Towards greener last-mile operations: Supporting cargo-bike logistics through optimized routing of multi-modal urban delivery fleets
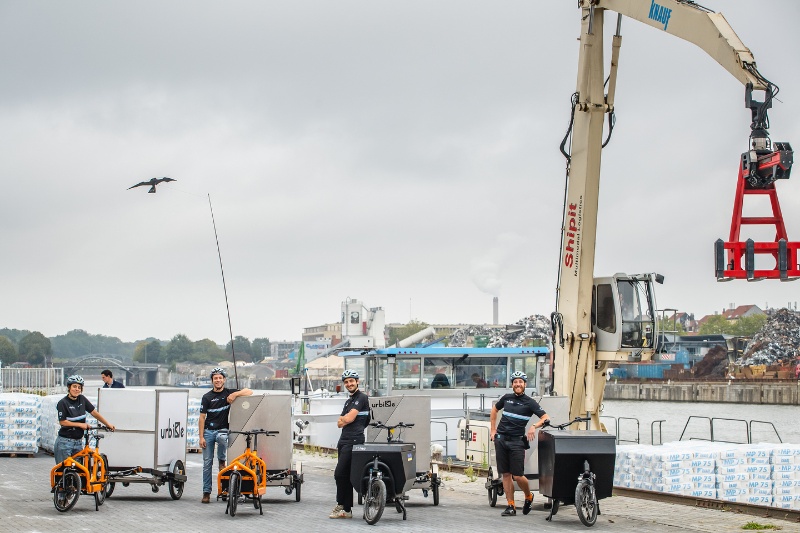
PI and co-PIs: Maria Astefanoaei (IT University of Copenhagen); Akash Srivastava (MIT-IBM Watson AI Lab, IBM Research); Kai Xu (University of Edinburgh); Nicolas Collignon (Kale Collective); Esben Sørig (Kale Collective); Soonmyeong Yoon (Kale Collective)
Funding amount: $145,000
Project overview: As greenhouse gas emissions associated with the transportation of goods remain high, cargo bikes offer promise as a partial alternative to vans and other carriers in the urban setting. This project aims to solve the data-related challenges that are currently hindering the wider adoption of the cargo bike in logistics operations by developing a data-driven simulation tool that would allow for high-fidelity simulation of hybrid (van + cargo-bike) fleet operation under real-world settings. This work, which can be applied to any urban region with OpenStreetMap data, could influence both urban logistics operators and city decision makers, by enabling more effective planning. Wider adoption of cargo bikes in logistics operations could significantly reduce emissions (in comparison to both diesel vans and electric vans), as well as alleviating traffic congestion, noise pollution, and van-related accidents.
Full abstract:
Click to expand
Light goods vehicles (LGV) used extensively in the last mile of delivery are one of the leading polluters in cities. Recently, cargo bike logistics has been put forward as a competitive, zero-emission candidate for replacing LGVs in urban areas, with experts estimating 51% of van deliveries being replaceable by cargo bikes. Due to their faster speeds, shorter parking times and more efficient routes across cities, they can out-compete traditional van logistics when operated effectively. Despite this competitive advantages in dense urban areas, their widespread adoption by logistics operator is limited. This is primarily due to the lack of inexpensive ways of accurately evaluating their impact on the cost of business. To this end, we aim to develop data-driven tools to allow for high-fidelity simulation of hybrid (vans+cargo-bikes) fleet-operation under real-world settings. Our simulator will enable key stakeholders to run feasibility studies towards optimizing and diversifying their fleet composition in a cost-effective manner. Finally, the proposed simulator will also allow for applying new Machine Learning methods such as reinforcement learning and hybrid approaches such as Bayesian-symbolic generative models to the problem of routing mixed fleets for last mile deliveries, taking into account the cargo-bike specific problems of operators.
Transportation Cities & Urban Planning Sustainability