Detecting Flooding in Fiji's Croplands
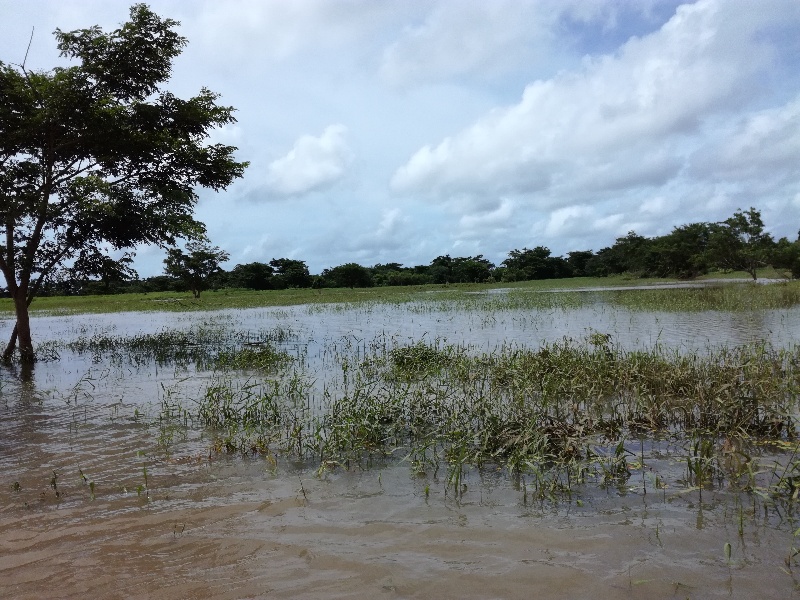
PI and co-PIs: John Duncan (University of Western Australia); Bryan Boruff (University of Western Australia); Nathan Wales (University of Western Australia); Solomoni Nagaunavou (School of Geography, Earth Science, and Environment, The University of the South Pacific); Renata Varea (Ministry of Agriculture, Government of Fiji); Kevin Davies (School of Geosciences, The University of Sydney); Eleanor Bruce (School of Geosciences, The University of Sydney)
Funding amount: $145,000
Project overview: Climate change is influencing the occurrence of tropical cyclones, threatening food production as well as the safety of local communities. This project is developing remote sensing tools allowing the government of Fiji to rapidly map flooding in croplands, supporting national adaptation efforts and frontline disaster response strategies in the agricultural sector. By facilitating quicker and more targeted responses to crop damage, the project has the potential to mitigate economic losses and preserve the livelihoods of vulnerable small-scale farmers across Fiji, as well as pointing the way to similar maps across the region.
Full abstract:
Click to expand
This project is a collaboration between researchers at Australian and Fijian universities and the Fijian Government’s Ministry of Agriculture. It will develop machine learning methods to map flooding in Fiji’s croplands following tropical cyclone / storm events. In recent years, tropical cyclone events have been causing crop damages in excess of $100 million impacting subsistence livelihoods and commercial farms. A major task of agricultural officials is undertaking post-event damage assessments to allocate relief and to support farmers with recovering their operations. Given the wide swaths of areas impacted by tropical cyclones and the remote locations of many farms, damage assessment can be a time-consuming task. Further, many small-scale farmers are missed in programs targeting response, recovery, and pre-event phases of the disaster management cycle. These are often farmers with the fewest resources to adapt and respond to climatic challenges. To support the Ministry of Agriculture’s flood response and planning activities, this project will develop a workflow that combines machine learning and geostatistical algorithms to generate post-cyclone / storm cropland flood maps from satellite images. This workflow will update cropland flood maps as new satellite images are captured and will guide agricultural official’s damage assessments. This workflow will be sensitive to flooding in small-scale farming systems to ensure these impacts are accounted for in post-event damage assessments. These algorithms will also be applied to an archive of satellite images to generate a recent history of cropland flood extents. The application of these methods will contribute to Fiji’s adaptation efforts and disaster response in the agricultural sector aligning with Adaptation Target 7 of Fiji’s 2021 update to its Nationally Determined Contribution to the Paris Agreement to develop monitoring systems to mitigate the impact of flooding and cyclones.
Disaster Management and Relief Agriculture & Food Earth Observation & Monitoring Extreme Weather Computer Vision & Remote Sensing