NeurIPS 2023 Workshop: Tackling Climate Change with Machine Learning
About
Many in the ML community wish to take action on climate change, but are unsure of the pathways through which they can have the most impact. This workshop highlights work that demonstrates that, while no silver bullet, ML can be an invaluable tool in reducing greenhouse gas emissions and in helping society adapt to the effects of climate change. Climate change is a complex problem, for which action takes many forms - from theoretical advances to deployment of new technology. Many of these actions represent high-impact opportunities for real-world change, and are simultaneously interesting academic research problems.
This workshop was held as part of the Conference on Neural Information Processing Systems (NeurIPS) 2023 in New Orleans, Louisiana, United States. This iteration of the workshop was focused on the theme of blending new and existing knowledge systems to invite and inspire submissions that consider how a machine learning advancement can leverage and integrate existing knowledge.
About the Workshop
The main workshop took place on December 16, 2023. The schedule is available below, with links to papers, videos, and slides.
Schedule
Speakers
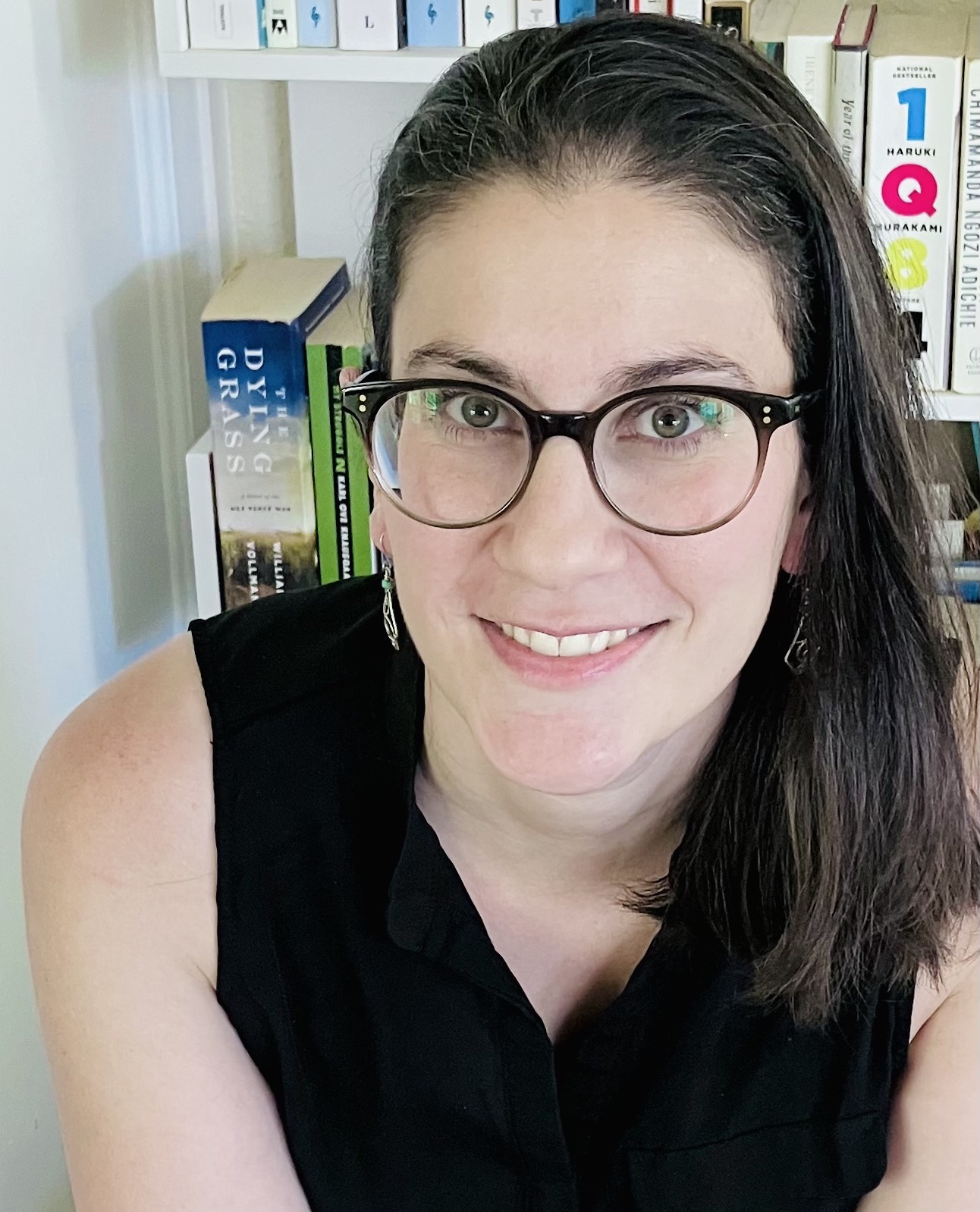
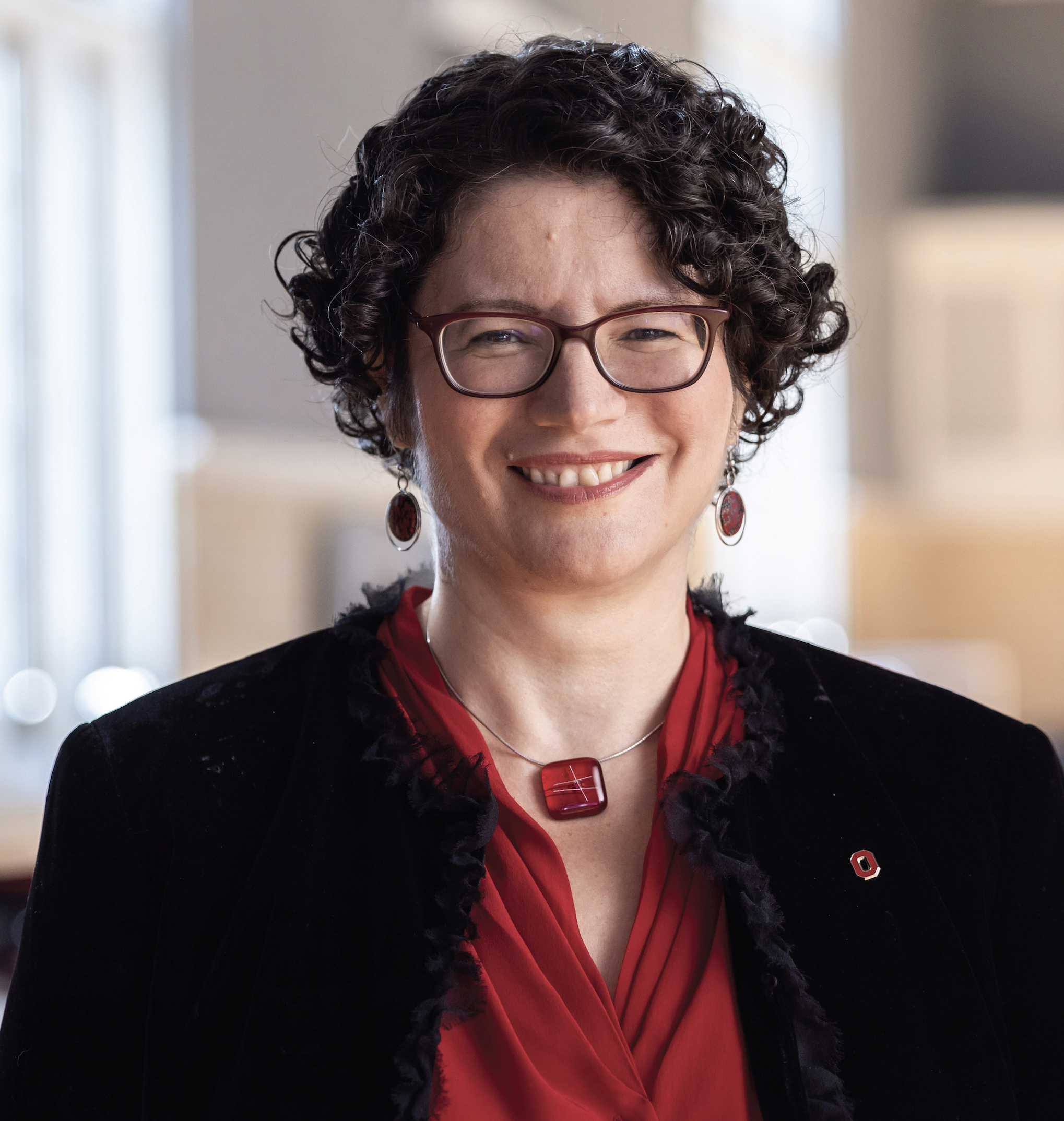
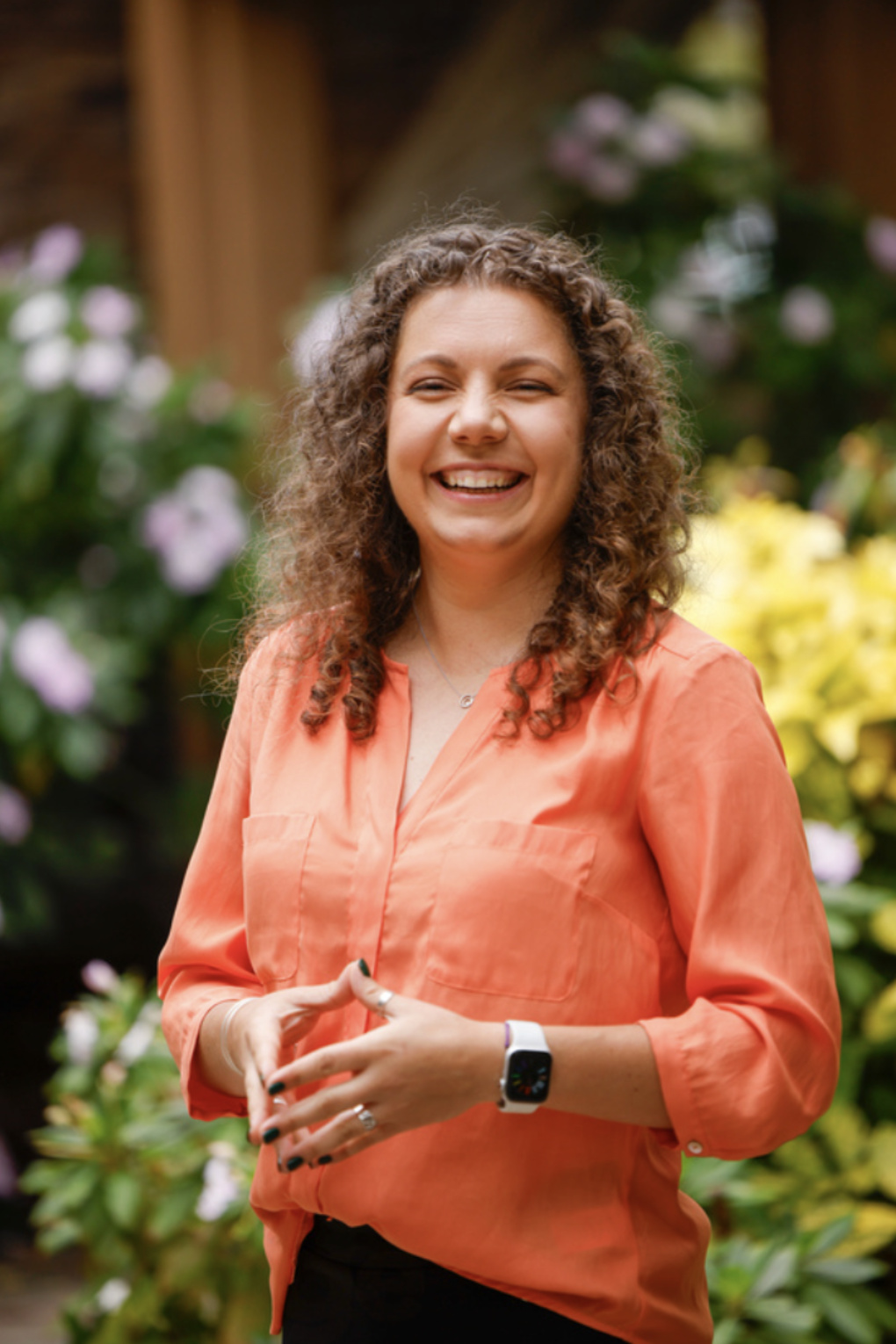
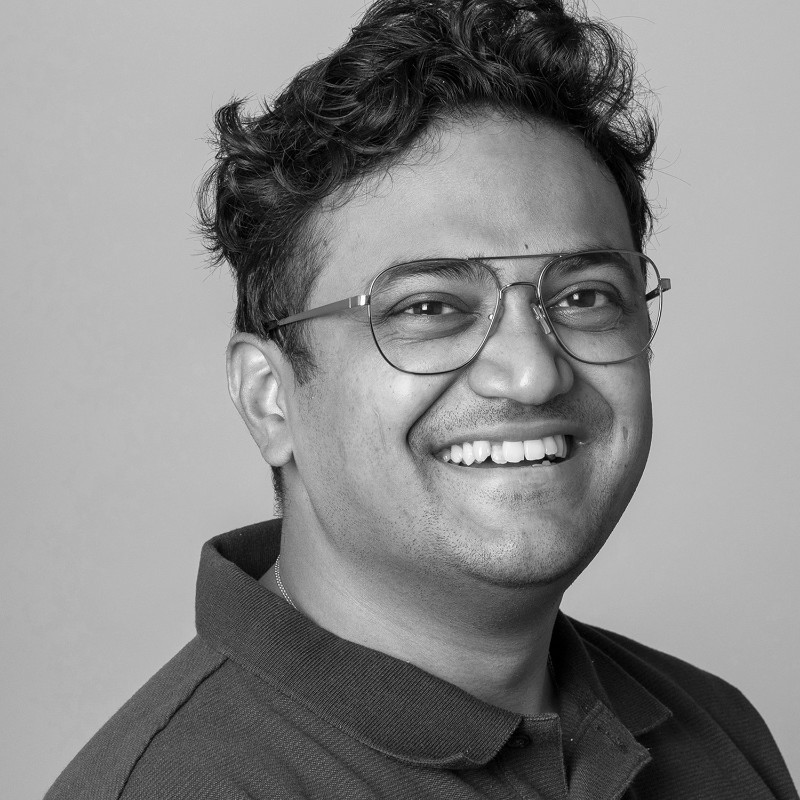
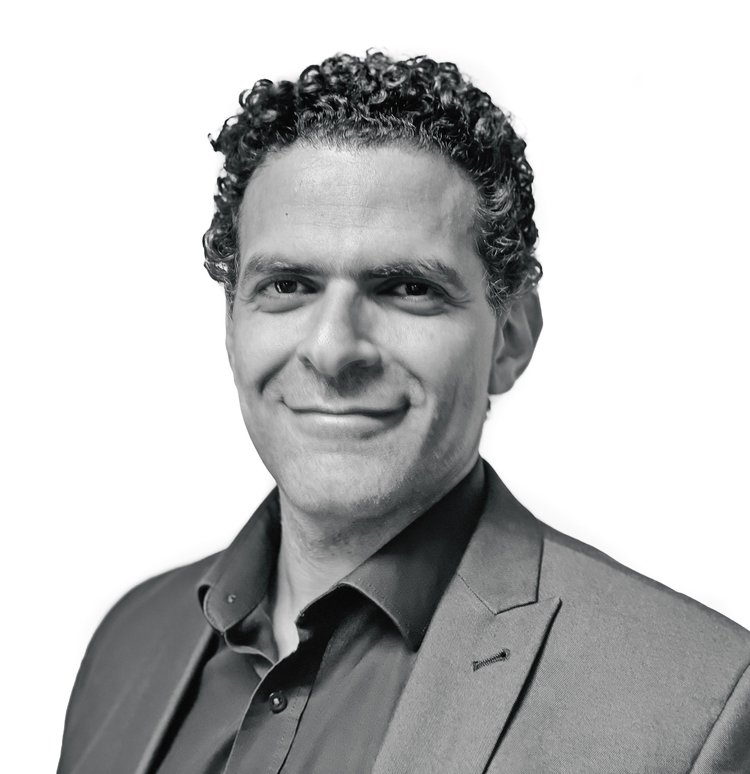
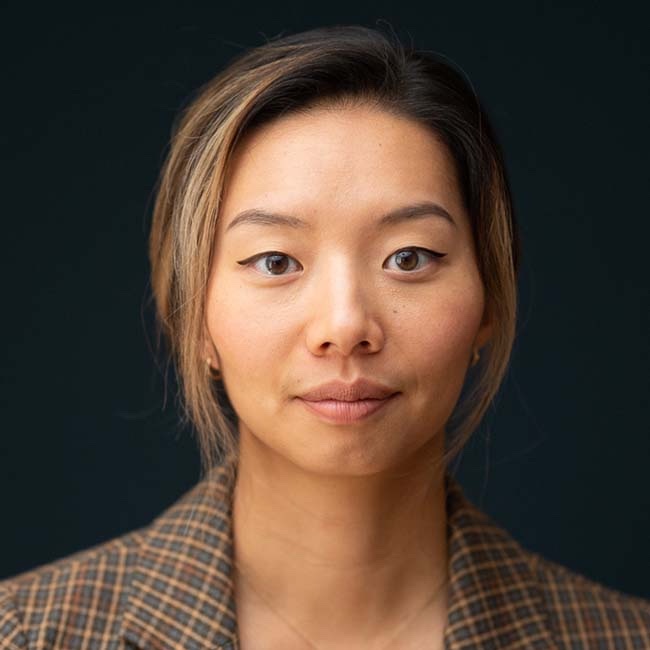
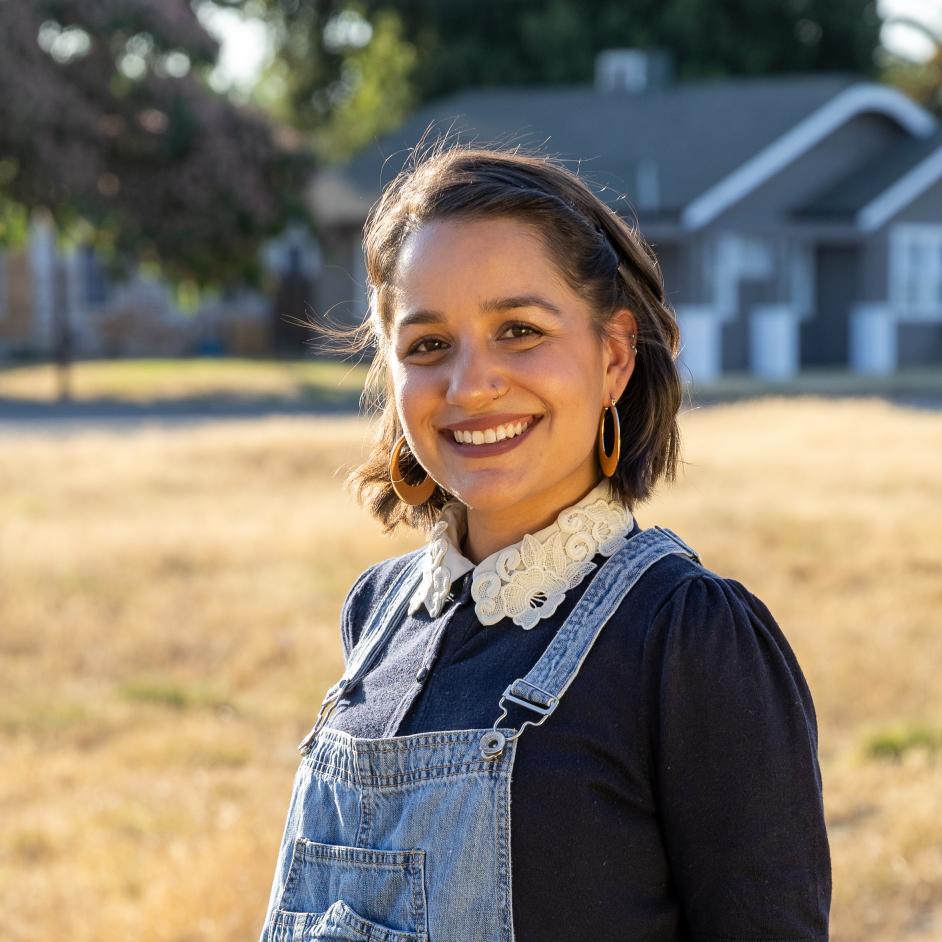
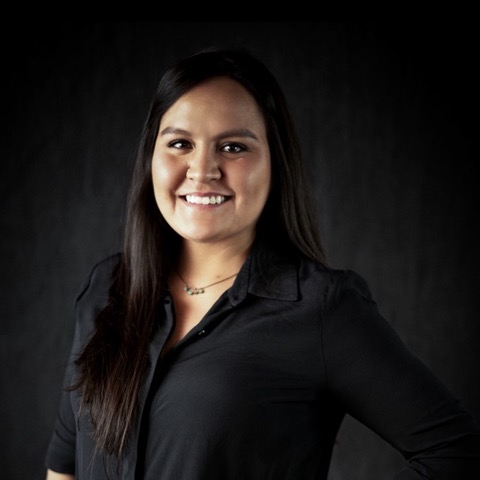
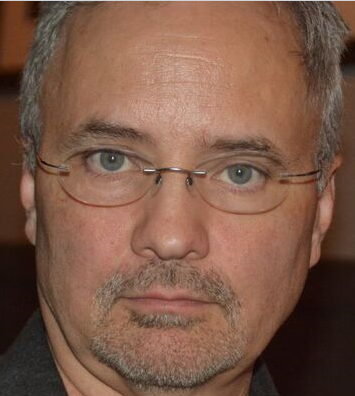
Accepted Works
Works were submitted to one of three tracks: Papers, Proposals, or Tutorials.
