Extracting and Discovering New Measurements from Climate Text Sources
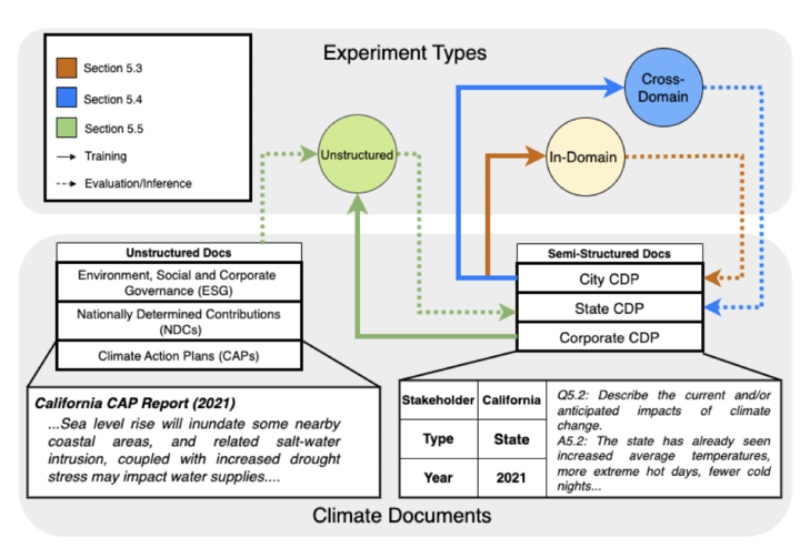
PI and co-PIs: Taylor Berg-Kirkpatrick (University of California San Diego); Tom Corringham (Scripps Institution of Oceanography)
Funding amount: $145,000
Project overview: Much of the world’s climate-relevant data is not readily available for use by researchers and policymakers. Specifically, numerous sources, such as agency reports and corporate filings, include important figures buried in text that is not machine-readable. This project aims to create a natural language processing framework to pick out numerical information from climate text sources, allowing users to quickly extract numbers and units in response to a query. The team will release both the trained AI tool and a new dataset of closely annotated text to guide future research. Stakeholders across many areas of climate science and adaptation have expressed interest in using the proposed toolkit.
Full abstract:
Click to expand
Achieving a just and efficient transition from fossil fuels to renewable energy and implementing just and efficient climate adaptation strategies are among the most significant global challenges of the 21st century. Meeting these challenges requires reliable data. There is a key role for machine learning (ML) and natural language processing (NLP) techniques to extract data from a growing body of text-based climate documents. We propose to develop a novel NLP framework that supports fine-grained extraction of numerical quantities in climate text sources, along with their corresponding unit types; and further, that supports user-guided filtration of extracted measurements according to their semantic types -- e.g. 'rainfall' or 'flood area'. Our system will enable climate scientists to efficiently sift through vast quantities of raw text in order to create new and more useful datasets, as well as extract and aggregate relevant statistics. Further, in order to train and validate our proposed system, we aim to collect a large-scale dataset of climate text sources with manual fine-grained annotations which may be of use to the broader community.
Impact Assessment Societal Adaptation & Resilience Supply Chains Transportation Natural Language Processing