ICLR 2024 Workshop: Tackling Climate Change with Machine Learning
About
Many in the ML community wish to take action on climate change, but are unsure how to have the most impact. This workshop will highlight work that demonstrates that, while ML is no silver bullet, it can be an invaluable tool in reducing greenhouse gas emissions and in helping society adapt to the effects of climate change.
Climate change is a complex problem for which action takes many forms, from advancing theory to deploying new technology. Many of these actions represent high-impact opportunities for real-world change, and simultaneously pose interesting academic research problems.
This workshop is part of a series that aims to bring together those applying ML to climate change challenges and facilitate cross-pollination between ML researchers and experts in climate-relevant fields.
Building on our past workshops on this topic, this edition focuses on two aspects related to fostering the maturity of ML applications for tackling climate change. First, the workshop will shed light on work that employs, analyzes, or critiques ML methods and their use for climate change mitigation and adaptation, including both research and deployment. In addition, we will discuss the key investment mechanics and policy frameworks needed to advance these applications toward just and equitable large-scale deployment and achieve positive societal-scale impact.
About ICLR
This workshop is part of the International Conference on Learning Representations (ICLR), one of the premier conferences on machine learning. For information on how to attend the ICLR conference, please see here https://iclr.cc/Conferences/2024.
About the Workshop
The main workshop took place on May 11, 2024. The recording and the schedule are available below, with links to papers, videos, and slides.
Schedule Full Recording
Time (Workshop) | Event |
---|---|
Opening Remarks | |
Spotlight presentations | |
(63) "Building Ocean Climate Emulators" | |
(19) "Advancing Earth System Model Calibration: A Diffusion-Based Method" | |
(11) "Extreme Precipitation Nowcasting using Transformer-based generative models" | |
(79) "One Prompt Fits All: Visual Prompt-Tuning for Remote Sensing Segmentation" | |
Poster Session 1 | |
Coffee Break | |
Keynote 1 | |
Panel 1: Pathways to Industry Deployment: Enabling and Sustaining Maturity of ML Applications for Climate
Details: (click to expand)Panelists:
|
|
Lunch | |
Keynote 2 | |