Click the links below for information about each submission, including slides, videos, and papers.
Papers
Title | Authors |
---|---|
(1) Machine learning for gap-filling in greenhouse gas emissions databases | Luke Cullen (University of Cambridge); Andrea Marinoni (UiT the Arctic University of Norway); Jonathan M Cullen (University of Cambridge) |
(2) EarthPT: a foundation model for Earth Observation | Michael J Smith (Aspia Space); Luke Fleming (Aspia Space); James Geach (Aspia Space) |
(3) Towards Understanding Climate Change Perceptions: A Social Media Dataset | Katharina Prasse (University of Siegen); Steffen Jung (MPII); Isaac B Bravo (Technische Universität München); Stefanie Walter (Technical University of Munich); Margret Keuper (University of Siegen, Max Planck Institute for Informatics) |
(4) Adaptive-Labeling for Enhancing Remote Sensing Cloud Understanding | Jay Gala (NMIMS); Sauradip Nag (University of Surrey); Huichou Huang (City University of Hong Kong); Ruirui Liu (Brunel University London); Xiatian Zhu (University of Surrey) |
(5) Flamingo: Environmental Impact Factor Matching for Life Cycle Assessment with Zero-Shot ML | Bharathan Balaji (Amazon); Venkata Sai Gargeya Vunnava (amazon); Nina Domingo (Amazon); Shikhar Gupta (Amazon); Harsh Gupta (Amazon); Geoffrey Guest (Amazon); Aravind Srinivasan (Amazon); Kellen Axten (Amazon); Jared Kramer (Amazon) |
(6) AtmoRep: A stochastic model of atmosphere dynamics using large scale representation learning | ilaria luise (CERN) |
(7) Artificial Intelligence for Methane Mitigation : Through an Automated Determination of Oil and Gas Methane Emissions Profiles | Jade Eva Guisiano (Sorbonne / ISEP / Polytechnique / UNEP); Thomas LAUVAUX (Université de Reims); Eric Moulines (Ecole Polytechnique); Jérémie Sublime (ISEP) |
(8) Augmenting Ground-Level PM2.5 Prediction via Kriging-Based Pseudo-Label Generation | lei Duan (Duke University); Ziyang Jiang (Duke University); David Carlson (Duke University) |
(9) Physics-Informed Domain-Aware Atmospheric Radiative Transfer Emulator for All Sky Conditions | Piyush Garg (Argonne National Laboratory); Emil Constantinescu (Argonne National Laboratory); Bethany Lusch (Argonne National Lab); Troy Arcomano (Argonne National Laboratory); Jiali Wang (Argonne National Laboratory); Rao Kotamarthi (Argonne National Laboratory) |
(10) Can We Reliably Improve the Robustness to Image Acquisition of Remote Sensing of PV Systems? | Gabriel Kasmi (Mines Paris - PSL); Laurent Dubus (RTE France); Yves-Marie Saint-Drenan (Mines Paris - PSL); Philippe Blanc (Mines Paris - PSL) |
(11) Uncertainty Quantification of the Madden–Julian Oscillation with Gaussian Processes | Haoyuan Chen (Texas A&M University); Emil Constantinescu (Argonne National Laboratory); Vishwas Rao (Argonne National Laboratory); Cristiana Stan (George Mason University) |
(12) Ocean Wave Energy: Optimizing Reinforcement Learning Agents for Effective Deployment | Vineet Gundecha (Hewlett Packard Enterpise); Sahand Ghorbanpour (Hewlett Packard Enterprise); Ashwin Ramesh Babu (Hewlett Packard Enterprise Labs); Avisek Naug (Hewlett Packard Enterprise); Alexandre Pichard (Carnegie Clean Energy); mathieu Cocho (Carnegie Clean Energy); Soumyendu Sarkar (Hewlett Packard Enterprise) |
(13) Sustainable Data Center Modeling: A Multi-Agent Reinforcement Learning Benchmark | Soumyendu Sarkar (Hewlett Packard Enterprise); Avisek Naug (Hewlett Packard Enterprise); Antonio Guillen (Hewlett Packard Enterprise); Ricardo Luna Gutierrez (Hewlett Packard Enterprise); Vineet Gundecha (Hewlett Packard Enterpise); Sahand Ghorbanpour (Hewlett Packard Enterprise); Sajad Mousavi (Hewlett Packard Enterprise); Ashwin Ramesh Babu (Hewlett Packard Enterprise Labs) |
(14) ACE: A fast, skillful learned global atmospheric model for climate prediction | Oliver Watt-Meyer (Allen Institute for AI); Gideon Dresdner (Allen Institute for AI Climate Science); Jeremy McGibbon (Allen Institute for AI); Spencer K Clark (Allen Institute for Artificial Intelligence); James Duncan (University of California, Berkeley); Brian Henn (Allen Institute for AI); Matthew Peters (AI2); Noah D Brenowitz (NVIDIA); Karthik Kashinath (NVIDIA); Mike Pritchard (NVIDIA); Boris Bonev (NVIDIA); Christopher Bretherton (Allen Institute for AI) |
(15) A Configurable Pythonic Data Center Model for Sustainable Cooling and ML Integration | Avisek Naug (Hewlett Packard Enterprise); Antonio Guillen (Hewlett Packard Enterprise); Ricardo Luna Gutierrez (Hewlett Packard Enterprise); Vineet Gundecha (Hewlett Packard Enterpise); Sahand Ghorbanpour (Hewlett Packard Enterprise); Sajad Mousavi (Hewlett Packard Enterprise); Ashwin Ramesh Babu (Hewlett Packard Enterprise Labs); Soumyendu Sarkar (Hewlett Packard Enterprise) |
(16) Mapping Housing Stock Characteristics from Drone Images for Climate Resilience in the Caribbean | Isabelle Tingzon (The World Bank); Nuala Margaret Cowan (The World Bank); Pierre Chrzanowski (The World Bank) |
(17) Graph-based Neural Weather Prediction for Limited Area Modeling | Joel Oskarsson (Linköping University); Tomas Landelius (SMHI); Fredrik Lindsten (Linköping University) |
(18) Self-supervised Pre-training for Precipitation Post-processor | Sojung An (Korea Institute of Atmosphere Prediction Systems); Junha Lee (Korea Institute of Industrial Technology); Jiyeon Jang (Korea Institute of Atmosphere Prediction Systems); Inchae Na (Korea Institute of Atmosphere Prediction Systems); Wooyeon Park (Korea Institute of Atmosphere Prediction Systems); Sujeong You (KITECH) |
(19) Enhancing Data Center Sustainability with a 3D CNN-Based CFD Surrogate Model | Soumyendu Sarkar (Hewlett Packard Enterprise); Antonio Guillen (Hewlett Packard Enterprise); Zachariah Carmichael (University of Notre Dame); Vineet Gundecha (Hewlett Packard Enterpise); Avisek Naug (Hewlett Packard Enterprise); Ashwin Ramesh Babu (Hewlett Packard Enterprise Labs); Ricardo Luna Gutierrez (Hewlett Packard Enterprise) |
(20) Price-Aware Deep Learning for Electricity Markets | Vladimir Dvorkin (Massachusetts Institute of Technology); Ferdinando Fioretto (University of Virginia) |
(21) A machine learning framework for correcting under-resolved simulations of turbulent systems using nudged datasets | Benedikt Barthel (MIT); Themis Sapsis (MIT) |
(22) Can Deep Learning help to forecast deforestation in the Amazonian Rainforest? | Tim Engelmann (ETH Zurich); Malte Toetzke (ETH Zurich) |
(23) Spatially-resolved emulation of climate extremes via machine learning stochastic models | Mengze Wang (Massachusetts Institute of Technology); Andre Souza (Massachusetts Institute of Technology); Raffaele Ferrari (Massachusetts Institute of Technology); Themis Sapsis (MIT) |
(24) Reinforcement Learning control for Airborne Wind Energy production | Lorenzo Basile (University of Trieste); Maria Grazia Berni (University of Trieste); Antonio Celani (ICTP) |
(25) Extreme Event Prediction with Multi-agent Reinforcement Learning-based Parametrization of Atmospheric and Oceanic Turbulence | Rambod Mojgani (Rice University); Daniel Waelchli (ETHZ); Yifei Guan (Rice University); Petros Koumoutsakos (Harvard); Pedram Hassanzadeh (Rice University) |
(26) Machine learning derived sub-seasonal to seasonal extremes | Daniel Salles Civitarese (IBM Research, Brazil); Bianca Zadrozny (IBM Research) |
(27) Understanding Opinions Towards Climate Change on Social Media | Yashaswi Pupneja (University of Montreal); Yuesong Zou (McGill University); Sacha Levy (Yale University); Shenyang Huang (Mila/McGill University) |
(28) Real-time Carbon Footprint Minimization in Sustainable Data Centers wth Reinforcement Learning Best ML Innovation | Soumyendu Sarkar (Hewlett Packard Enterprise); Avisek Naug (Hewlett Packard Enterprise); Ricardo Luna Gutierrez (Hewlett Packard Enterprise); Antonio Guillen (Hewlett Packard Enterprise); Vineet Gundecha (Hewlett Packard Enterpise); Ashwin Ramesh Babu (Hewlett Packard Enterprise Labs); Cullen Bash (HPE) |
(29) Machine learning applications for weather and climate predictions need greater focus on extremes | Peter Watson (Bristol) |
(30) Climate-sensitive Urban Planning through Optimization of Tree Placements | Simon Schrodi (University of Freiburg); Ferdinand Briegel (University of Freiburg); Max J. Argus (University Of Freiburg); Andreas Christen (University of Freiburg); Thomas Brox (University of Freiburg) |
(31) Reinforcement Learning for Wildfire Mitigation in Simulated Disaster Environments | Alexander Tapley (The MITRE Corporation); savanna o smith (MITRE); Tim Welsh (The MITRE Corporation); Aidan Fennelly (The MITRE Corporation); Dhanuj M Gandikota (The MITRE Corporation); Marissa Dotter (MITRE Corporation); Michael Doyle (The MITRE Corporation); Michael Threet (MITRE) |
(32) Combining deep generative models with extreme value theory for synthetic hazard simulation: a multivariate and spatially coherent approach | Alison M Peard (University of Oxford); Jim Hall (University of Oxford) |
(33) Uncertainty Quantified Machine Learning for Street Level Flooding Predictions in Norfolk, Virginia | Steven Goldenberg (Thomas Jefferson National Accelerator Facility); Diana McSpadden (Thomas Jefferson National Accelerator Facility); Binata Roy (University of Virginia); Malachi Schram (Thomas Jefferson National Accelerator Facility); Jonathan Goodall (University of Virginia); Heather Richter (Old Dominion University) |
