fall out of style quickly, leading to frequent discards.
Fewer color variations and shorter design cycles can lessen the strain on manufacturing facilities and reduce emissions related to production.
Durability:
Producing durable products that withstand wear and tear means consumers won’t need to replace them as often, reducing the overall demand for new products.
Durable products require fewer replacements, which lowers the demand for manufacturing new items and the associated emissions from production processes.
These metrics provide invaluable insights into product quality, offering opportunities to reduce environmental harm, enhance future product development, and curtail the overproduction of unwanted items. When combined with other performance indicators like return rates, these insights not only contribute to environmental sustainability but also bolster business efficiency.
Our analysis of these quality metrics revealed the following key findings:
- 39% of products achieved a fit score below 80%
- 6% of products received a fit score below 50%
- 2% of products scored below 50% in material quality.
- 80% of products received a material score exceeding 80%.
This shows, in our sample, that there is significant room for improvement in fit, while material is normally well perceived by consumers. There are further insights that can be obtained for a particular product as well as the entire range. These results are shared to illustrate how this data can drive quality and design strategy.
Our Results, Limitations, and Training Resources
This methodology isn’t without its shortcomings and drawbacks. One of the biggest drawbacks is that by the time enough review data is collected, the damage process has already started. We recommend leveraging expert reviews as a proxy when insufficient consumer data is available. Another challenge is that, in many cases, products with issues have already been produced in large quantities due to large lead times and the limited producer capacity. If insights from consumer reviews are coupled with Make to Order strategies and localized manufacturing, it will reduce inventory requirements while increasing agility in the feedback cycle and the potential to enhance quality improvements.
In conclusion, using aspect-based sentiment analysis on consumer reviews offers a promising avenue to increase consumer satisfaction thereby reducing returns and excess inventory, ultimately leading to decreases in the carbon footprints of fashion goods. By harnessing the power of consumer feedback and NLP, we have the potential to not only create better, longer-lasting products but also to mitigate the environmental toll of these products and pave the way for a more sustainable and responsible future.
If you are interested in leveraging this methodology for your research or industry, we have published a notebook with Kaggle data that walks you through an analysis using ABSA.
Our mission
We are a passionate group of data scientists and machine learning engineers committed to using AI to reduce product footprint and improve product decision-making.
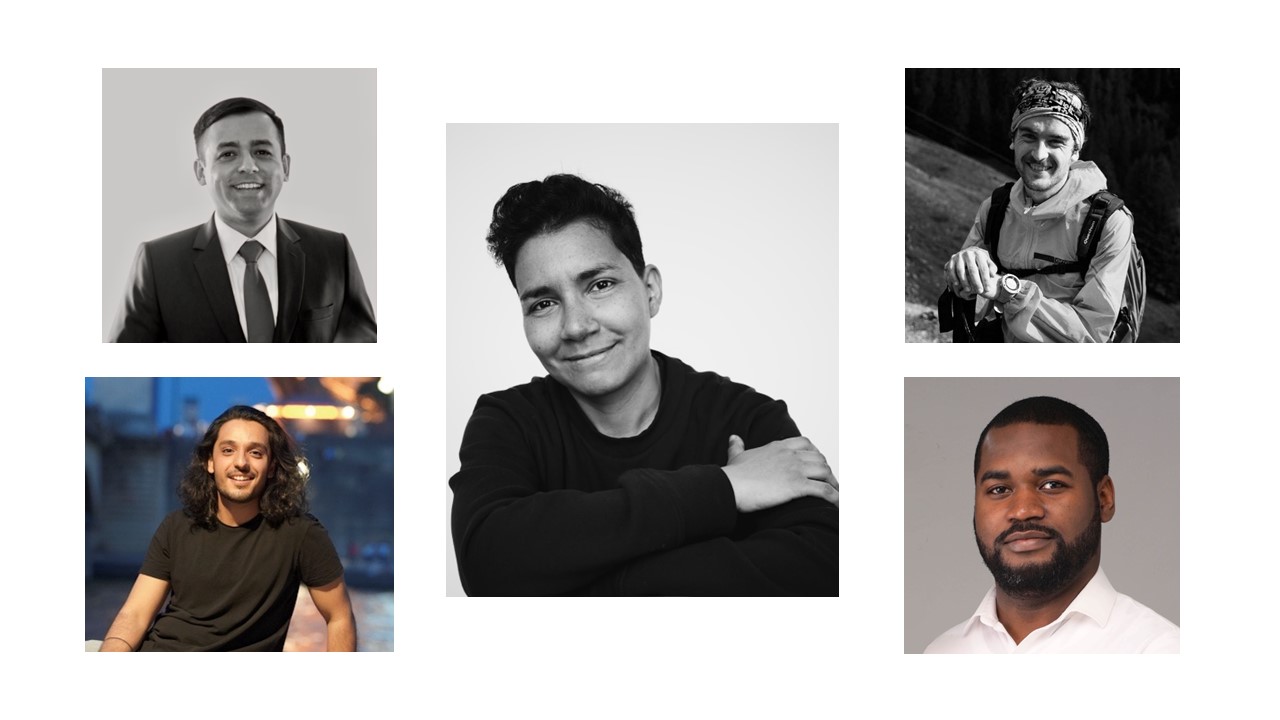