Spotlight presentations | |
(21) "Reconstructing the Breathless Ocean with Spatio-Temporal Graph Learning" | |
(62) "Global Vegetation Modeling With Pre-Trained Weather Transformers" | |
(77) "Planning for Floods & Droughts: Intro to AI-Driven Hydrological Modeling" | |
(16) "Time-Varying Constraint-Aware Reinforcement Learning for Energy Storage Control" | |
(7) "Scaling Transformers for Skillful and Reliable Medium-range Weather Forecasting" | |
Poster Session 2 | |
Networking session (sponsor: Celonis) | |
Panel 2: Shaping the ML Innovation Landscape with a Climate Lens
Details: (click to expand)Panelists:
|
|
Closing Remarks & Announcement of Best Paper Awards |
Speakers
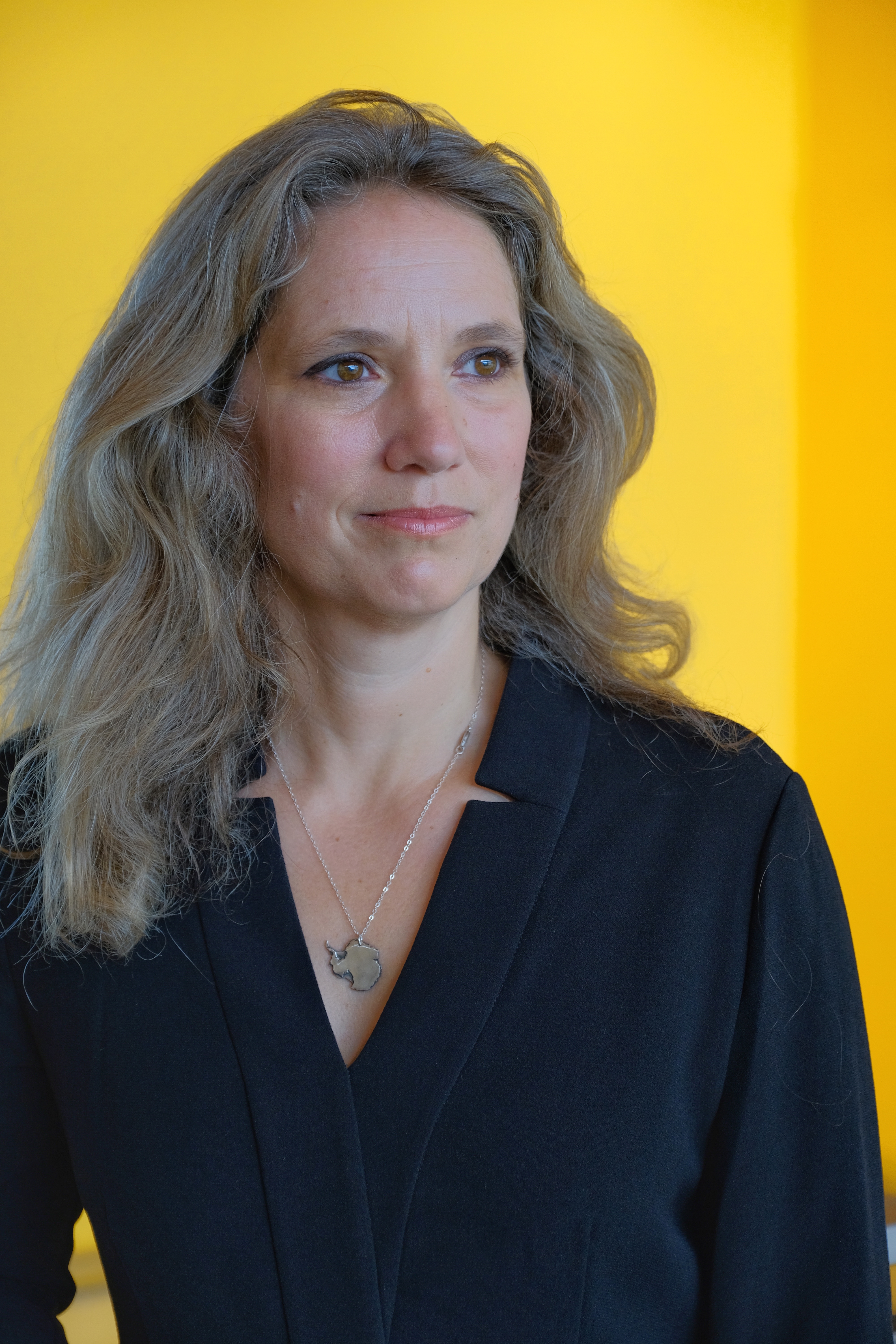
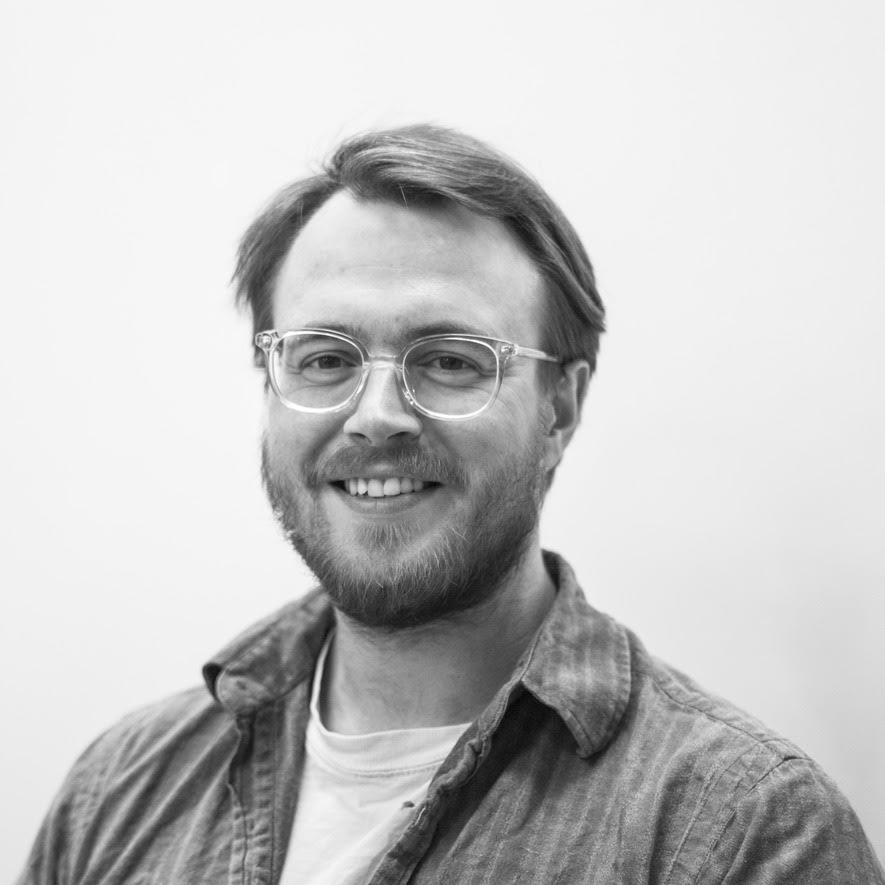
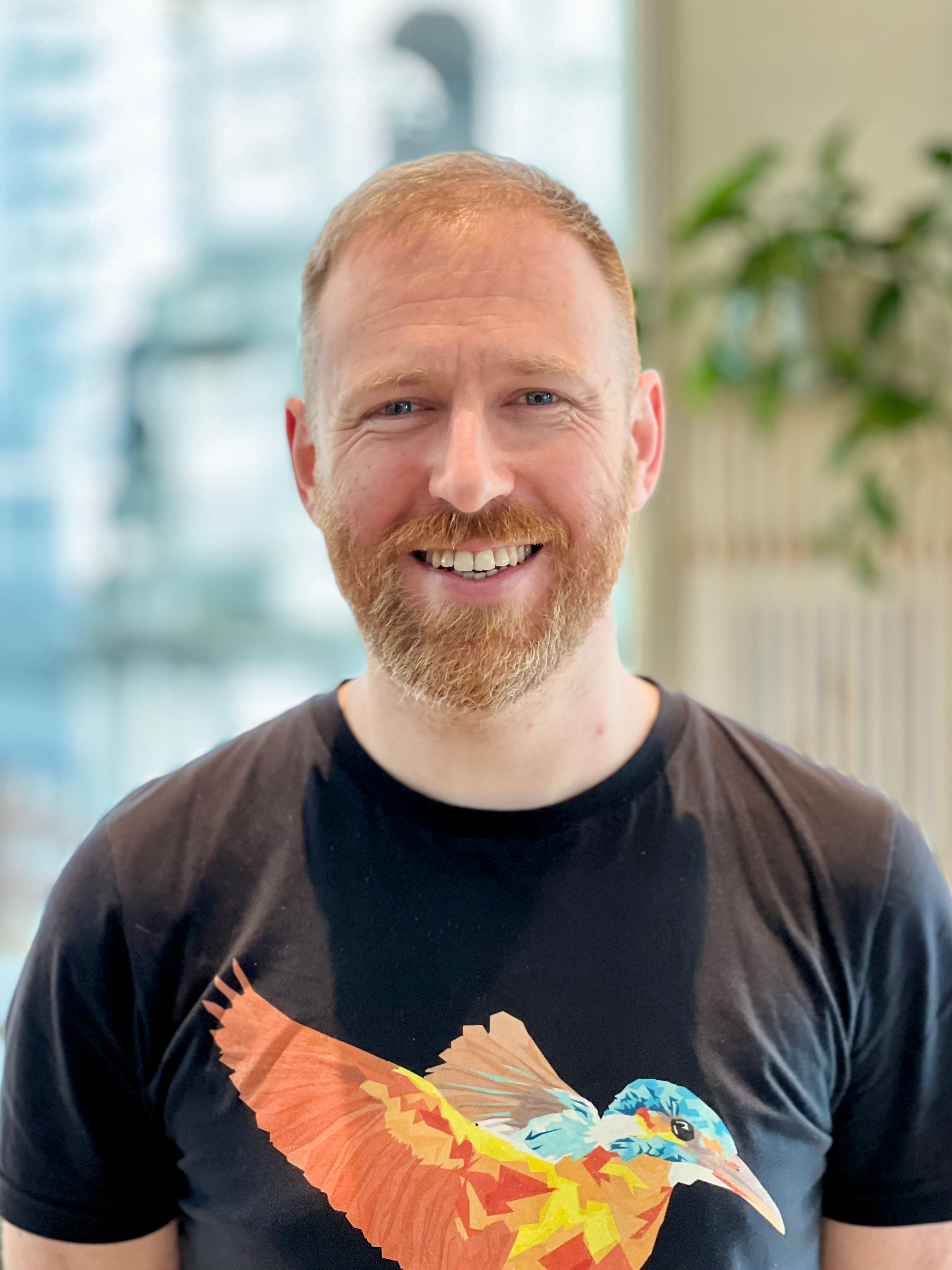
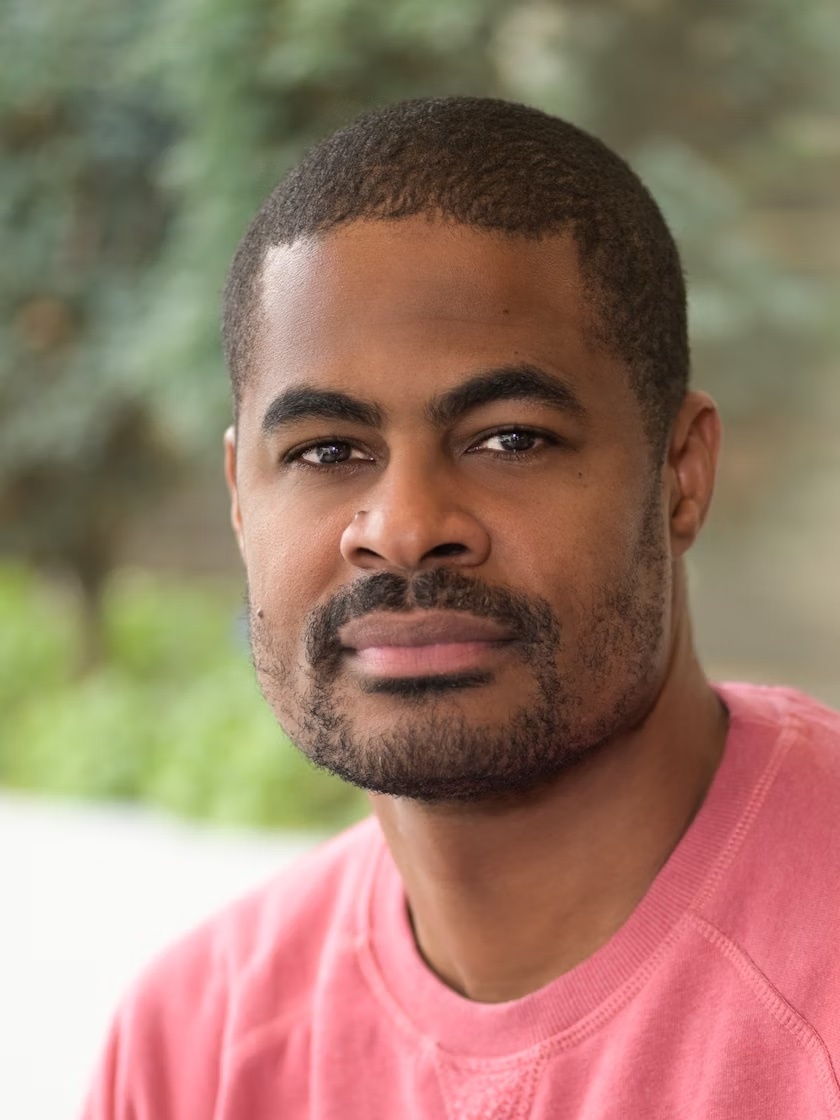
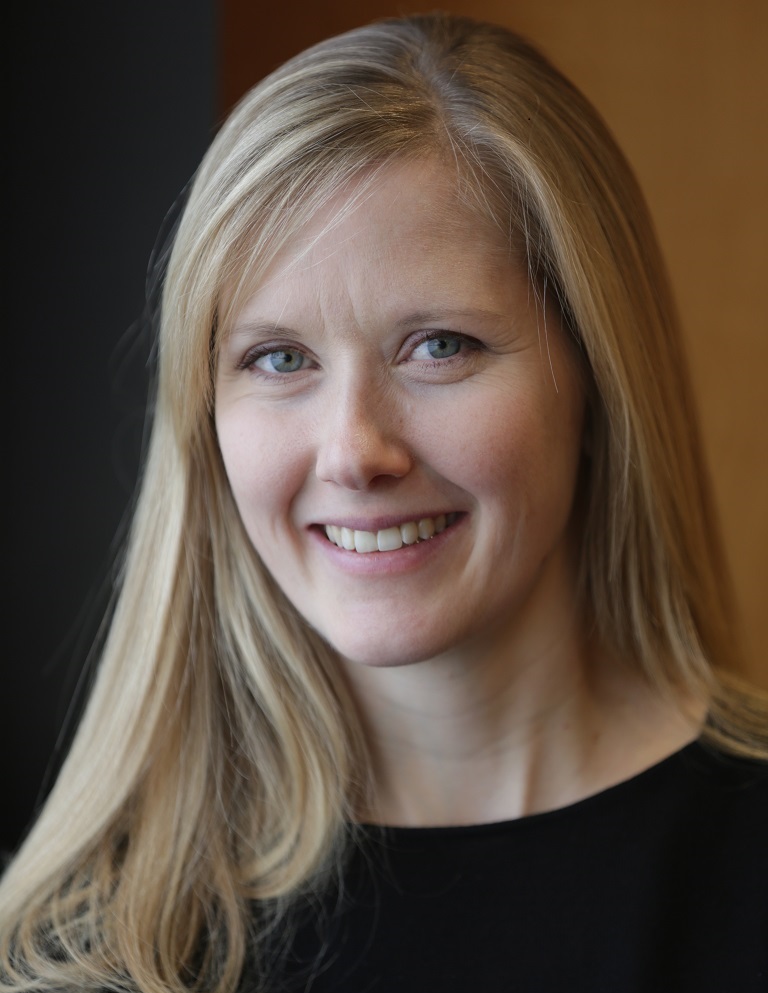
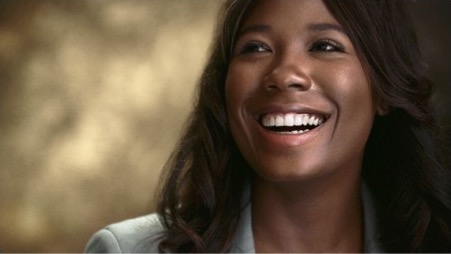
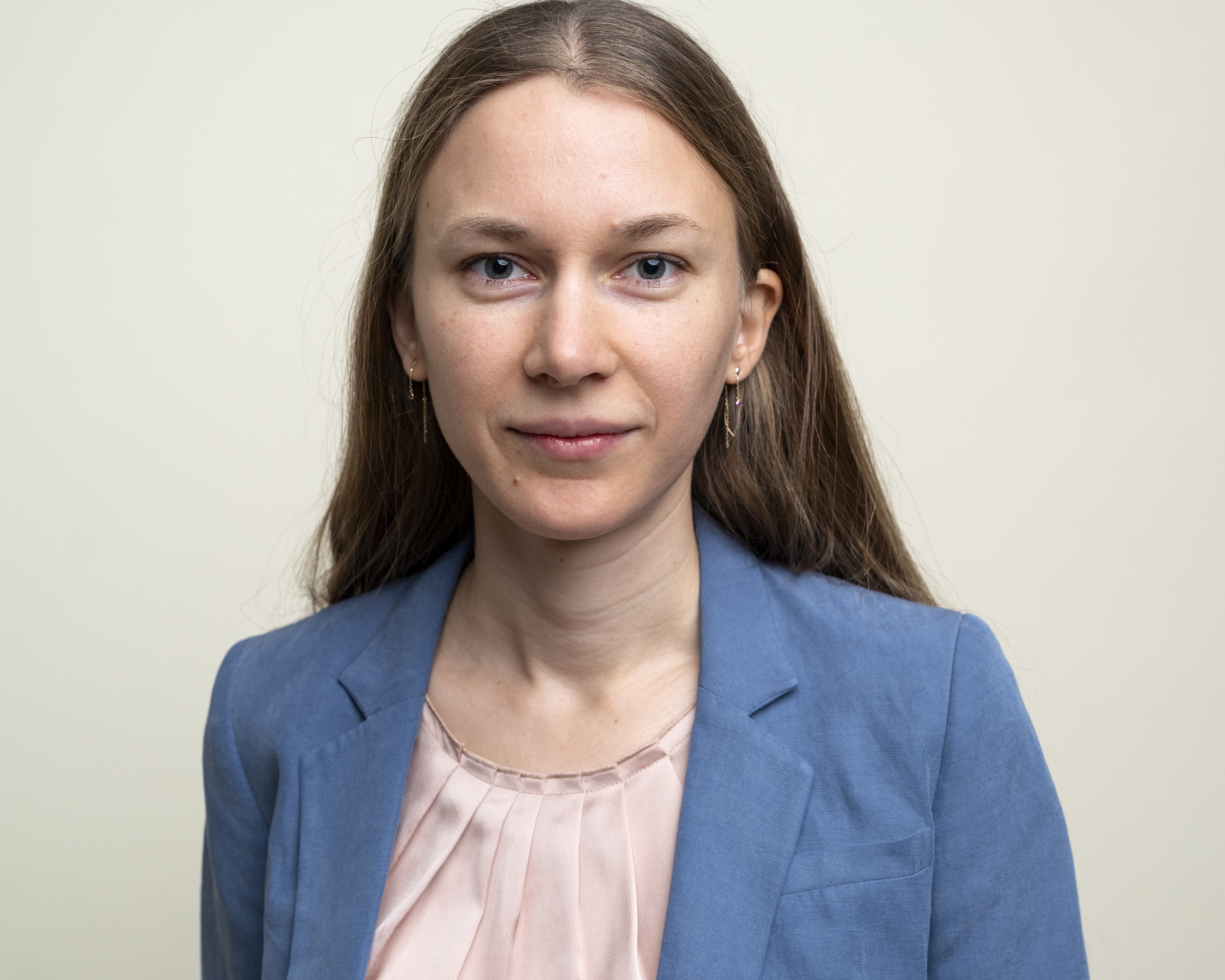
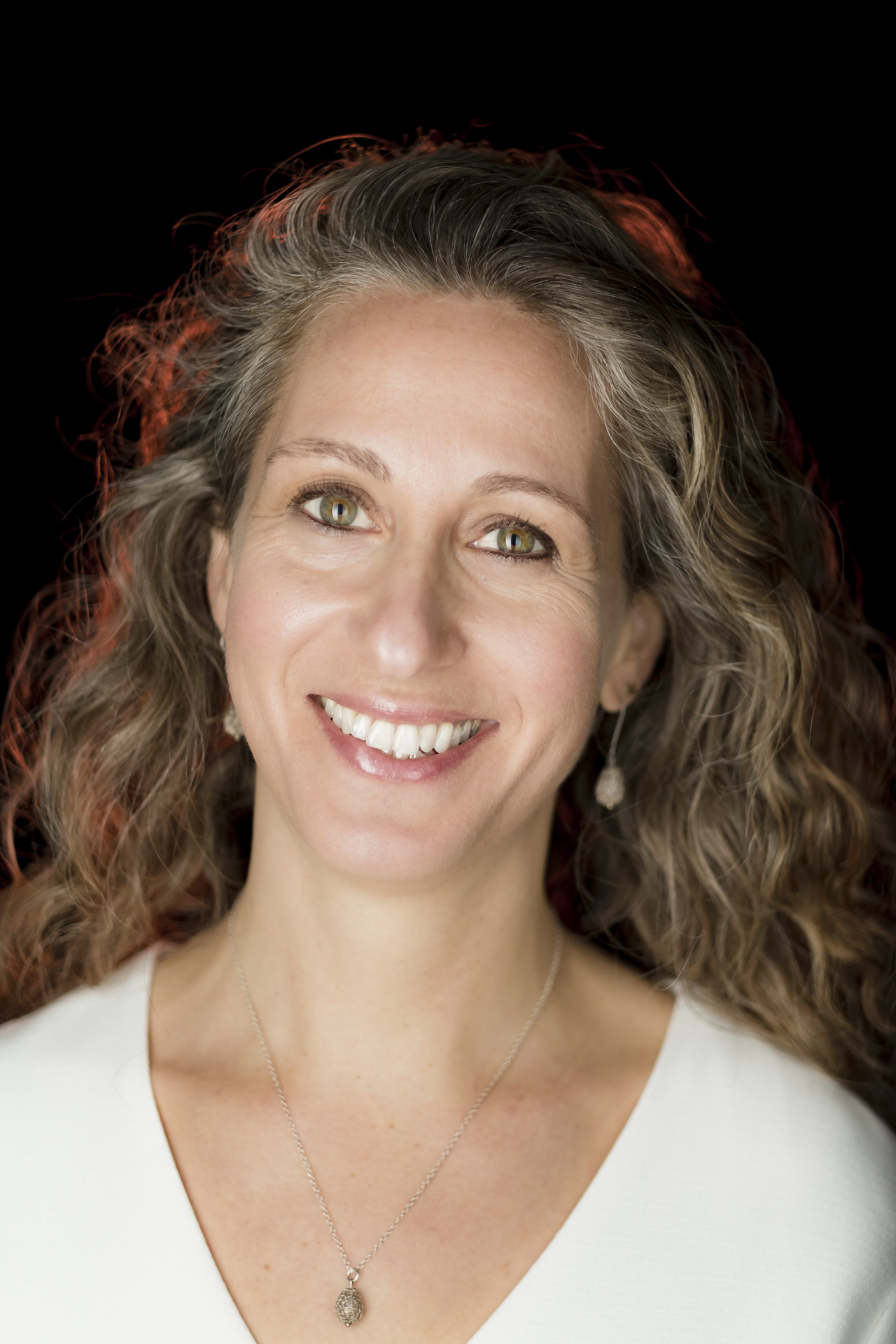
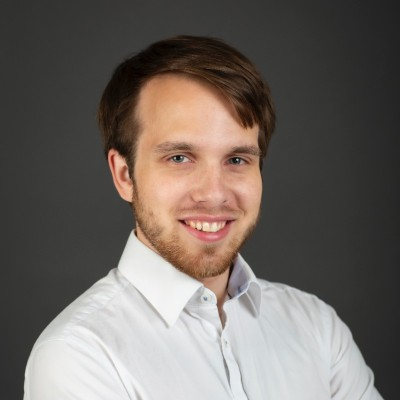
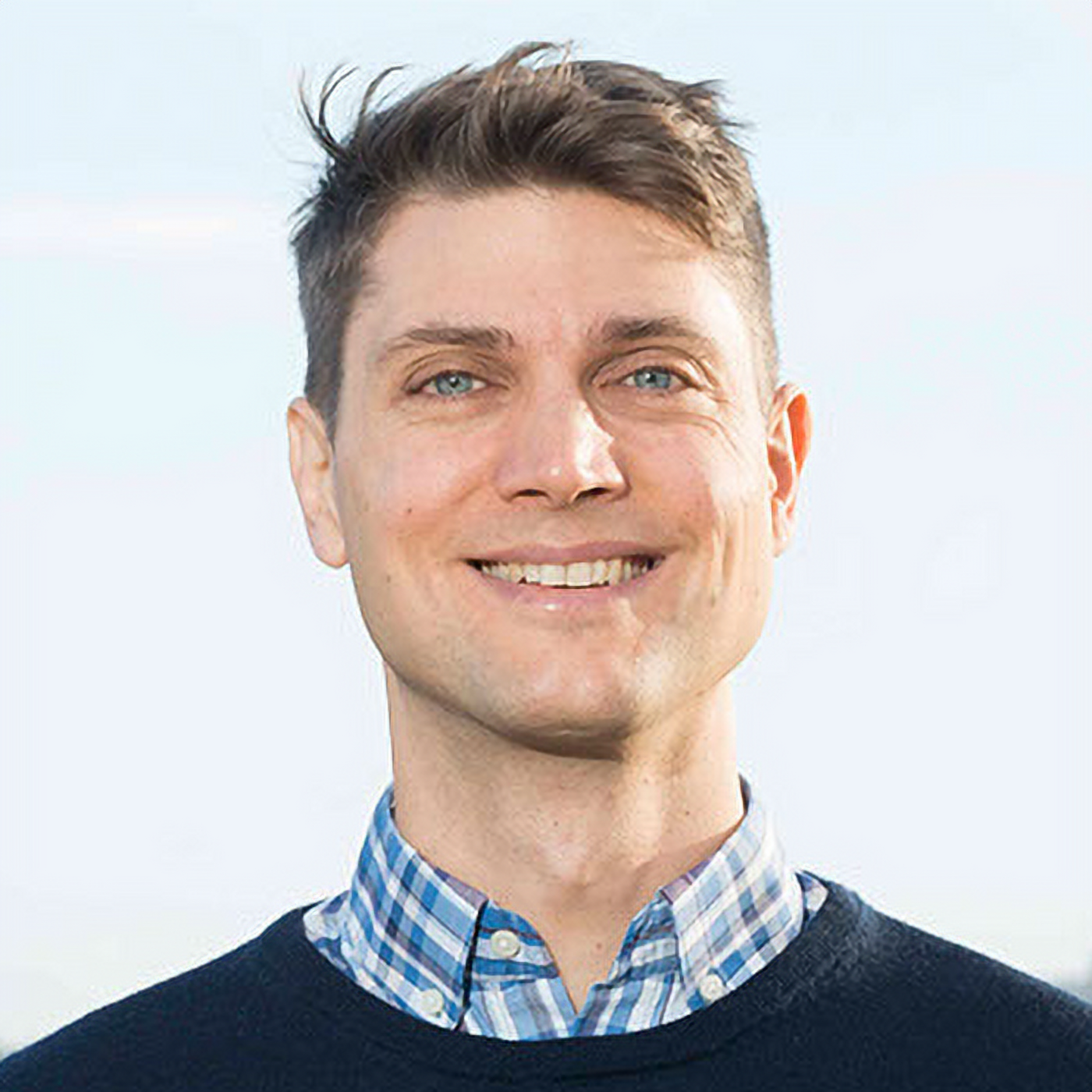
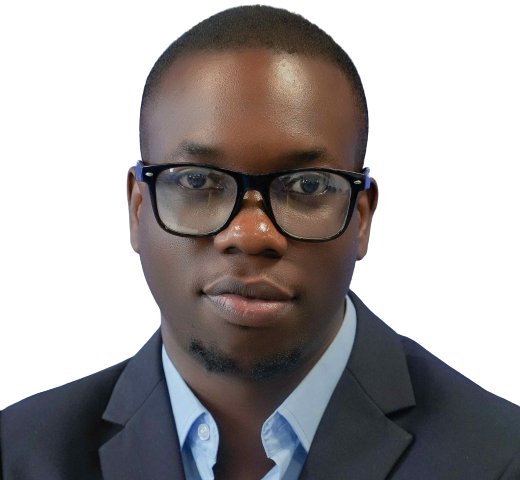
Accepted Works
Works were submitted to one of three tracks: Papers, Proposals, or Tutorials.