(34) Weakly-semi-supervised object detection in remotely sensed imagery | Ji Hun Wang (Stanford University); Jeremy Irvin (Stanford University); Beri Kohen Behar (Stanford University); Ha Tran (Stanford University); Raghav Samavedam (Stanford University); Quentin Hsu (Stanford University); Andrew Ng (Stanford University) |
(35) Hybridizing Physics and Neural ODEs for Predicting Plasma Inductance Dynamics in Tokamak Fusion Reactors | Allen Wang (MIT); Cristina Rea (MIT); Darren Garnier (MIT) |
(36) Explainable Offline-online Training of Neural Networks for Multi-scale Climate Modeling | Hamid Alizadeh Pahlavan (Rice University); Pedram Hassanzadeh (Rice University); M. Joan Alexander (NorthWest Research Associates) |
(37) Southern Ocean Dynamics Under Climate Change: New Knowledge Through Physics-Guided Machine Learning | William J Yik (Harvey Mudd College); Maike Sonnewald (University of California, Davis); Mariana Clare (ECMWF); Redouane Lguensat (IPSL) |
(38) Prototype-oriented Unsupervised Change Detection for Disaster Management | YoungTack Oh (SI Analytics); Minseok Seo (si-analytics); Doyi Kim (SI Analytics); Junghoon Seo (SI Analytics) |
(39) How to Recycle: General Vision-Language Model without Task Tuning for Predicting Object Recyclability | Eliot Park (Harvard Medical School); Eddy Pan (Harvard Medical School); Shreya Johri (Harvard Medical School); Pranav Rajpurkar (Harvard Medical School) |
(40) Flowering Onset Detection: Traditional Learning vs. Deep Learning Performance in a Sparse Label Context | Mauricio Soroco (University of British Columbia); Joel Hempel (University of British Columbia); Xinze Xiong (University of British Columbia); Mathias Lécuyer (University of British Columbia); Joséphine Gantois (University of British Columbia) |
(41) Zero shot microclimate prediction with deep learning | Iman Deznabi (UMass); Peeyush Kumar (Microsoft Research); Madalina Fiterau (University of Massachusetts Amherst) |
(42) Glacier Movement Prediction with Attention-based Recurrent Neural Networks and Satellite Data | Jonas Müller (University of Tübingen); Raphael Braun (University of Tübingen); Hendrik P. A. Lensch (University of Tübingen); Nicole Ludwig (University of Tübingen) |
(43) A Causal Discovery Approach To Learn How Urban Form Shapes Sustainable Mobility Across Continents | Felix Wagner (TU Berlin, MCC Berlin); Florian Nachtigall (MCC Berlin); Lukas B Franken (University of Edinburgh); Nikola Milojevic-Dupont (Mercator Research Institute on Global Commons and Climate Change (MCC)); Marta C. González (Berkeley); Jakob Runge (TU Berlin); Rafael Pereira (IPEA); Felix Creutzig (Mercator Research Institute on Global Commons and Climate Change (MCC)) |
(44) Unleashing the Autoconversion Rates Forecasting: Evidential Regression from Satellite Data | Maria C Novitasari (University College London); Johannes Quaas (Universität Leipzig); Miguel Rodrigues (University College London) |
(45) Detailed Glacier Area Change Analysis in the European Alps with Deep Learning | Codrut-Andrei Diaconu (DLR); Jonathan Bamber (Technical University of Munich) |
(46) An LSTM-based Downscaling Framework for Australian Precipitation Projections | Matthias Bittner (Vienna University of Technology); Sanaa Hobeichi (The University of New South Wales); Muhammad Zawish (Walton Institute, WIT); Samo DIATTA (Assane Seck University of Ziguinchor); Remigius Ozioko (University of Nigeria); Sharon Xu (Indigo Ag); Axel Jantsch (TU Wien) |
(47) A machine learning pipeline for automated insect monitoring | Aditya Jain (Mila); Fagner Cunha (Federal University of Amazonas); Michael Bunsen (Mila, eButterfly); Léonard Pasi (EPFL); Anna Viklund (Daresay); Maxim Larrivee (Montreal Insectarium); David Rolnick (McGill University, Mila) |
(48) Climate Variable Downscaling with Conditional Normalizing Flows | Christina Elisabeth Winkler (Mila); Paula Harder (Mila); David Rolnick (McGill University, Mila) |
(49) Global Coastline Evolution Forecasting from Satellite Imagery using Deep Learning | Guillaume RIU (Laboratory of Spatial Geophysics and Oceanography Studies); Mahmoud AL NAJAR (Laboratory of Spatial Geophysics and Oceanography Studies); Gregoire THOUMYRE (Laboratory of Spatial Geophysics and Oceanography Studies); Rafael ALMAR (Laboratory of Spatial Geophysics and Oceanography Studies); Dennis Wilson (ISAE) |
(50) Can Reinforcement Learning support policy makers? A preliminary study with Integrated Assessment Models | Theodore LM Wolf (Carbon Re); Nantas Nardelli (CarbonRe); John Shawe-Taylor (University College London); Maria Perez-Ortiz (University College London) |
(51) IceCloudNet: Cirrus and mixed-phase cloud prediction from SEVIRI input learned from sparse supervision | Kai Jeggle (ETH Zurich); Mikolaj Czerkawski (ESA); Federico Serva (European Space Agency, Italian Space Agency); Bertrand Le Saux (European Space Agency (ESA)); David Neubauer (ETH Zurich); Ulrike Lohmann (ETH Zurich) |
(52) SAM-CD: Change Detection in Remote Sensing Using Segment Anything Model | Faroq ALTam (Elm Company); Thariq Khalid (Elm Company); Athul Mathew (Elm Company); Andrew Carnell (Elm Company); Riad Souissi (Elm Company) |
(53) Segment-then-Classify: Few-shot instance segmentation for environmental remote sensing | Yang Hu (University of California, Santa Barbara); Kelly Caylor (UCSB); Anna S Boser (UCSB) |
(54) Surrogate modeling based History Matching for an Earth system model of intermediate complexity | Maya Janvier (Centrale Supélec); Redouane Lguensat (IPSL); Julie Deshayes (LOCEAN IPSL); Aurélien Quiquet (LSCE); Didier Roche (LSCE); V. Balaji (Schmidt Futures) |
(55) FireSight: Short-Term Fire Hazard Prediction Based on Active Fire Remote Sensing Data | Julia Gottfriedsen (OroraTech GmbH); Johanna Strebl (OroraTech GmbH); Max Berrendorf (Ludwig-Maximilians-Universität München); Martin Langer (OroraTech GmbH); Volker Tresp (Ludwig-Maximilians-Universität München) |
(56) Scaling Sodium-ion Battery Development with NLP | Mrigi Munjal (Massachusetts Institute of Technology); Thorben Pein (TU Munich); Vineeth Venugopal (Massachusetts Institute of Technology); Kevin Huang (Massachusetts Institute of Technology); Elsa Olivetti (Massachusetts Institute of Technology) |
(57) The built environment and induced transport CO2 emissions: A double machine learning approach to account for residential self-selection | Florian Nachtigall (Technical University of Berlin); Felix Wagner (TU Berlin, MCC Berlin); Peter Berrill (Technical University of Berlin); Felix Creutzig (Mercator Research Institute on Global Commons and Climate Change (MCC)) |
(58) Lightweight, Pre-trained Transformers for Remote Sensing Timeseries | Gabriel Tseng (NASA Harvest); Ruben Cartuyvels (KULeuven); Ivan Zvonkov (University of Maryland); Mirali Purohit (Arizona State University (ASU)); David Rolnick (McGill University, Mila); Hannah R Kerner (Arizona State University) |
(59) Top-down Green-ups: Satellite Sensing and Deep Models to Predict Buffelgrass Phenology | Lucas Rosenblatt (NYU); Bin Han (University of Washington); Erin Posthumus (USA NPN); Theresa Crimmins (USA NPN); Bill G Howe (University of Washington) |
(60) Typhoon Intensity Prediction with Vision Transformer | Huanxin Chen (South China University of Technology); Pengshuai Yin (South China University of Technology); Huichou Huang (City University of Hong Kong); Qingyao Wu (South China University of Technology); Ruirui Liu (Brunel University London); Xiatian Zhu (University of Surrey) |
(61) Data Assimilation using ERA5, ASOS, and the U-STN model for Weather Forecasting over the UK | WENQI WANG (Imperial College London); Jacob Bieker (Open Climate Fix); Rossella Arcucci (Imperial College London); Cesar Quilodran-Casas (Imperial College London) |
(62) Stress-testing the coupled behavior of hybrid physics-machine learning climate simulations on an unseen, warmer climate | Jerry Lin (University of California, Irvine); Mohamed Aziz Bhouri (Columbia University); Tom G Beucler (Columbia University & UCI); Sungduk Yu (University of California, Irvine); Michael Pritchard (UCI) |
(63) Deploying Reinforcement Learning based Economizer Optimization at Scale | Ivan Cui (Amazon); Wei Yih Yap (Amazon); Charles Prosper (Independant); Bharathan Balaji (Amazon); Jake Chen (Amazon) |
(64) The Power of Explainability in Forecast-Informed Deep Learning Models for Flood Mitigation | Jimeng Shi (Florida International University); Vitalii Stebliankin (FIU); Giri Narasimhan (Florida International University) |
(65) Surrogate Neural Networks to Estimate Parametric Sensitivity of Ocean Models | Yixuan Sun (Argonne National Laboratory); Elizabeth Cucuzzella (Tufts University); Steven Brus (Argonne National Laboratory); Sri Hari Krishna Narayanan (Argonne National Laboratory); Balu Nadiga (Los Alamos National Lab); Luke Van Roekel (Los Alamos National Laboratory); Jan Hückelheim (Argonne National Laboratory); Sandeep Madireddy (Argonne National Laboratory) |
(66) Towards a spatio-temporal deep learning approach to predict malaria outbreaks using earth observation measurements in South Asia | Usman Nazir (Lahore University of Management Sciences); Ahzam Ejaz (Lahore University of Management Sciences); Muhammad Talha Quddoos (Lahore University of Management Sciences); Momin Uppal (Lahore University of Management Sciences); Sara khalid (University of Oxford) |
