Learning Power System Dynamics in the Frequency Domain
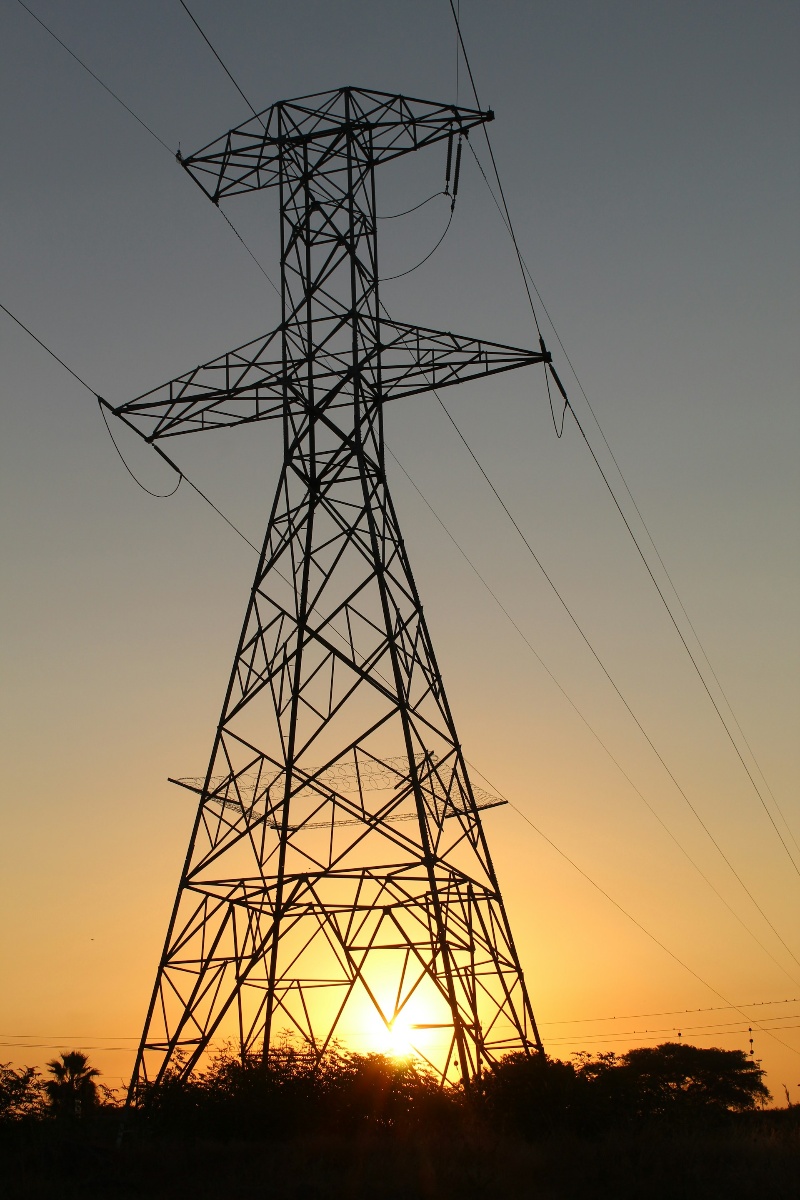
PI and co-PIs: Baosen Zhang (University of Washington); Weiwei Yang (Microsoft Research); Yixing Xu (Breakthrough Energy)
Funding amount: $145,000
Project overview: The energy transition will require significant improvements to electrical grid efficiency and robustness to disruption. Solving such problems entails effective dynamic simulation of the grid, which has been very computationally challenging to date. This project proposes a new machine learning framework which uses computations in the Fourier space to model power system dynamics, significantly improving prediction speed and accuracy. The team aims to use the methodology in particular to address the critical problem of security-constrained optimal power flow. Overall, improved ML predictions of power systems should make it possible to advance climate change mitigation goals by better integrating renewable resources into the grid and improving grid stability.
Full abstract:
Click to expand
With the goal of reducing carbon emissions and mitigating climate change, many regions have set ambitious targets to generate electricity with close to 100\% renewables. However, actual renewable generations are often curtailed due to their uncertainty, especially because the difficulty in checking their impact on the dynamic stability of the grid. The dynamic behavior of a power grid is governed by a large number of nonlinear ordinary differential equations. To safely operate the system, operators need to check that the states described by this set of equations stay within prescribed limits after various faults. But existing numerical solvers are slow and machine learning approaches have been proposed to improve computational efficiency. However, current learning methods are difficult to scale, suffer from overfitting and fails to capture unstable behaviors. This project, we propose a framework for power system dynamic simulation using discrete Fourier transform to learn in the frequency domain. We show that although the time domain trajectories are complicated, they are sparse in the frequency domain. The system topology and fault information are encoded through a 3D Fourier transform, and we learn the right filtering and nonlinear transformations in this domain. Our preliminary results show that our approach speeds up computation by orders of magnitude while remaining highly accurate. We work to provide both sound theoretical understanding of the proposed method and integrating it into existing tools. The results would be implemented by the team and released as open source tools to the wider community.
Power & Energy