Click the links below for information about each submission, including slides, videos, and papers.
Papers
Title | Authors |
---|---|
(1) Mapping Land Naturalness from Sentinel-2 using Deep Contextual and Geographical Priors | Burak Ekim (University of the Bundeswehr); Michael Schmitt (University of the Bundeswehr Munich) |
(2) Structured spectral reconstruction for scalable soil organic carbon inference | Evan A Coleman (MIT); Sujay Nair (Georgia Institute of Technology); Xinyi Zeng (Coho Climate Advisors); Elsa Olivetti (MIT Department of Materials Science & Engineering) |
(3) ClimateQ&A : bridging the gap between climate scientists and the general public | Natalia de la Calzada (Ekimetrics); Theo Alves Da Costa (Ekimetrics); Annabelle Blangero (Ekimetrics); Nicolas CHESNEAU (EKIMETRICS) |
(4) Improving Streamflow Predictions with Vision Transformers | Kshitij Tayal (Oak Ridge National Lab); Arvind Renganathan (University of Minnesota); Dan Lu (Oak Ridge National Laboratory) |
(5) Grapevine Disease Prediction Using Climate Variables from Multi-Sensor Remote Sensing Imagery via a Transformer Model | Weiying Zhao (Deep Planet); Natalia Efremova (Queen Mary University London) |
(6) Towards Ecological Network Analysis with Gromov-Wasserstein Distances | Kai M Hung (Rice University); Ann Finneran (Rice University); Alex Zalles (Rice University); Lydia Beaudrot (Rice University); Cesar Uribe (Rice University) |
(7) Scaling Transformers for Skillful and Reliable Medium-range Weather Forecasting Overall Best Paper | Tung Nguyen (University of California, Los Angeles); Rohan Shah (Carnegie Mellon University); Hritik Bansal (UCLA); Troy Arcomano (Argonne National Laboratory); Sandeep Madireddy (Argonne National Laboratory); Romit Maulik (Argonne National Laboratory); Veerabhadra Kotamarthi (Argonne National Laboratory); Ian Foster (Computation Institute); Aditya Grover (UCLA) |
(8) Categorization of Meteorological Data by Contrastive Clustering | Michael Dammann (HAW Hamburg); Ina Mattis (DWD); Michael Neitzke (HAW Hamburg); Ralf Möller (University of Lübeck) |
(9) AI-driven emulation of ocean dynamics on sub-seasonal scales | Suyash Bire (Massachusetts Institute of Technology); Jean Kossaifi (NVIDIA); Simone Silvestri (Massachusetts Institute of Technology); Nikola Kovachki (Nvidia Corp.); Kamyar Azizzadenesheli (Nvidia Corp.); Chris N Hill (MIT); Animashree Anandkumar (Caltech) |
(10) Calibrating Bayesian UNet++ for Sub-seasonal Forecasting | Büşra Asan (Istanbul Technical University); Abdullah Akgül (University of Southern Denmark); Alper Unal (Istanbul Technical University); Melih Kandemir (University of Southern Denmark); Gozde Unal (Istanbul Technical University) |
(11) Extreme Precipitation Nowcasting using Transformer-based generative models | Cristian Meo (TUDelft); Mircea T Lica (Delft University of Technology); Ankush Roy (TUDelft); Zeina Boucher (TUDelft); Junzhe Yin (TUDelft); Yanbo Wang (Delft University of Technology); Ruben Imhoff (Deltares); Remko Uijlenhoet (TUDelft); Justin Dauwels (TU Delft) |
(12) GeoFormer: A Vision and Sequence Transformer-based Approach for Greenhouse Gas Monitoring | Madhav Khirwar (Independent) |
(13) Paddy Doctor: Open Dataset and Automated Pest Identification Using Pre-trained Deep Learning Models | Petchiammal A (Manonmaniam Sundaranar University, Tirunelveli, Tamil Nadu); Pandarasamy Arjunan (Indian Institute of Science, Bangalore) |
(14) Using expired weather forecasts to supply 10 000y of data for accurate planning of a renewable European energy system | Petr Dolezal (AI4ER CDT, University of Cambridge); Emily Shuckburgh (University of Cambridge) |
(15) Black carbon plumes from gas flaring in North Africa identified from multi-spectral imagery with deep learning | Alexandre Tuel (Galeio); Thomas Kerdreux (INRIA/ ENS); Louis THIRY (ENS Paris) |
(16) Time-Varying Constraint-Aware Reinforcement Learning for Energy Storage Control | Jaeik Jeong (Electronics and Telecommunications Research Institute (ETRI)); Tai-Yeon Ku (Electronics and Telecommunications Research Institute (ETRI)); Wan-Ki Park (Electronics and Telecommunications Research Institute (ETRI)) |
(17) Towards a Data-Driven Understanding of Cloud Structure Formation | Ann-Christin Wörl (Johannes Gutenberg University); Michael Wand (University of Mainz); Peter Spichtinger (Johannes Gutenberg University) |
(18) Bee Activity Prediction and Pattern Recognition in Environmental Data | Christine Preisach (University of Applied Sciences Karlsruhe); Marius Herrmann (Karlsruhe Institute of Technology) |
(19) Advancing Earth System Model Calibration: A Diffusion-Based Method Honorable Mention | Yanfang Liu (Oak Ridge National Laboratory); Dan Lu (Oak Ridge National Laboratory); Zezhong Zhang (Oak Ridge National Laboratory); Feng Bao (Florida State University); Guannan Zhang (Oak Ridge National Laboratory) |
(20) Towards Downscaling Global AOD with Machine Learning | Josh Millar (Imperial College London); Paula Harder (Mila); Lilli J Freischem (University of Oxford); Philipp Weiss (University of Oxford); Philip Stier (University of Oxford) |
(21) Reconstructing the Breathless Ocean with Spatio-Temporal Graph Learning | Bin Lu (Shanghai Jiao Tong University); Ze Zhao (Shanghai Jiao Tong University); Luyu Han (Shanghai Jiao Tong University); Xiaoying Gan (Shanghai Jiao Tong University); Yuntao Zhou (Shanghai Jiao Tong University); Lei Zhou (Shanghai Jiao Tong Univ); Luoyi Fu (Shanghai Jiao Tong University); Xinbing Wang (Shanghai Jiao Tong University); Chenghu Zhou (Institute of Geographic Sciences and Natural Resources Research, CAS); Jing Zhang (Shanghai Jiao Tong University) |
(22) Analyzing the secondary wastewater-treatment process using Faster R-CNN and YOLOv5 object detection algorithms | Offir Inbar (Tel-Aviv University); Moni Shahar (Tel Aviv University); Jacob Gidron (Tel-Aviv University); Ido Cohen (Tel-Aviv University); Dror Avisar (Tel-Aviv University) |
(23) Diffusion-Based Joint Temperature and Precipitation Emulation of Earth System Models | Katie Christensen (Western Washington University); Lyric Otto (Western Washington University); Seth Bassetti (Utah State University); Claudia Tebaldi (Joint Global Change Research Institute); Brian Hutchinson (Western Washington University) |
(24) Verifying Practices of Regenerative Agriculture: African Smallholder Farmer Dataset for Remote Sensing and Machine Learning | Yohei Nakayama (Degas Ltd); Grace Antwi (Degas Ltd); Seiko Shirasaka (Keio University) |
(25) Semi-Supervised Domain Adaptation for Wildfire Detection | Joo Young Jang (Alchera); Youngseo Cha (Alchera); Jisu Kim (Alchera); SooHyung Lee (Alchera); Geonu Lee (Alchera); Minkook Cho (Alchera); Young Hwang (Alchera); Nojun Kwak (Seoul National University) |
(26) Identifying Climate Targets in National Laws and Policies using Machine Learning | Matyas Juhasz (Climate Policy Radar); Tina Marchand (Climate Policy Radar); Roshan Melwani (Climate Policy Radar); Kalyan Dutia (Climate Policy Radar); Sarah Goodenough (Climate Policy Radar); Harrison Pim (Climate Policy Radar); Henry Franks (Climate Policy Radar) |
(27) Empowering Sustainable Finance: Leveraging Large Language Models for Climate-Aware Investments | Ayush Jain (IBM Research); Manikandan Padmanaban (IBM Research India); Jagabondhu Hazra (IBM Research India); Shantanu Godbole (IBM India); Hendrik Hamann (IBM Research) |
(28) Near-real-time monitoring of global ocean carbon sink | Xiaofan Gui (Microsoft Research); Jiang Bian (Microsoft Research) |
(29) WindDragon: enhancing wind power forecasting with automated deep learning | Julie Keisler (INRIA, EDF R&D); Etienne Le Naour (Sorbonne University, EDF R&D) |
(30) Neural Processes for Short-Term Forecasting of Weather Attributes | Benedetta L Mussati (University of Oxford); Helen McKay (Mind Foundry); Stephen Roberts (University of Oxford) |
(31) EU Climate Change News Index: Forecasting EU ETS prices with online news | Aron Pap (BGSE); Aron D Hartvig (Corvinus University of Budapest, Cambridge Econometrics); Péter Pálos (Budapest University of Technology and Economics) |
(32) Explaining Zeolite Synthesis-Structure Relationships using Aggregated SHAP Analysis | Elton Pan (MIT) |
(33) Fast non-stationary geospatial modelling with multiresolution (wavelet) Gaussian processes | Talay M Cheema (University of Cambridge); Carl Edward Rasmussen (Cambridge University) |