(67) Contextual Reinforcement Learning for Offshore Wind Farm Bidding | David Cole (University of Wisconsin-Madison); Himanshu Sharma (Pacific Northwest National Laboratory); Wei Wang (Pacific Northwest National Laboratory) |
(68) CityTFT: Temporal Fusion Transformer for Urban Building Energy Modeling | Ting-Yu Dai (The University of Texas at Austin); Dev Niyogi (The University of Texas at Austin); Zoltan Nagy (The University of Texas at Austin) |
(69) A Scalable Network-Aware Multi-Agent Reinforcement Learning Framework for Distributed Converter-based Microgrid Voltage Control | Han Xu (Tsinghua University); Guannan Qu (Carnegie Mellon University) |
(70) Reinforcement Learning in agent-based modeling to reduce carbon emissions in transportation | Yuhao Yuan (UC Berkeley); Felipe Leno da Silva (Lawrence Livermore National Laboratory); Ruben Glatt (Lawrence Livermore National Laboratory) |
(71) Continuous Convolutional Neural Networks for Disruption Prediction in Nuclear Fusion Plasmas | William F Arnold (KAIST); Lucas Spangher (MIT PSFC); Cristina Rea (MIT PSFC) |
(72) Comparing Data-Driven and Mechanistic Models for Predicting Phenology in Deciduous Broadleaf Forests | Christian Reimers (Department of Biogeochemical Integration, Max Planck Institute for Biogeochemistry); David Hafezi Rachti (Department of Biogeochemical Integration, Max Planck Institute for Biogeochemistry); Guohua Liu (Department of Biogeochemical Integration, Max Planck Institute for Biogeochemistry); Alexander Winkler (Department of Biogeochemical Integration, Max Planck Institute for Biogeochemistry) |
(73) RMM-VAE: a machine learning method for identifying probabilistic weather regimes targeted to a local-scale impact variable | Fiona R Spuler (University of Reading); Marlene Kretschmer (Universität Leipzig); Yevgeniya Kovalchuck (University College London); Magdalena Balmaseda (ECMWF); Ted Shepherd (University of Reading) |
(74) Accelerating GHG Emissions Inference: A Lagrangian Particle Dispersion Model Emulator Using Graph Neural Networks | Elena Fillola (University of Bristol); Raul Santos Rodriguez (University of Bristol); Matt Rigby (University of Bristol) |
(75) Gaussian Processes for Monitoring Air-Quality in Kampala | Clara Stoddart (Imperial College London); Lauren Shrack (Massachusetts Institute of Technology); Usman Abdul-Ganiy (AirQo, Makerere University); Richard Sserunjogi (AirQo, Makerere University); Engineer Bainomugisha (AirQo, Makerere University); Deo Okure (AirQo, Makerere University); Ruth Misener (Imperial College London); Jose Pablo Folch (Imperial College London); Ruby Sedgwick (Imperial College London) |
(76) Large Scale Masked Autoencoding for Reducing Label Requirements on SAR Data Overall Best Paper | Matthew J Allen (University of Cambridge); Francisco Dorr (Independent); Joseph A Gallego (National University Of Colombia); Laura Martínez-Ferrer (University of Valencia); Freddie Kalaitzis (University of Oxford); Raul Ramos-Pollan (Universidad de Antioquia); Anna Jungbluth (European Space Agency) |
(77) Sim2Real for Environmental Neural Processes | Jonas Scholz (University of Cambridge) |
(78) Methane Plume Detection with U-Net Segmentation on Sentinel-2 Image Data | Berenice du Baret (ISAE-Supaero); Simon Finos (ISAE-Supaero); Hugo Guiglion (ISAE-Supaero); Dennis Wilson (ISAE) |
(79) Machine Learning Assisted Bayesian Calibration of Model Physics Parameters for Wetland Methane Emissions: A Case Study at a FLUXNET-CH4 Site | Sandeep Chinta (Massachusetts Institute of Technology); Xiang Gao (Massachusetts Institute of Technology); Qing Zhu (Lawrence Berkeley National Laboratory) |
(80) ALAS: Active Learning for Autoconversion Rates Prediction from Satellite Data | Maria C Novitasari (University College London); Johanness Quaas (Universität Leipzig); Miguel Rodrigues (University College London) |
(81) Towards Causal Representations of Climate Model Data | Julien Boussard (Columbia University); Chandni Nagda (University of Illinois at Urbana-Champaign); Julia Kaltenborn (McGill University); Charlotte Lange (Mila); Yaniv Gurwicz (Intel Labs); Peer Nowack (Grantham Institute, Imperial College London. Department of Physics, Imperial College. Data Science Institute, Imperial College. School of Environmental Sciences, University of East Anglia); David Rolnick (McGill University, Mila) |
(82) ClimateX: Do LLMs Accurately Assess Human Expert Confidence in Climate Statements? | Romain Lacombe (Stanford University); Kerrie Wu (Stanford University); Eddie Dilworth (Stanford University) |
(83) Improving Flood Insights: Diffusion-based SAR to EO Image Translation | Minseok Seo (si-analytics); YoungTack Oh (SI Analytics); Doyi Kim (SI Analytics); Dongmin Kang (SIA); Yeji Choi (SI Analytics) |
(84) A Wildfire Vulnerability Index for Businesses Using Machine Learning Approaches | Andrew Byrnes (Dun and Bradstreet); Lisa Stites (Dun and Bradstreet) |
(85) Exploring Causal Relationship between Environment and Drizzle Properties using Machine Learning | Piyush Garg (Argonne National Laboratory); Virendra Ghate (Argonne National Laboratory); Maria Cadeddu (Argonne National Laboratory); Bethany Lusch (Argonne National Lab) |
(86) Asset Bundling for Wind Power Forecasting | Hanyu Zhang (Georgia Institute of Technology); Mathieu Tanneau (Georgia Institute of Technology); Chaofan Huang (Georgia Institute of Technology); Roshan Joseph (Georgia Institute of Technology); Shangkun Wang (Georgia Institute of Technology); Pascal Van Hentenryck (Georgia Institute of Technology) |
(87) Soil Organic Carbon Estimation from Climate-related Features with Graph Neural Network | Weiying Zhao (Deep Planet); Natalia Efremova (Queen Mary University London) |
(88) Deep Glacier Image Velocimetry: Mapping glacier velocities from Sentinel-2 imagery with deep learning | James B Tlhomole (Imperial College London); Matthew Piggott (Imperial College London); Graham Hughes (Imperial College London) |
(89) DeepEn2023: Energy Datasets for Edge Artificial Intelligence | XIAOLONG TU (Georgia State University); Anik Mallik (University of North Carolina at Charlotte); Jiang Xie (University of North Carolina at Charlotte); Haoxin Wang (Georgia State University) |
(90) Interpretable machine learning approach to understand U.S. prevented planting events and project climate change impacts | Haynes Stephens (University of Chicago) |
(91) GraphTransformers for Geospatial Forecasting of Hurricane Trajectories | Satyaki Chakraborty (Carnegie Mellon University); Pallavi Banerjee (University of Washington) |
(92) Integrating Building Survey Data with Geospatial Data: A Cluster-Based Ethical Approach | Vidisha Chowdhury (University of Pennsylvania); Gabriela Gongora-Svartzman (Carnegie Mellon University); Erin D Trochim (University of Alaska Fairbanks); Philippe Schicker (Carnegie Mellon University) |
(93) Breeding Programs Optimization with Reinforcement Learning | Omar G. Younis (ETH Zurich); Luca Corinzia (ETH Zurich - Information Science & Engineering Group); Ioannis N Athanasiadis (Wageningen University and Research); Andreas Krause (ETH Zürich); Joachim Buhmann (ETH Zurich); Matteo Turchetta (ETH Zurich) |
(94) Discovering Effective Policies for Land-Use Planning Best Pathway to Impact | Risto Miikkulainen (UT Austin; Cognizant Technology Solutions); Olivier Francon (Cognizant AI Labs); Daniel Young (Cognizant AI Labs); Babak Hodjat (Cognizant AI Labs); Hugo Cunha (Cognizant AI Labs); Jacob Bieker (Open Climate Fix) |
(95) Probabilistic land cover modeling via deep autoregressive models | Christopher Krapu (Duke University); Ryan Calder (Virginia Tech); Mark Borsuk (Duke University) |
(96) Simulating the Air Quality Impact of Prescribed Fires Using a Graph Neural Network-Based PM2.5 Emissions Forecasting System | Kyleen Liao (Saratoga High School); Jatan Buch (Columbia University); Kara D. Lamb (Columbia University); Pierre Gentine (Columbia University) |
(97) Hyperspectral shadow removal with iterative logistic regression and latent Parametric Linear Combination of Gaussians | Core Francisco Park (Harvard University); Maya Nasr (Harvard University); Manuel Pérez-Carrasco (University of Concepcion); Eleanor Walker (Harvard University); Douglas Finkbeiner (Harvard University); Cecilia Garraffo (AstroAI at the Center for Astrophysics, Harvard & Smitnsonian) |
(98) Cooperative Logistics: Can Artificial Intelligence Enable Trustworthy Cooperation at Scale? | Stephen Mak (University of Cambridge); Tim Pearce (Microsoft Research); Matthew Macfarlane (University of Amsterdam); Liming Xu (University of Cambridge); Michael Ostroumov (Value Chain Lab); Alexandra Brintrup (University of Cambridge) |
(99) Resource Efficient and Generalizable Representation Learning of High-Dimensional Weather and Climate Data | Juan Nathaniel (Columbia University); Marcus Freitag (IBM); Patrick Curran (Environment and Climate Change Canada); Isabel Ruddick (Environment and Climate Change Canada); Johannes Schmude (IBM) |
(100) Difference Learning for Air Quality Forecasting Transport Emulation | Reed R Chen (Johns Hopkins University Applied Physics Laboratory); Christopher Ribaudo (Johns Hopkins University Applied Physics Laboratory); Jennifer Sleeman (University of Maryland, Baltimore County and Johns Hopkins University Applied Physics Laboratory); Chace Ashcraft (JHU/APL); Marisa Hughes (JHU) |
(101) Fusion of Physics-Based Wildfire Spread Models with Satellite Data using Generative Algorithms | Bryan Shaddy (University of Southern California); Deep Ray (University of Maryland); Angel Farguell (San Jose State University); Valentina Calaza (University of Southern California); Jan Mandel (University of Colorado Denver); James Haley (Cooperative Institute for Research in the Atmosphere); Kyle Hilburn (Cooperative Institute for Research in the Atmosphere); Derek Mallia (University of Utah); Adam Kochanski (San Jose State University); Assad Oberai (University of Southern California) |
(102) A 3D super-resolution of wind fields via physics-informed pixel-wise self-attention generative adversarial network | Takuya Kurihana (University of Chicago); Levente Klein (IBM Research); Kyongmin Yeo (IBM Research); Daniela Szwarcman (IBM Research); Bruce G Elmegreen (IBM Research); Surya Karthik Mukkavilli (IBM Research, Zurich); Johannes Schmude (IBM) |
(103) Proof-of-concept: Using ChatGPT to Translate and Modernize an Earth System Model from Fortran to Python/JAX | Anthony Zhou (Columbia University), Linnia Hawkins (Columbia University), Pierre Gentine (Columbia University) |
(104) Learning to forecast diagnostic parameters using pre-trained weather embedding | Peetak Mitra (Excarta); Vivek Ramavajjala (Excarta) |
(105) PressureML: Modelling Pressure Waves to Generate Large-Scale Water-Usage Insights in Buildings | Tanmaey Gupta (Microsoft Research India); Anupam Sobti (IIT Delhi); Akshay Nambi (Microsoft Research) |
(106) Facilitating Battery Swapping Services for Freight Trucks with Spatial-Temporal Demand Prediction | Linyu Liu (Tsinghua University); Zhen Dai (Chongqing Expressway Group Company); Shiji Song (Department of Automation, Tsinghua University); Xiaocheng Li (Imperial College London); Guanting Chen (The University of North Carolina at Chapel Hill) |
(107) Domain Adaptation for Sustainable Soil Management using Causal and Contrastive Constraint Minimization | Somya Sharma (U. Minnesota); Swati Sharma (Microsoft Research); RAFAEL PADILHA (Microsoft Research); Emre Kiciman (Microsoft Research); Ranveer Chandra (Microsoft Research) |
(108) Inference of CO2 flow patterns--a feasibility study | Abhinav Prakash Gahlot (Georgia Institute of Technology); Huseyin Tuna Erdinc (Georgia Institute of Technology); Rafael Orozco (Georgia Institute of Technology); Ziyi Yin (Georgia Institute of Technology); Felix Herrmann (Georgia Institute of Technology) |
(109) Generative Nowcasting of Marine Fog Visibility in the Grand Banks area and Sable Island in Canada | Eren Gultepe (Southern Illinois University Edwardsville); Sen Wang (University of Notre Dame); Byron Blomquist (NOAA); Harindra Fernando (University of Notre Dame); Patrick Kreidl (University of North Florida); David Delene (University of North Dakota); Ismail Gultepe (Ontario Tech University) |
(110) Data-Driven Traffic Reconstruction and Kernel Methods for Identifying Stop-and-Go Congestion | Edgar Ramirez Sanchez (MIT); Shreyaa Raghavan (MIT); Cathy Wu () |
(111) AI assisted Search for Atmospheric CO2 Capture | Shivashankar Shivashankar (Student) |
(112) Towards Recommendations for Value Sensitive Sustainable Consumption | Thomas Asikis (University of Zurich) |
(113) Towards autonomous large-scale monitoring the health of urban trees using mobile sensing | Akshit Gupta (Delft University of Technology); Martine Rutten (Delft University of Technology); RANGA RAO VENKATESHA PRASAD (TUDelft); Remko Uijlenhoet (Delft University of Technology) |
(114) Towards Global, General-Purpose Pretrained Geographic Location Encoders | Konstantin Klemmer (Microsoft Research); ESTHER ROLF (Google Research); Caleb Robinson (Microsoft AI for Good Research Lab); Lester Mackey (Microsoft Research); Marc Rußwurm (École Polytechnique Fédérale de Lausanne) |
(115) Spatially Regularized Graph Attention Autoencoder Framework for Detecting Rainfall Extremes | Mihir Agarwal (IIT Gandhinagar); Progyan Das (IIT Gandhinagar); Udit Bhatia (IIT Gandhinagar) |
(116) Antarctic Bed Topography Super-Resolution via Transfer Learning | Kim Bente (The University of Sydney); Roman Marchant (University of Technology Sydney); Fabio Ramos (NVIDIA, The University of Sydney) |
(117) Elucidating the Relationship Between Climate Change and Poverty using Graph Neural Networks, Ensemble Models, and Remote Sensing Data | Parinthapat Pengpun (Bangkok Christian International School); Alessandro Salatiello (University of Tuebingen) |
(118) Sustainability AI copilot: Analyze & ideate at scale to enable positive impact | Rajagopal A (Indian Institute of Technology); Nirmala V (Queen Marys); Immanuel Raja (Karunya University); Arun V (NIT) |
Proposals
Title | Authors |
---|---|
(119) Causality and Explainability for Trustworthy Integrated Pest Management | Ilias Tsoumas (National Observatory of Athens); Vasileios Sitokonstantinou (University of Valencia); Georgios Giannarakis (National Observatory of Athens); Evagelia Lampiri (University of Thessaly); Christos Athanassiou (University of Thessaly); Gustau Camps-Valls (Universitat de València); Charalampos Kontoes (National Observatory of Athens); Ioannis N Athanasiadis (Wageningen University and Research) |
(120) Monitoring Sustainable Global Development Along Shared Socioeconomic Pathways | Michelle Wan (University of Cambridge); Jeff Clark (University of Bristol); Edward Small (Royal Melbourne Institute of Technology); Elena Fillola (University of Bristol); Raul Santos Rodriguez (University of Bristol) |
(121) Understanding Climate Legislation Decisions with Machine Learning | Jeff Clark (University of Bristol); Michelle Wan (University of Cambridge); Raul Santos Rodriguez (University of Bristol) |
(122) Mapping the Landscape of Artificial Intelligence in Climate Change Research: A Meta-Analysis on Impact and Applications | Christian Burmester (Osnabrück University); Teresa Scantamburlo (UniversityofVenice) |
(123) Unlocking the Potential of Renewable Energy Through Curtailment Prediction | Bilge Acun (Meta / FAIR); Brent Morgan (Meta); Henry Richardson (WattTime); Nat Steinsultz (WattTime); Carole-Jean Wu (Meta / FAIR) |
(124) Assessing data limitations in ML-based LCLU | Angel Encalada-Davila (ESPOL); Christian Tutiven (ESPOL University); Jose E Cordova-Garcia (ESPOL) |
(125) Predicting Adsorption Energies for Catalyst Screening with Transfer Learning Using Crystal Hamiltonian Graph Neural Network | Angelina Chen (Foothill College/Lawrence Berkeley National Lab); Hui Zheng (Lawrence Berkeley National Lab); Paula Harder (Mila) |
(126) Physics-informed DeepONet for battery state prediction | Keyan Guo (Peking University) |
(127) Decarbonizing Maritime Operations: A Data-Driven Revolution | Ismail Bourzak (UQAR); Loubna Benabou (UQAR); Sara El Mekkaoui (DNV); Abdelaziz Berrado (EMI Engineering School) |
(128) High-resolution Global Building Emissions Estimation using Satellite Imagery | Paul J Markakis (Duke University); Jordan Malof (University of Montana); Trey Gowdy (Duke University); Leslie Collins (Duke University); Aaron Davitt (WattTime); Gabriela Volpato (WattTime); Kyle Bradbury (Duke University) |
(129) Sand Mining Watch: Leveraging Earth Observation Foundation Models to Inform Sustainable Development | Ando Shah (UC Berkeley); Suraj R Nair (UC Berkeley); Tom Boehnel (TU Munich); Joshua Blumenstock (University of California, Berkeley) |
(130) Understanding Insect Range Shifts with Out-of-Distribution Detection | Yuyan Chen (McGill University, Mila); David Rolnick (McGill University, Mila) |
Tutorials
Title | Authors |
---|---|
(131) Aquaculture Mapping: Detecting and Classifying Aquaculture Ponds using Deep Learning | John Christian G Nacpil (Thinking Machines Data Science, Inc.); Joshua Cortez (Thinking Machines Data Science) |
(132) Zero-Emission Vehicle Intelligence (ZEVi): Effectively Charging Electric Vehicles at Scale Without Breaking Power Systems (or the Bank) | Shasha Lin (NextEra Mobility); Jonathan Brophy (NextEra Mobility); Tamara Monge (NextEra Mobility); Jamie Hussman (NextEra Mobility); Michelle Lee (NextEra Mobility); Sam Penrose (NextEra Mobility) |
(133) Agile Modeling for Bioacoustic Monitoring | tom denton (google); Jenny Hamer (Google Research); Rob Laber (Google) |
Informational Webinar
We recently conducted two informational webinars answering questions about the mentorship program and how to prepare a successful submission for the workshop. The video recording of the Aug 16 webinar can be found here. –>
Organizers
Rasika Bhalerao (Northeastern University)
Mark Roth (Climate LLC)
Kai Jeggle (ETH Zurich)
Jorge Montalvo (Centrica)
Shiva Madadkhani (Technical University of Munich)
Yoshua Bengio (Mila, UdeM, CIFAR, IVADO)
Tutorials Track Organizers
Isabelle Tingzon (The World Bank/GFDRR)
Melanie Hanna (DataRobot)
Nadia Ahmed (UC Irvine)
Shafat Rahman (Otoll)
Mentors
Akshata Kishore Moharir (Boeing)
Alessandro Salatiello (Amazon)
Ankur Narang (VAB)
Benjamin Huynh (Johns Hopkins University)
Beril Kallfelz (Yeditepe Üniversitesi)
Bertrand Le Saux (ESA)
Bharathan Balaji (Amazon)
Elie Alhajjar (RAND)
Ferdinando Fioretto (Univeristy of Virginia)
Gideon Dresdner (Allen AI Institute)
Lekha Patel (Sandia National Laboratories)
Lisa Tang (Simon Fraser University)
Lucas Spangher (MIT Plasma Science and Fusion Center)
Maryam Rahnemoonfar (Lehigh University)
Michael Smith (Aspia Space)
Nikolaos Dionelis (ESA)
Paula Harder (McGill University, Mila)
Peter Jack Naylor (ESA)
Quentin Paletta (ESA)
Reshmi Ghosh (Microsoft Research)