(34) PostRainBench: A comprehensive benchmark and a new model for precipitation forecasting | Yujin Tang (The Hong Kong University of Science and Technology (Guangzhou)); Jiaming Zhou (Hong Kong University of Science and Technology (Guangzhou)); Xiang Pan (Nanjing University); Zeying Gong (Hong Kong University of Science and Technology (Guangzhou)); Junwei Liang (The Hong Kong University of Science and Technology (Guangzhou)) |
(35) Physics-informed Machine Learning-based Cloud Microphysics parameterization for Earth System Models | Ellen Sarauer (German Aerospace Center (DLR)); Mierk Schwabe (Deutsches Zentrum für Luft- und Raumfahrt e.V. (DLR), Institut für Physik der Atmosphäre, Oberpfaffenhofen, Germany); Philipp Weiss (University of Oxford); Axel Lauer (Deutsches Zentrum für Luft- und Raumfahrt e.V. (DLR), Institut für Physik der Atmosphäre, Oberpfaffenhofen, Germany); Philip Stier (University of Oxford); Veronika Eyring (Deutsches Zentrum für Luft- und Raumfahrt e.V. (DLR), Institut für Physik der Atmosphäre, Oberpfaffenhofen, Germany; University of Bremen, Institute of Environmental Physics (IUP), Bremen, Germany) |
(36) From spectra to biophysical insights: end-to-end learning with a biased radiative transfer model | Yihang She (University of Cambridge); Clement Atzberger (Mantle Labs); Andrew Blake (University of Cambridge, Mantle Labs); Srinivasan Keshav (University of Cambridge) |
(37) Model Failure or Data Corruption? Exploring Inconsistencies in Building Energy Ratings with Self-Supervised Contrastive Learning | Qian Xiao (Trinity College Dublin); Dan Liu (Trinity College Dublin); Kevin Credit (Maynooth University) |
(38) Interpretable Machine Learning for Extreme Events detection: An application to droughts in the Po River Basin | Paolo Bonetti (Politecnico di Milano); Matteo Giuliani (Politecnico di Milano); Veronica Cardigliano (Politecnico di Milano); Alberto Maria Metelli (Politecnico di Milano); Marcello Restelli (Politecnico di Milano); Andrea Castelletti (Politecnico di Milano) |
(39) Literature Mining with Large Language Models to Assist the Development of Sustainable Building Materials | Yifei Duan (Massachusetts Institute of Technology); Yixi Tian (Massachusetts Institute of Technology); Soumya Ghosh (IBM Research); Richard Goodwin (IBM T.J. Watson Research Center); Vineeth Venugopal (Massachusetts Institute of Technology); Jeremy Gregory (Massachusetts Institute of Technology); Jie Chen (IBM Research); Elsa Olivetti (Massachusetts Institute of Technology) |
(40) A Deep Learning Technology Suite for Cost-Effective Sequestered CO2 Monitoring | Wenyi Hu (SLB); Son Phan (SLB); Cen Li (SLB); Aria Abubakar (SLB) |
(41) On the potential of Optimal Transport in Geospatial Data Science | Nina V Wiedemann (ETH Zurich); Martin Raubal (ETH Zürich) |
(42) ExioML: Eco-economic dataset for Machine Learning in Global Sectoral Sustainability | Yanming Guo (University of Sydney) |
(43) FARADAY: SYNTHETIC SMART METER GENERATOR FOR THE SMART GRID | Sheng Chai (Centre for Net Zero); Gus Chadney (Centre for Net Zero) |
(44) Generalizable Temperature Nowcasting with Physics-Constrained RNNs for Predictive Maintenance of Wind Turbine Components | Johannes Exenberger (Graz University of Technology); Matteo Di Salvo (Sirius Energy Automation); Thomas Hirsch (Graz University of Technology); Franz Wotawa (Graz University of Technology, Institute for Software Technology); Gerald Schweiger (Graz University of Technology) |
(45) Forecasting Tropical Cyclones with Cascaded Diffusion Models | Pritthijit Nath (Imperial College London); Pancham Shukla (Imperial College London); Shuai Wang (University of Delaware); Cesar Quilodran-Casas (Imperial College London) |
(46) Forecasting regional PV power in Great Britain with a multi-modal late fusion network | James Fulton (Open Climate Fix); Jacob Bieker (Open Climate Fix); Peter Dudfield (Open Climate Fix); Solomon Cotton (Open Climate Fix); Zakari Watts (Open Climate Fix); Jack Kelly (Open Climate Fix) |
(47) Exploring Graph Neural Networks to Predict the Seagrasses Ecosystem State in the Italian Seas | Angelica Bianconi (University School for Advanced Studies (IUSS) Pavia & Ca’ Foscari University of Venice); Sebastiano Vascon (Ca' Foscari University of Venice & European Centre for Living Technology); Elisa Furlan (Centro Euro-Mediterraneo sui Cambiamenti Climatici (CMCC) & Ca' Foscari University of Venice); Andrea Critto (Centro Euro-Mediterraneo sui Cambiamenti Climatici (CMCC) & Ca' Foscari University of Venice) |
(48) Global High Resolution CO2 monitoring using Super Resolution | Andrianirina Rakotoharisoa (Imperial College London); Rossella Arcucci (Imperial College London) |
(49) DiffObs: Generative Diffusion for Global Forecasting of Satellite Observations | Jason Stock (Colorado State University); Jaideep Pathak (NVIDIA Corporation); Yair Cohen (NVIDIA Corporation); Mike Pritchard (NVIDIA Corporation); Piyush Garg (NVIDIA); Dale Durran (NVIDIA Corporation); Morteza Mardani (NVIDIA Corporation); Noah D Brenowitz (NVIDIA) |
(50) A cautionary tale about deep learning-based climate emulators | Björn Lütjens (Massachussets Institute of Technology); Raffaele Ferrari (Massachusetts Institute of Technology); Paolo Giani (Massachusetts Institute of Technology); Dava Newman (MIT); Andre Souza (Massachusetts Institute of Technology); Duncan Watson-Parris (University of California San Diego); Noelle Selin (Massachusetts Institute of Technology) |
(51) CausalPrompt: Enhancing LLMs with Weakly Supervised Causal Reasoning for Robust Performance in Non-Language Tasks | Tung-Wei Lin (University of California, Berkeley); Vanshaj Khattar (Virginia Tech); Yuxuan Huang (University College London); Junho Hong (University of Michigan); Ruoxi Jia (Virginia Tech); Chen-Ching Liu (Virginia Tech); Alberto L Sangiovanni-Vincentelli (University of California, Berkeley); Ming Jin (Virginia Tech) |
(52) Estimating the age of buildings from satellite and morphological features to create a pan-EU Digital Building Stock Model | Jeremias Wenzel (Universiteit Twente); Ana M. Martinez (European Commission - Joint Research Centre); Pietro Florio (European Commission - Joint Research Centre); Katarzyna Goch (Institute of Geography and Spatial Organization Polish Academy of Sciences) |
(53) Probabilistic electricity price forecasting through conformalized deep ensembles | Alessandro Brusaferri (National Research Council of Italy); Andrea Ballarino (National Research Council of Italy); Luigi Grossi (University of Parma); Fabrizio Laurini (University of Parma) |
(54) Interpretable Machine Learning for power systems: Establishing Confidence in SHapley Additive exPlanationS | Tabia Ahmad (University of Strathclyde); Robert Hamilton (Shell); Panagiotis Papadopoulos (University of Manchester); Samuel Chevalier (University of Vermont); Ilgiz Murzakhanov (Technical University of Denmark); Rahul Nellikkath (Technical University of Denmark); Jochen Bernhard Stiasny (Technical University of Denmark); Spyros Chatzivasileiadis (Technical University of Denmark) |
(55) DETECTION OF METEOROLOGICAL VARIABLES IN A WIND FARM INFLUENCING THE EXTREME WIND SPEED BY HETEROGENEOUS GRANGER CAUSALITY | Katerina Schindlerova (UniVie); Irene Schicker (Geos); Kejsi Hoxhallari (UniVie); Claudia Plant (University of Vienna, Austria) |
(56) Machine Learning for the Detection of Arctic Melt Ponds from Infrared Imagery | Marlena Reil (University of Osnabrück and University of Bremen, Institute of Environmental Physics); Gunnar Spreen (University of Bremen, Institute of Environmental Physics); Marcus Huntemann (University of Bremen, Institute of Environmental Physics); Lena Buth (Alfred Wegener Institute); Dennis Wilson (University of Toulouse, ISAE-Supaero) |
(57) Identifying Complex Dynamics of Power Grid Frequency | Xinyi Wen (Karlsruhe Institute of Technology); Ulrich Oberhofer (Karlsruhe Institute of Technology); Leonardo Rydin Gorjão (Norwegian University of Life Sciences); G.Cigdem YALCIN (Istanbul University); Veit Hagenmeyer (Karlsruhe Institute of Technology (KIT)); Benjamin Schäfer (Karlsruhe Institute of Technology) |
(58) Imbalance-aware Presence-only Loss Function for Species Distribution Modeling | Robin Zbinden (EPFL); Nina van Tiel (EPFL); Marc Rußwurm (Wageningen University); Devis Tuia (EPFL) |
(59) Deep Gaussian Processes and inversion for decision support in model-based climate change mitigation and adaptation problems | bertrand nortier (University of Exeter); daniel williamson (University of Exeter); mattia mancini (University of Exeter); amy binner (University of Exeter); brett day (University of Exeter); ian bateman (University of Exeter) |
(60) Generalized Policy Learning for Smart Grids: FL TRPO Approach | Yunxiang LI (MBZUAI); Nicolas M Cuadrado (MBZUAI); Samuel Horváth (MBZUAI); Martin Takac (Mohamed bin Zayed University of Artificial Intelligence) |
(61) A Deep Learning Framework to Efficiently Estimate Precipitation at the Convection Permitting Scale | Valentina Blasone (University of Trieste); Erika Coppola (Earth System Physics Section, ICTP, Trieste); Guido Sanguinetti (SISSA); Viplove Arora (Theoretical and Scientific Data Science, SISSA, Trieste); Serafina Di Gioia (Earth System Physics Section, ICTP, Trieste); Luca Bortolussi (University of Trieste) |
(62) Global Vegetation Modeling With Pre-Trained Weather Transformers | Pascal Janetzky (University Wuerzburg); Florian Gallusser (Universität Würzburg); Simon Hentschel (Julius-Maximilians-Universität of Würzburg); Andreas Hotho (University of Wuerzburg); Anna Krause (Universität Würzburg, Department of Computer Science, CHair X Data Science) |
(63) Building Ocean Climate Emulators | Adam Subel (New York University); Laure Zanna (New York University) |
(64) Neural Tree Reconstruction for the Open Forest Observatory | Marissa Ramirez de Chanlatte (UC Berkeley); Arjun Rewari (Darrell Group, Berkeley AI Research Lab); Trevor Darrell (UC Berkeley); Derek Young (University of California Davis) |
(65) Graph Neural Network Based Screening of Metal-Organic Frameworks for CO2 Capture | Zikri Bayraktar (Schlumberger Doll Research); Mengying Li (Schlumberger Doll Research); Shahnawaz Molla (Schlumberger Doll Research) |
(66) Predicting Species Occurrence Patterns from Partial Observations | Hager Radi Abdelwahed (Mila: Quebec AI Institute); Mélisande Teng (Mila, Université de Montréal); David Rolnick (MIT) |
(67) Towards Scalable Deep Species Distribution Modelling using Global Remote Sensing | Emily Morris (University of Cambridge); Anil Madhavapeddy (University of Cambridge); Sadiq Jaffer (University of Cambridge); David Coomes (University of Cambridge) |
Proposals
Title | Authors |
---|---|
(68) Valuation and Profit Allocation for Electric Vehicle Battery Data in a Data Market | Junkang Chen (Peking University); Guannan He (Peking University) |
(69) SkyImageNet: Towards a large-scale sky image dataset for solar power forecasting | Yuhao Nie (Massachusetts Institute of Technology); Quentin Paletta (European Space Research Institute); Sherrie Wang (MIT) |
(70) An Adaptive Hydropower Management Approach for Downstream Ecosystem Preservation | Cecília Coelho (University of Minho); Ming Jin (Virginia Tech); M. Fernanda P. Costa (Dep. Mathematics, University of Minho); Luís L. Ferrás (University of Porto) |
(71) A Benchmark Dataset for Meteorological Downscaling | Michael Langguth (Juelich Supercomputing Centre - Forschungszentrum Juelich); Paula Harder (Mila); Irene Schicker (Geos); Ankit Patnala (Juelich Supercomputing Centre - Forschungszentrum Juelich); Sebastian Lehner (GeoSphere Austria); Konrad Mayer (GeoSphere Austria); Markus Dabernig (GeoSphere Austria) |
(72) Enabling Clean Energy Resilience with Machine Learning-Empowered Underground Hydrogen Storage | Alvaro R Carbonero Gonzales (Los Alamos National Lab); Shaowen Mao (Los Alamos National Laboratory); Mohamed Mehana (Los Alamos National Lab) |
(73) Adjustment of ocean carbon sink predictions with an emission-driven Earth system model using a deep neural network | Reinel Sospedra-Alfonso (Environment and Climate Change Canada); Parsa Gooya (Environment and Climate Change Canada); Johannes Exenberger (Graz University of Technology) |
(74) Calibrating Earth System Models with Bayesian Optimal Experimental Design | Tim Reichelt (University of Oxford); Shahine Bouabid (University of Oxford); Luke Ong (University of Oxford); Duncan Watson-Parris (University of California San Diego); Tom Rainforth (University of Oxford) |
(75) Severe Wind Event Prediction with Multivariate Physics-Informed Deep Learning | Willa Potosnak (Carnegie Mellon University); Cristian I Challu (Carnegie Mellon University); Kin G. Olivares (Carnegie Mellon University); James K Miller (Carnegie Mellon University); Artur Dubrawski (Carnegie Mellon University) |
Tutorials
Title | Authors |
---|---|
(76) Empowering Safe Reinforcement Learning in Power System Control with CommonPower | Hannah Markgraf (Technical University of Munich); Michael Eichelbeck (Technical University of Munich); Matthias Althoff (Technical University of Munich) |
(77) Planning for Floods & Droughts: Intro to AI-Driven Hydrological Modeling | Kshitij Tayal (Oak Ridge National Lab); Arvind Renganathan (University of Minnesota); Dan Lu (Oak Ridge National Laboratory) |
(78) Understanding drivers of climate extremes using regime-specific causal graphs | Oana-Iuliana Popescu (Institute of Data Science, German Aerospace Center (DLR)); Wiebke Günther (German Aerospace Center); Raed Hamed (Institute for Environmental Studies, VU Amsterdam); Dominik Schumacher (4Institute for Atmospheric and Climate Science, ETH Zürich); Martin Rabel (DLR); Dim Coumou (IVM/VU); Jakob Runge (Institute of Data Science, German Aerospace Center (DLR)) |
(79) One Prompt Fits All: Visual Prompt-Tuning for Remote Sensing Segmentation Audience Choice | Marshall Wang (Vector Institute); John Willes (Vector Institute); Deval Pandya (Vector Institute) |
(80) 1. Building Sustainable Futures: Tutorial on Carbon Footprint Analysis and Mitigation Strategies Using Counter Factual Queries | Kumar Saurav (IBM); Manikandan Padmanaban (IBM Research India); Ayush Jain (IBM Research); Jagabondhu Hazra (IBM Research India) |
Call for Submissions
We invite submissions of short papers using machine learning to address problems in climate mitigation, adaptation, or science, including but not limited to the following topics:
- Agriculture and food
- Behavioural and social science
- Buildings
- Carbon capture and sequestration
- Cities and urban planning
- Climate finance and economics
- Climate justice
- Climate science and climate modeling
- Disaster management and relief
- Earth observations and monitoring
- Earth science
- Ecosystems and biodiversity
- Extreme weather
- Forestry and other land use
- Health
- Heavy industry and manufacturing
- Local and indigenous knowledge systems
- Materials science and discovery
- Oceans and marine systems
- Power and energy systems
- Public policy
- Societal adaptation and resilience
- Supply chains
- Transportation
All machine learning techniques are welcome, from kernel methods to deep learning. Each submission should make clear why the application has (or could have) a pathway to positive impacts regarding climate change. We highly encourage submissions which make their data and code publicly available. Accepted submissions will be invited to give poster presentations, of which some will be selected for spotlight talks.
The workshop does not publish proceedings, and submissions are non-archival. Submission to this workshop does not preclude future publication. Previously published work may be submitted under certain circumstances (see the FAQ).
All papers and proposals submissions must be through the submission website. Submissions will be reviewed double-blind; do your best to anonymize your submission, and do not include identifying information for authors in the PDF. Authors are required to use the workshop style template (based on the ICLR style files), available for LaTeX.
All tutorial submissions must be through the submission website.
Please see the Tips for Submissions and FAQ, and contact climatechangeai.iclr2024@gmail.com with questions.
Submission Tracks
There are three tracks for submissions: (i) Papers, (ii) Proposals and (iii) Tutorials. Submissions are limited to 4 pages for the Papers track, and 3 pages for the Proposals track, in PDF format (see examples from previous workshops here). References do not count towards this total. Supplementary appendices are allowed but will be read at the discretion of the reviewers. All submissions must explain why the proposed work has (or could have) positive impacts regarding climate change.
PAPERS Track
Work that is in progress, published, and/or deployed.
Submissions for the Papers track should describe projects relevant to climate change that involve machine learning. These may include (but are not limited to) academic research; deployed results from startups, industry, public institutions, etc.; and climate-relevant datasets.