Salva Ruhling Cachay (UC San Diego)
Seyed Masoud Mohseni-Bonab (Hydro-Quebec Research Institute)
Tejasri Nampally (Indian Institute of Technology Hyderabad)
Ying-Jung C. Deweese (Descartes Labs / Georgia Insititute of Technology)
Program Committee
Adithya Ramachandran (Pattern Recognition Lab, Friedrich Alexander University, Erlangen)
Adwaita J. Jadhav (Apple)
Akanksha Ahuja (NOA)
Akshat Bhandari (Manipal Institute of Technology, Manipal)
Alberto Carpentieri (Bern University of Applied Science)
Alexander Pondaven (University of Oxford)
Alexandra V. Puchko (Kettle RE)
Alona Zharova (Humboldt University of Berlin)
Amirmohammad Naeini (York University)
Andreas K. Maier (Pattern Recognition Lab, FAU Erlangen-Nuremberg)
Andrew Ross (Harvard University)
Anna Kwa (Allen Institute for Artificial Intelligence)
Annika Stechemesser (Potsdam Insitute for Climate Impact Research)
Antoine Blanchard (Verisk)
Aranildo Lima (Environment and Climate Change Canada (ECCC))
Arlei Silva (Rice University)
Arvind T. Mohan (Los Alamos National Laboratory)
Ashwin Ramesh Babu (Hewlett Packard Enterprise Labs)
Babak Jalalzadeh Fard (University of Nebraska Medical Center)
Bartosz Pieliński (University of Warsaw)
Bedartha Goswami (University of Tübingen)
Biswadip Dey (Siemens Corporation, Technology)
Brian Hutchinson (Western Washington University)
Carla Roesch (University of Edinburgh)
Chace Ashcraft (JHU/APL)
Charles Anderson (Colorado State University)
Christian Lessig (Otto-von-Guericke-Universitat Magdeburg)
Christopher Yeh (California Institute of Technology)
Claire Robin (Biogeochemical Integration, Max-Planck-Institute for Biogeochemistry, Jena, Germany)
Dan Lu (Oak Ridge National Laboratory)
Daniel Salles Civitarese (IBM Research, Brazil)
David Rolnick (McGill University, Mila)
David Russell (University of California, Davis)
Deval Pandya (Shell Global solutions)
Diana Windemuth (Climate LLC)
Difan Zhang (PNNL)
Donghu Guo (Imperial College London)
Donna Vakalis (Universite de Montreal, Mila)
Doron Hazan (MIT)
Elham Kheradmand (University of Montreal)
Erin D. Trochim (University of Alaska Fairbanks)
Frank Liu (Old Dominion University)
Fred Otieno (IBM)
Gina M. Wong (Climate Change for AI)
Hao Sheng (Stanford University)
Hao Wang (Monash University)
Hari Prasanna Das (UC Berkeley)
Henry Addison (University of Bristol)
Hovig Bayandorian
Ilias Tsoumas (National Observatory of Athens)
Ioana Colfescu (National Centre for Atmospheric Science)
Ioannis N. Athanasiadis (Wageningen University and Research)
Ioannis Papoutsis (National Observatory of Athens)
Isabelle Tingzon (The World Bank/GFDRR)
Jade Guisiano (Climate Change AI)
James Duncan (University of California, Berkeley)
Jan Drgona (Pacific Northwest National Laboratory)
Jinyoung Park (KAIST)
Jishi Zhang (Lawerence Livermore National Laboratory)
Johannes Exenberger (Graz University of Technology)
Jonathan Fürst (ZHAW Zurich University of Applied Sciences)
Jorge Montalvo Arvizu (Solario)
Joris Guerin (LAAS-CNRS)
Joseph Early (University of Southampton)
Julian de Hoog (The University of Melbourne)
Julien Lenhardt (Leipzig University)
Kai Jeggle (ETH Zurich)
Kai-Hendrik Cohrs (University of Valencia)
Kaiser Olga (University)
Kameswari Devi Ayyagari (Dalhousie University)
Ken C. L. Wong (IBM Research – Almaden Research Center)
Kevin McCloskey (Google)
Konstantin Klemmer (Microsoft Research)
Kritika A. Gadpayle (CSTEP)
Kyle Bradbury (Duke University)
Kyongsik Yun (California Institute of Technology)
Leslie Hwang (Arizona State University)
Lucas Pereira (Interactive Technologies Institute, LARSyS, Técnico Lisboa)
Lucas Spangher (MIT PSFC)
Luis A. Fuentes (CIDE)
Madelyn Gaumer (University of Washington)
Mahdi Torabi Rad (Beyond Limits)
Maitreyee Marathe (University of Wisconsin-Madison)
Manmeet Singh (The University of Texas at Austin)
Marc Rußwurm (Wageningen University)
Marcus Voss (TU Berlin)
Maria João Sousa (Cornell Tech, Cornell University)
Maria S. Astefanoaei (IT University of Copenhagen)
Marie C. McGraw (Cooperative Institute for Research in the Atmosphere | CIRA)
Marius Wiggert (UC Berkeley)
Mark Roth (Climate LLC)
Markus Zechner (Stanford University)
Massimo A. Bollasina (University of Edinburgh)
Matias Quintana (National University of Singapore)
Matthias Hertel (KIT)
Maxim Khomiakov (Technical University of Denmark)
Mengxue Zhang (Universitat de València)
Milan Jain (PNNL)
Mohamed Elhabashy (MIT)
Mohit Anand (Helmholtz Centre for Environmental Research - UFZ)
Muhammad F. Kasim (University of Oxford)
Nadia Ahmed (UC Irvine)
Nahian Ahmed (Oregon State University)
Niccolo Dalmasso (J.P. Morgan Chase)
Nicolas Collignon (University of Edinburgh)
Nicole Ludwig (University of Tübingen)
Nidhin Harilal (University of Colorado, Boulder)
Noman Bashir (University of Massachusetts Amherst)
Norhan M. Bayomi (MIT Environmental Solutions Initiative)
Olalekan J. Akintande (University of Ibadan)
Olivia Johnson (Climate Change AI)
Olivia Mendivil Ramos (Climate Change AI)
Panagiotis Moutis (Carnegie Mellon University)
Patrick Lestrange (Climate LLC)
Paul T. Griffiths (National Centre for Atmospheric Science, Cambridge University)
Peer Nowack (Karlsruhe Institute of Technology)
Peetak P. Mitra (Excarta)
Priya Donti (MIT)
Qiao Kang (Memorial University)
Rambod Mojgani (Rice University)
Rasika V. Bhalerao (Northeastern University)
Redouane Lguensat (IPSL)
Reinel Sospedra-Alfonso (Environment and Climate Change Canada)
Reshmi Ghosh (Microsoft Corp.)
Rishikesh Ranade (Ansys Inc)
Rodrigo Rene Rai Munoz Abujder (Johns Hopkins Applied Physics Laboratory)
Ruben Cartuyvels (KU Leuven)
Ryan J. Compton (Climate LLC)
Sam J. Silva (The University of Southern California)
Samarth Vadia (LMU Munich)
Sambhav S. Rohatgi (Spacesense.ai)
Sara El Mekkaoui (DNV)
Sasha Luccioni (Hugging Face)
Satish Kumar (University of California, Santa Barbara)
Seb Hickman (University of Cambridge)
Sebastian Ruf (Northeastern University)
Shafat Rahman (Otoll)
Shahine Bouabid (University of Oxford)
Sharon Xu (Indigo Ag)
Shashank Subramanian (Lawrence Berkeley National Laboratory)
Shiheng Duan (Lawrence Livermore National Laboratory)
Shikhar Nilabh (Amphos21)
Shiva Madadkhani (Technical University of Munich)
Shruti Kulkarni (Indian Institute of Science (IISc))
Shuang Yu (Lawrence Livermore National Laboratory)
Shuyang Xiang (Square Sense)
Silke K. Kaiser (Hertie School)
Siming Bayer (Pattern Recognition Lab, Friedrich-Alexander University)
Somya Sharma (U. Minnesota)
Soumyendu Sarkar (Hewlett Packard Enterprise)
Sriya Rallabandi (Manipal Institute of Technology)
Stefan Feuerriegel (LMU Munich)
Swati Sharma (Microsoft Research)
Tanveer Syeda-Mahmood (IBM Research)
Tao Ge (Washington University in St. Louis)
Tariq Shahzad (University of Johannesburg)
Themis Sapsis (MIT)
Tianle Yuan (NASA)
Tristan Ballard (Sust Inc)
Utkarsha Agwan (U.C. Berkeley)
Victoria Preston (Northeastern University)
Vili Hätönen (Emblica)
Vindula Jayawardana (MIT)
Vineet Jagadeesan Nair (MIT)
Vishal V. Batchu (Google)
Vitus Benson (Max-Planck-Institute for Biogeochemistry)
Xiaojuan Liu (Climate Change AI)
Yanru Zhang (University of Electronic Science and Technology of China)
Yimeng Min (Cornell University)
Ying-Jung C. Deweese (Descartes Labs / Georgia Insititute of Technology)
Yizheng Wang (Stanford University)
Zhecheng Wang (Stanford University)
Zikri Bayraktar (Schlumberger Doll Research)
Ziyang Jiang (Duke University)
Zoltan Nagy (The University of Texas at Austin)
Call for Submissions
We invite submissions of short papers using machine learning to address problems in climate mitigation, adaptation, or modeling, including but not limited to the following topics:
- Agriculture and food
- Behavioural and social science
- Buildings
- Carbon capture and sequestration
- Cities and urban planning
- Climate finance and economics
- Climate justice
- Climate science and climate modeling
- Disaster management and relief
- Earth observations and monitoring
- Earth science
- Ecosystems and biodiversity
- Extreme weather
- Forestry and other land use
- Health
- Heavy industry and manufacturing
- Local and indigenous knowledge systems
- Materials science and discovery
- Oceans and marine systems
- Power and energy systems
- Public policy
- Societal adaptation and resilience
- Supply chains
- Transportation
All machine learning techniques are welcome, from kernel methods to deep learning. Each submission should make clear why the application has (or could have) a pathway to positive impacts regarding climate change. We highly encourage submissions which make their data publicly available. Accepted submissions will be invited to give poster presentations, of which some will be selected for spotlight talks.
The workshop does not publish proceedings, and submissions are non-archival. Submission to this workshop does not preclude future publication. Previously published work may be submitted under certain circumstances (see the FAQ).
All papers and proposals submissions must be through the submission website. Submissions will be reviewed double-blind; do your best to anonymize your submission, and do not include identifying information for authors in the PDF. Authors are required to use the workshop style template (based on the NeurIPS style files), available for LaTeX and docx format.
All tutorials submissions must be through the submission website.
Please see the Tips for Submissions and FAQ, and contact climatechangeai.neurips2023@gmail.com with questions.