Submissions should provide experimental or theoretical validation of the method presented, as well as specifying what gap the method fills. Authors should clearly illustrate a pathway to climate impact, i.e., identify the way in which this work fits into broader efforts to address climate change. Algorithms need not be novel from a machine learning perspective if they are applied in a novel setting. Details of methodology need not be revealed if they are proprietary, though transparency is highly encouraged.
Submissions creating novel datasets are welcome. Datasets should be properly documented with regards to their provenance and contents and designed to permit machine learning research (e.g. formatted with clear benchmarks for evaluation).
PROPOSALS Track
Early-stage work and detailed descriptions of ideas for future work.
Submissions for the Proposals track should describe detailed ideas for how machine learning can be used to solve climate-relevant problems. While less constrained than the Papers track, Proposals will be subject to a very high standard of review. Ideas should be justified as extensively as possible, including motivation for why the problem being solved is important in tackling climate change, discussion of why current methods are inadequate, explanation of the proposed method, and discussion of the pathway to climate impact. Preliminary results are optional.
TUTORIALS Track
Interactive notebooks for insightful step-by-step walkthroughs
Submissions for the Tutorials track should demonstrate the use of machine learning methods and tools (e.g. libraries, packages, services, datasets, or frameworks) to address climate-relevant challenges.
Tutorial proposals will be due January 10, 2024, and should take the form of an abstract that includes a clear and concise description of what users can expect to learn. Proposal submissions will be reviewed based on their potential impact and usability by the climate and AI research community. Submissions will also be assessed based on the originality of the problem space. Please review our list of CCAI tutorials to minimize substantial overlap with existing tutorials.
For accepted proposals, the first iteration of the tutorial will be due February 18, 2024 and should be in the form of executable notebooks that follows CCAI’s Tutorial Template. Authors must submit their notebook in a self-contained runnable environment (e.g. Colab, Binder) to allow users to easily access and run the tutorial. We expect tutorials to be 75-90% complete by this first deadline in order for our reviewers to provide adequate feedback and select tutorials for spotlight presentations. Tutorials accepted during this round will be invited to make final submissions by April 7, 2024.
Notebook submissions will be assessed based on clarity, accessibility, and code quality. We ask that authors emphasize real-world impacts of their ML models by answering questions such as Who will be using the models/outputs and how will they be used? What decisions will be made based on these models? How will this impact existing systems/the environment/affected communities on the ground? For more information please review our tutorial development guidelines.
Tips for Submissions
- For examples of typical formatting and content, see submissions from our previous workshops.
- Be explicit: Describe how your proposed approach addresses climate change, demonstrating an understanding of the application area.
- Frame your work: The specific problem and/or data proposed should be contextualized in terms of prior work.
- Address the impact: Describe the practical implications of your method in addressing the problem you identify, as well as any relevant societal impacts or potential side-effects. We recommend reading our further guidelines on this aspect here.
- Explain the ML: Readers may not be familiar with the exact techniques you are using or may desire further detail.
- Justify the ML: Describe why the ML method involved is needed, and why it is a good match for the problem.
- Avoid jargon: Jargon is sometimes unavoidable but should be minimized. Ideal submissions will be accessible both to an ML audience and to experts in other relevant fields, without the need for field-specific knowledge. Feel free to direct readers to accessible overviews or review articles for background, where it is impossible to include context directly.
Addressing Impact
Tackling climate change requires translating ideas into action. The guidelines below will help you clearly present the importance of your work to a broad audience, hopefully including relevant decision-makers in industry, government, nonprofits, and other areas.
- Illustrate the link: Many types of work, from highly theoretical to deeply applied, can have clear pathways to climate impact. Some links may be direct, such as improving solar forecasting to increase utilization within existing electric grids. Others may take several steps to explain, such as improving computer vision techniques for classifying clouds, which could help climate scientists seeking to understand fundamental climate dynamics.
- Consider your target audience: Try to convey with relative specificity why and to whom solving the problem at hand will be useful. If studying extreme weather prediction, consider how you would communicate your key findings to a government disaster response agency. If analyzing a supply chain optimization pilot program, what are the main takeaways for industries who might adopt this technology? To ensure your work will be impactful, where possible we recommend co-developing projects with relevant stakeholders or reaching out to them early in the process for feedback. We encourage you to use this opportunity to do so!
- Outline key metrics: Quantitative or qualitative assessments of how well your results (or for proposals, anticipated results) compare to existing methods are encouraged. Try to give a sense of the importance of your problem and your findings. We encourage you to convey why the particular metrics you choose are relevant from a climate change perspective. For instance, if you are evaluating your machine learning model on the basis of accuracy, how does improved accuracy on a machine learning model translate to climate impact, and why is accuracy the best metric to use in this context?
- Be clear and concise: The discussion of impact does not need to be lengthy, just clear.
- Convey the big picture: Ultimately, the goal of Climate Change AI is to “empower work that meaningfully addresses the climate crisis.” Try to make sure that from the beginning, you contextualize your method and its impacts in terms of this objective.
Interactive Q&A
If you have further questions on how to participate in the workshop, you can ask those directly via the interactive Q&A session hosted on our community platform. You can also contact us via email at climatechangeai.iclr2024@gmail.com. For recordings of informational webinars of previous editions of our workshop, please see here.
Organizers
Shiva Madadkhani (Technical University of Munich)
Arthur Ouaknine (McGill, Mila)
Rasika Bhalerao (Northeastern University)
Millie Chapman (National Center for Ecological Analysis and Synthesis)
Jesse Dunietz (Climate Change AI)
Nikola Milojevic-Dupont (MCC Berlin, Technical University of Berlin)
Olivia Mendivil Ramos (Climate Change AI)
David Rolnick (McGill, Mila)
Yoshua Bengio (Mila, UdeM)
Tutorials Track Organizers
Isabelle Tingzon (The World Bank/GFDRR)
Nadia Ahmed (UC Irvine)
Shafat Rahman (University of Southern California)
Mentors
Adam Boyd (US Department of Energy)
Ahmed Gomaa (Cairo University)
Akshata Kishore Moharir (Microsoft)
Akshay Iyer (SkySpecs)
Alex Lew Wen Jie (Chulalongkorn University, ClimateShares, Environman)
Ali Ahmadalipour (Google[X])
Angelos Chronis (Infrared City GmbH, Austrian Institute of Technology GmbH)
Arjan Goemé (EnerGyPT)
Dar Mehta (Climatix AI)
Defu Cao (University of Southern California)
Dennis Wilson (University of Toulouse)
Elie Alhajjar (RAND)
Gowdhaman Sadhasivam (Orbital Insight)
Houlong Zhuang (Arizona State University)
Kitgak Simon (United Nations Sustainable Development Solutions Network (SDSN))
Manja Sachet (Wilson Sonsini Goodrich & Rosati)
Mikolaj Czerkawski (European Space Agency)
Ming Jin (Virginia Tech)
Mohamed Jendoubi (Uluumy)
Moiz Khan Sherwani (University of Copenhagen)
Nicole Hu (One Concern, Inc)
Nino Lazariia (Cleantech Group)
Pandarasamy Arjunan (Indian Institute of Science)
Praveen Pankajakshan (Cropin AI Lab)
Priyanka Dwivedi (Deep Learning Analytics)
Tanmay Laud (Megagon Labs)
Tayebwa Philips (Healing Health Healing Hearts)
Ying-Jung Chen (Georgia Institute of Technology /Open Geospatial Consortium)
Program Committee
Abhinav Prakash Gahlot (Georgia Institute of Technology)
Akshit Gupta (Delft University of Technology)
Alberto Chapchap (GS Cap)
Alexander Pondaven (University of Oxford)
Alison Peard (University of Oxford)
Alona Zharova (Humboldt University of Berlin)
Andrew Byrnes (Dun and Bradstreet)
Andrew Ross (Harvard University)
Anna Kwa (Allen Institute for Artificial Intelligence)
Antoine Blanchard (Verisk)
Bedartha Goswami (University of Tübingen)
Bertrand Le Saux (ESA, Phi-lab)
Bharathan Balaji (Amazon)
Bilge Acun (Meta, FAIR)
Björn Lütjens (MIT)
Cesar Quilodran-Casas (Imperial College London)
Chace Ashcraft (JHU, APL)
Charles Anderson (Colorado State University)
Christian Lessig (Otto-von-Guericke-Universitat Magdeburg)
Christian Reimers (Department of Biogeochemical Integration, Max Planck Institute for Biogeochemistry)
Christina Winkler (Mila)
Christopher Krapu (Duke University)
Core Francisco Park (Harvard University)
Dan Lu (Oak Ridge National Laboratory)
Danica Vukadinovic Greetham (Capgemini Engineering)
Daniel Salles Civitarese (IBM Research, Brazil)
Dara Farrell (Graduate of University of Washington)
David Dao (ETH Zurich)
David Rolnick (McGill University, Mila)
David Russell (Carnegie Mellon University)
Dennis Wilson (ISAE)
Difan Zhang (PNNL)
Donna Vakalis (Universite de Montreal, Mila)
Edgar Ramirez Sanchez (MIT)
Elena Fillola (University of Bristol)
Eren Gultepe (Southern Illinois University Edwardsville)
Faroq ALTam (Elm Company)
Fiona Spuler (University of Reading)
Florian Nachtigall (Technical University of Berlin)
Gabriel Kasmi (Mines Paris, PSL)
Gabriel Tseng (NASA Harvest)
Gabriela Gongora-Svartzman (Carnegie Mellon University)
Gege Wen (Stanford University)