Submission Tracks
There are three tracks for submissions: (i) Papers, (ii) Proposals, (iii) Tutorials. Submissions are limited to 4 pages for the Papers track, and 3 pages for the Proposals track, in PDF format (see examples from past workshops). References do not count towards this total. Supplementary appendices are allowed but will be read at the discretion of the reviewers. All submissions must explain why the proposed work has (or could have) positive impacts regarding climate change.
PAPERS Track
Work that is in progress, published, and/or deployed.
Submissions for the Papers track should describe projects relevant to climate change that involve machine learning. These may include (but are not limited to) academic research; deployed results from startups, industry, public institutions, etc.; and climate-relevant datasets.
Submissions should provide experimental or theoretical validation of the method presented, as well as specifying what gap the method fills. Authors should clearly illustrate a pathway to climate impact, i.e., identify the way in which this work fits into broader efforts to address climate change. Algorithms need not be novel from a machine learning perspective if they are applied in a novel setting. Details of methodology need not be revealed if they are proprietary, though transparency is highly encouraged.
Submissions creating novel datasets are welcomed. Datasets should be designed to permit machine learning research (e.g. formatted with clear benchmarks for evaluation). In this case, baseline experimental results on the dataset are preferred, but not required.
PROPOSALS Track
Early-stage work and detailed descriptions of ideas for future work.
Submissions for the Proposals track should describe detailed ideas for how machine learning can be used to solve climate-relevant problems. While less constrained than the Papers track, Proposals will be subject to a very high standard of review. Ideas should be justified as extensively as possible, including motivation for why the problem being solved is important in tackling climate change, discussion of why current methods are inadequate, explanation of the proposed method, and discussion of the pathway to climate impact. Preliminary results are optional.
TUTORIALS Track
Interactive notebooks for insightful step-by-step walkthroughs
Submissions for the Tutorials track should introduce or demonstrate the use of ML methods and tools such as libraries, packages, services, datasets, or frameworks to address a problem related to climate change. Tutorial proposals (due Aug 8) should take the form of an abstract and should include a clear and concise description of users’ expected learning outcomes from the tutorial. Notification of proposal acceptance will be on Aug 18. Midterm tutorial submissions (due Sep 29) and Final tutorial submissions (due Nov 3) should be in the form of executable notebooks (e.g. Jupyter, Colab). Submissions will be reviewed based on their potential impact and overall usability by the climate and AI research community. For more information, check out our tutorial proposal guidelines.
Tips for Submissions
- For examples of typical formatting and content, see submissions from our previous workshops.
- Be explicit: Describe how your proposed approach addresses climate change, demonstrating an understanding of the application area.
- Frame your work: The specific problem and/or data proposed should be contextualized in terms of prior work.
- Address the impact: Describe the practical implications of your method in addressing the problem you identify, as well as any relevant societal impacts or potential side-effects. We recommend reading our further guidelines on this aspect here.
- Explain the ML: Readers may not be familiar with the exact techniques you are using or may desire further detail.
- Justify the ML: Describe why the ML method involved is needed, and why it is a good match for the problem.
- Avoid jargon: Jargon is sometimes unavoidable but should be minimized. Ideal submissions will be accessible both to an ML audience and to experts in other relevant fields, without the need for field-specific knowledge. Feel free to direct readers to accessible overviews or review articles for background, where it is impossible to include context directly.
Addressing Impact
Tackling climate change requires translating ideas into action. The guidelines below will help you clearly present the importance of your work to a broad audience, hopefully including relevant decision-makers in industry, government, nonprofits, and other areas.
- Illustrate the link: Many types of work, from highly theoretical to deeply applied, can have clear pathways to climate impact. Some links may be direct, such as improving solar forecasting to increase utilization within existing electric grids. Others may take several steps to explain, such as improving computer vision techniques for classifying clouds, which could help climate scientists seeking to understand fundamental climate dynamics.
- Consider your target audience: Try to convey with relative specificity why and to whom solving the problem at hand will be useful. If studying extreme weather prediction, consider how you would communicate your key findings to a government disaster response agency. If analyzing a supply chain optimization pilot program, what are the main takeaways for industries who might adopt this technology? To ensure your work will be impactful, where possible we recommend co-developing projects with relevant stakeholders or reaching out to them early in the process for feedback. We encourage you to use this opportunity to do so!
- Outline key metrics: Quantitative or qualitative assessments of how well your results (or for proposals, anticipated results) compare to existing methods are encouraged. Try to give a sense of the importance of your problem and your findings. We encourage you to convey why the particular metrics you choose are relevant from a climate change perspective. For instance, if you are evaluating your machine learning model on the basis of accuracy, how does improved accuracy on a machine learning model translate to climate impact, and why is accuracy the best metric to use in this context?
- Be clear and concise: The discussion of impact does not need to be lengthy, just clear.
- Convey the big picture: Ultimately, the goal of Climate Change AI is to “empower work that meaningfully addresses the climate crisis.” Try to make sure that from the beginning, you contextualize your method and its impacts in terms of this objective.
Mentorship Program
We are hosting a mentorship program to facilitate exchange between potential workshop submitters and experts working in topic areas relevant to the workshop. The goal of this program is to foster cross-disciplinary collaborations and ultimately increase the quality and potential impact of submitted work.
Expectations:
Mentors are expected to guide mentees during the CCAI mentorship program as they prepare submissions for this workshop.
Examples of mentor-mentee interactions may include:
- In-depth discussion of relevant related work in the area of the Paper or Proposal, to ensure submissions are well-framed and contextualized in terms of prior work.
- Iterating on the core idea of a Proposal to ensure that the climate change application is well-posed and the ML techniques used are well-suited.
- Giving feedback on the writing or presentation of a Paper or Proposal to bring it to the right level of maturity for submission.
Mentees are expected to initiate contact with their assigned mentor and put in the work and effort necessary to prepare a Paper or Proposal submission by Sep 29.
We suggest that after the mentor-mentee matching is made, a first (physical or digital) meeting should take place within the first week (Aug 25-31) to discuss the Paper or Proposal and set expectations for the mentorship period. Subsequent interactions can take place either through meetings or via email discussions, following the expectations set during the initial meeting, culminating in a final version of a Paper or Proposal submitted via the CMT portal by Sep 29.
Mentors and mentees must abide by the following Code of Conduct: https://www.climatechange.ai/code_of_conduct.
Application
Applications are due by Aug 18.
- Application to be a mentee: https://forms.gle/ZWBPEkFXP4cc7J1n9
- Application to be a mentor: https://forms.gle/ubkFVLtuwpEFxJ2k8
Sponsors
Frequently Asked Questions
Virtual Attendance FAQ
Q: I’m unsure if I will be able to attend in person, can I still submit a paper or proposal?
A: Yes! Please see the section titled Updates to our Workshop for more details
Q: Is there a separate review process for virtual submissions?
A: No. The review process will be exactly the same. Reviewers will have no knowledge of the author’s attendance status.
Q: Is there a limit to the number of virtual submissions you will accept?
A: No!
Mentorship Program FAQ
Q: Are mentors allowed to be authors on the paper for which they provided mentorship?
A: Yes, mentors can be co-authors but not reviewers.
Q: What happens if the mentor/mentee does not fulfill their duties, or if major issues come up?
A: Please email us at climatechangeai.neurips2023@gmail.com and we will do our best to help resolve the situation. Potential breaches of the Code of Conduct will be responded to promptly as detailed therein.
Q: What happens if I apply to be a mentee but do not get paired with a mentor?
A: While we will do our best, we cannot guarantee pairings for everyone. Even if you do not get paired with a mentor, we encourage you to submit a Paper or Proposal to the workshop, and our reviewers will provide you with guidance and feedback on how to improve it.
Q: What happens if my submission does not get accepted to the workshop?
A: While the mentorship program is meant to give early-career researchers and students the opportunity to improve the quality of their work, sometimes submissions will need further polishing and elaboration before being ready for presentation at a CCAI workshop. If this is the case, we invite you to take into account the comments made by the reviewers and to resubmit again to a subsequent CCAI workshop.
Q: I cannot guarantee that I can commit at least 4 hours to the program over the time period. Should I still apply as a mentor?
A: No. While the 4 hour time commitment is a suggestion, we do believe that it is necessary to ensure that all mentees receive the help and guidance they need.
Q: I do not have a background in machine learning; can I still apply to be a mentor/mentee?
A: Yes! We welcome applications from domains that are complementary to machine learning to solve the problems that we are targeting.
Q: What happens if my mentor/mentee wants to continue meeting after the workshop?
A: We welcome and encourage continued interactions after the official mentorship period. That said, neither the mentor nor the mentee should feel obligated to maintain contact.
Submission FAQ
Q: How can I keep up to date on this kind of stuff?
A: Sign up for our mailing list!
Q: I’m not in machine learning. Can I still submit?
A: Yes, absolutely! We welcome submissions from many fields. Do bear in mind, however, that the majority of attendees of the workshop will have a machine learning background; therefore, other fields should be introduced sufficiently to provide context for the work.
Q: What if my submission is accepted but I can’t attend the workshop?
A: You may ask someone else to present your work in your stead. In exceptional circumstances (e.g., visa issues), on a case-by-case basis, people will be able to send in a recording instead of being there in person.
Q: It’s hard for me to fit my submission on 3 or 4 pages. What should I do?
A: Feel free to include appendices with additional material (these should be part of the same PDF file as the main submission). Do not, however, put essential material in an appendix, as it will be read at the discretion of the reviewers.
Q: Can I send submissions directly by email?
A: No, please use the CMT website to make submissions.
Q: The submission website is asking for my name. Is this a problem for anonymization?
A: You should fill out your name and other info when asked on the submission website; CMT will keep your submission anonymous to reviewers.
Q: Do submissions for the Proposals track need to have experimental validation?
A: No, although some initial experiments or citation of published results would strengthen your submission.
Q: The submission website never sent me a confirmation email. Is this a problem?
A: No, the CMT system does not send automatic confirmation emails after a submission, though the submission should show up on the CMT page once submitted. If in any doubt regarding the submission process, please contact the organizers. Also please avoid making multiple submissions of the same article to CMT.
Q: Can I submit previously published work to this workshop?
A: Yes, though under limited circumstances. In particular, work that has previously been published at non-machine learning venues may be eligible for submission; however, work that has been published in conferences on machine learning or related fields is likely not eligible. If your work was previously accepted to a Climate Change AI workshop, this work should have changed or matured substantively to be eligible for resubmission. Please contact climatechangeai.neurips2023@gmail.com with any questions.
Q: Can I submit work to this workshop if I am also submitting to another NeurIPS 2023 workshop?
A: Yes. We cannot, however, guarantee that you will not be expected to present the material at a time that conflicts with the other workshop.