Guanting Chen (The University of North Carolina at Chapel Hill)
Hannah Kerner (Arizona State University)
Hanyu Zhang (Georgia Institute of Technology)
Hari Prasanna Das (UC Berkeley)
Himanshu Sharma (Pacific Northwest National Laboratory)
Hovig Bayandorian
Ilaria Luise (CERN)
Ilias Tsoumas (National Observatory of Athens)
Isabelle Tingzon (The World Bank/GFDRR)
James Tlhomole (Imperial College London)
Jeff Clark (University of Bristol)
Jennifer Sleeman (University of Maryland, Baltimore County and Johns Hopkins University Applied Physics Laboratory)
Jeremy Irvin (Stanford)
Jerry Lin (University of California, Irvine)
Ji Hun Wang (Stanford University)
Jimeng Shi (Florida International University)
Joel Oskarsson (Linköping University)
Johannes Schmude (IBM)
Jonathan Fürst (ZHAW Zurich University of Applied Sciences)
Jorge Montalvo Arvizu (Centrica)
Joris Guerin (LAAS-CNRS)
Jose Cordova-Garcia (ESPOL)
Joyjit Chatterjee (University of Hull)
Julien Boussard (Columbia University)
Kate Duffy (BAER Institute, NASA)
Katharina Prasse (University of Siegen)
Ken C. L. Wong (IBM Research, Almaden Research Center)
Kevin McCloskey (Google)
Kim Bente (The University of Sydney)
Konstantin Klemmer (Microsoft Research)
Kris Sankaran (University of Wisconsin-Madison)
Kyle Bradbury (Duke University)
Kyongsik Yun (California Institute of Technology)
Lea Boche (EPRI)
Lorenzo Basile (University of Trieste)
Lucas Rosenblatt (NYU)
Lucas Spangher (MIT PSFC)
Mahmoud AL NAJAR (Laboratory of Spatial Geophysics and Oceanography Studies)
Marc Rußwurm (Wageningen University)
Marcus Voss (TU Berlin)
Markus Leippold (University of Zurich)
Mathilde Letard (University of Rennes)
Matias Quintana (National University of Singapore)
Matthias Bittner (Vienna University of Technology)
Matthias Hertel (KIT)
Maya Janvier (Centrale Supélec)
Meareg Hailemariam (Climate Change AI)
Melanie Hanna (Climate Change AI)
Mengze Wang (Massachusetts Institute of Technology)
Michael Smith (Aspia Space)
Miguel Molina-Solana (Universidad de Granada)
Milan Jain (PNNL)
Mrigi Munjal (Massachusetts Institute of Technology)
Nadia Ahmed (UC Irvine)
Nicole Ludwig (University of Tübingen)
Nikola Milojevic-Dupont (Mercator Research Institute on Global Commons and Climate Change (MCC))
Olalekan Akintande (University of Ibadan)
Omar Younis (ETH Zurich)
Pallavi Banerjee (University of Washington)
Paul Griffiths (National Centre for Atmospheric Science, Cambridge University)
Paweł Gora (TensorCell)
Peer Nowack (Karlsruhe Institute of Technology)
Peter Watson (Bristol)
Priya Donti (MIT)
Qiao Kang (Memorial University)
Redouane Lguensat (IPSL)
Rodrigo Rene Rai Munoz Abujder (Johns Hopkins Applied Physics Laboratory)
Ruben Glatt (Lawrence Livermore National Laboratory)
Sagar Pande (UCLA)
Sandeep Chinta (Massachusetts Institute of Technology)
Sara El Mekkaoui (DNV)
Sasha Luccioni (Hugging Face)
Sebastian Ruf (Northeastern University)
Shafat Rahman (University of Southern California)
Siming Bayer (Pattern Recognition Lab, Friedrich-Alexander University)
Sojung An (Korea Institute of Atmospheric Prediction Systems)
Soumyendu Sarkar (Hewlett Packard Enterprise)
Stefan Feuerriegel (LMU Munich)
Sudipan Saha (Indian Institute of Technology Delhi)
Suraj R Nair (UC Berkeley)
Swati Sharma (Microsoft Research)
Tarek Alskaif (Wageningen University)
Tejasri Nampally (Indian Institute of Technology Hyderabad Telangana India)
Theodore Wolf (Carbon Re)
Thomas Asikis (University of Zurich)
Ting-Yu Dai (The University of Texas at Austin)
Usman Nazir (Lahore University of Management Sciences)
Victoria Preston (Northeastern University)
Vili Hätönen (Emblica)
Vladimir Dvorkin (University of Michigan)
Xiaojuan Liu (Climate Change AI)
Xiaolong Tu (Georgia State University)
Yanru Zhang (University of Electronic Science and Technology of China)
Yimeng Min (Cornell University)
Ying-Jung Deweese (Descartes Labs, Georgia Insititute of Technology)
Yixuan Sun (Argonne National Laboratory)
Zikri Bayraktar (Schlumberger Doll Research)
Ziyang Jiang (Duke University)
Mentorship Program
We are hosting a mentorship program to facilitate exchange between potential workshop submitters and experts working in topic areas relevant to the workshop. The goal of this program is to foster cross-disciplinary collaborations and ultimately increase the quality and potential impact of submitted work.
Expectations
Mentors are expected to guide mentees during the CCAI mentorship program as they prepare submissions for this workshop.
Examples of mentor-mentee interactions may include:
- In-depth discussion of relevant related work in the area of the Paper or Proposal, to ensure submissions are well-framed and contextualized in terms of prior work.
- Iterating on the core idea of a Proposal to ensure that the climate change application is well-posed and the ML techniques used are well-suited.
- Giving feedback on the writing or presentation of a Paper or Proposal to bring it to the right level of maturity for submission.
Mentees are expected to initiate contact with their assigned mentor and put in the work and effort necessary to prepare a Paper or Proposal submission by Feb 3.
We suggest that after the mentor-mentee matching is made, a first (physical or digital) meeting should take place within the first week (Jan 10-14) to discuss the Paper or Proposal and set expectations for the mentorship period. Subsequent interactions can take place either through meetings or via email discussions, following the expectations set during the initial meeting, culminating in a final version of a Paper or Proposal submitted via the CMT portal by Feb 3.
Mentors and mentees must abide by the Climate Change AI Code of Conduct: https://www.climatechange.ai/code_of_conduct.
Application
Applications are due by Jan 3 Jan 6.
Sponsors
Silver Sponsor
Bronze Sponsors
Frequently Asked Questions
Mentorship Program FAQ
Q: Are mentors allowed to be authors on the paper for which they provided mentorship?
A: Yes, mentors can be co-authors but not reviewers.
Q: What happens if the mentor/mentee does not fulfill their duties, or if major issues come up?
A: Please email us at climatechangeai.iclr2024@gmail.com and we will do our best to help resolve the situation. Potential breaches of the Code of Conduct will be responded to promptly as detailed therein.
Q: What happens if I apply to be a mentee but do not get paired with a mentor?
A: While we will do our best, we cannot guarantee pairings for everyone. Even if you do not get paired with a mentor, we encourage you to submit a Paper or Proposal to the workshop, and our reviewers will provide you with guidance and feedback on how to improve it.
Q: What happens if my submission does not get accepted to the workshop?
A: While the mentorship program is meant to give early-career researchers and students the opportunity to improve the quality of their work, sometimes submissions will need further polishing and elaboration before being ready for presentation at a CCAI workshop. If this is the case, we invite you to take into account the comments made by the reviewers and to resubmit again to a subsequent CCAI workshop.
Q: I cannot guarantee that I can commit at least 4 hours to the program over the time period. Should I still apply as a mentor?
A: No. While the 4 hour time commitment is a suggestion, we do believe that it is necessary to ensure that all mentees receive the help and guidance they need.
Q: I do not have a background in machine learning; can I still apply to be a mentor/mentee?
A: Yes! We welcome applications from domains that are complementary to machine learning to solve the problems that we are targeting.
Q: What happens if my mentor/mentee wants to continue meeting after the workshop?
A: We welcome and encourage continued interactions after the official mentorship period. That said, neither the mentor nor the mentee should feel obligated to maintain contact.
Submission FAQ
Q: How can I keep up to date on this kind of stuff?
A: Sign up for our mailing list!
Q: I’m not in machine learning. Can I still submit?
A: Yes, absolutely! We welcome submissions from many fields. Do bear in mind, however, that the majority of attendees of the workshop will have a machine learning background; therefore, other fields should be introduced sufficiently to provide context for the work.
Q: What if my submission is accepted but I can’t attend the workshop?
A: You may ask someone else to present your work in your stead.
Q: It’s hard for me to fit my submission on 3 or 4 pages. What should I do?
A: Feel free to include appendices with additional material (these should be part of the same PDF file as the main submission). Do not, however, put essential material in an appendix, as it will be read at the discretion of the reviewers.
Q: Can I send submissions directly by email?
A: No, please use the CMT website to make submissions.
Q: The submission website is asking for my name. Is this a problem for anonymization?
A: You should fill out your name and other info when asked on the submission website; CMT will keep your submission anonymous to reviewers.
Q: Do submissions for the Proposals track need to have experimental validation?
A: No, although some initial experiments or citation of published results would strengthen your submission.
Q: The submission website never sent me a confirmation email. Is this a problem?
A: No, the CMT system does not send automatic confirmation emails after a submission, though the submission should show up on the CMT page once submitted. If in any doubt regarding the submission process, please contact the organizers. Also please avoid making multiple submissions of the same article to CMT.
Q: Can I submit previously published work to this workshop?
A: Yes, though under limited circumstances. In particular, work that has previously been published at non-machine learning venues may be eligible for submission; however, work that has been published in conferences on machine learning or related fields is likely not eligible. If your work was previously accepted to a Climate Change AI workshop, this work should have changed or matured substantively to be eligible for resubmission. Please contact climatechangeai.iclr2024@gmail.com with any questions.
Q: Can I submit work to this workshop if I am also submitting to another ICLR 2024 workshop?
A: Yes. We cannot, however, guarantee that you will not be expected to present the material at a time that conflicts with the other workshop.