ICLR 2025 Workshop: Tackling Climate Change with Machine Learning
Livestream
About
Many in the ML community wish to take action on climate change, but are unsure how to have the most impact. This workshop will highlight work that demonstrates that, while ML is no silver bullet, it can be an invaluable tool in reducing greenhouse gas emissions and in helping society adapt to the effects of climate change.
Climate change is a complex problem for which action takes many forms, from advancing theory to deploying new technology. Many of these actions represent high-impact opportunities for real-world change, and simultaneously pose interesting academic research problems.
This workshop is part of a series that aims to bring together those applying ML to climate change challenges and facilitate cross-pollination between ML researchers and experts in climate-relevant fields.
Building on our past workshops on this topic, this edition focuses on two aspects related to data-centric approaches to ML for climate action. Data-centric machine learning is not only a timely topic within the ICLR community, as analyzing and engineering (pre)training datasets becomes increasingly important, but holds specific challenges and opportunities in climate-related areas, for example in smart grid management or water engineering. The goal of our workshop is to connect attendees from different areas at the intersection of climate change and ML, allowing them to share their experiences and learn from one another. The main workshop will take place on April 28, 2025.
About ICLR
This workshop is part of the International Conference on Learning Representations (ICLR), one of the premier conferences on machine learning, which will be held in Singapore between April 24 and 28, 2025. For information on how to attend the ICLR conference, please see here https://iclr.cc/Conferences/2025.
Important Dates
-
Dec 27, 2024: Mentorship program (via application form for mentors and mentees) -
Feb 3, 2025 (23:59, AoE): Paper and proposal submissions -
Mar 5, 2025: Decision notification - Apr 28, 2025: Workshop date
About the Workshop
The workshop will take place on April 28 in Hall 4 #3, Singapore EXPO. Please find the preliminary schedule below.
Schedule
Time (Workshop) | Time (Local) | Event |
---|---|---|
Opening Remarks
Details: (click to expand)Welcome and opening remarks |
||
Keynote Talk - Dr. Iadine Chades
Details: (click to expand)Title: Lessons from Developing Decision AI for NatureAbstract: Climate change is one of the most pressing challenges of our time, but solving it in isolation will not secure a liveable future. Biodiversity loss is deeply entwined with climate disruption, both in cause and consequence. Biodiverse ecosystems regulate the climate, buffer extreme events, and underpin resilience. Biodiversity is a foundational element of climate solutions, not a secondary concern. Restoring and protecting nature offers the most cost-effective way to remove carbon from the atmosphere. Biodiversity is also critical to our global economy: over US$44 trillion in economic value (more than half the world’s GDP) is moderately or highly dependent on nature and its services. In this talk I will share my experience developing AI tools to support decision-making in biodiversity conservation. Techniques such as Markov Decision Processes (MDPs), Partially Observable MDPs (POMDPs), and adaptive management allow us to plan interventions under uncertainty, account for feedbacks, and learn from unfolding ecological dynamics. These methods have been applied to problems from invasive species control to threatened species recovery, where they help navigate trade-offs between immediate action and long-term outcomes. But perhaps more important than the algorithms themselves are the lessons learned about developing AI for nature conservation. Reversing the biodiversity extinction crisis demands more than AI technical capability. It requires co-designing research with ecologists, social scientists, land owners, and policymakers: A mindset shift from “what can we build?” to “what does biodiversity actually need?”. This is the mission of the Environmental Informatics Hub at Monash, a new initiative I lead within the Faculty of IT, designed to build AI that supports the future of biodiversity, not just the future of technology. Bio: Iadine Chades is the Director of the Environmental Informatics Hub at the Faculty of Information Technology, Monash University, and Professor of AI in the Department of Data Science and AI. Her research lies at the forefront of linking domain sciences—such as ecology, epidemiology, and social sciences—with advanced quantitative tools from artificial intelligence (AI), developing innovative methods for smart decision-making under uncertainty and resource constraints. Building on her PhD work with Markov decision processes (MDP) applied to mobile robotics, Iadine shifted her focus in 2006 to apply these principles in conservation science by integrating AI with ecological and economic models to solve complex applied problems. |
||
Poster session w/ coffee break | ||
Spotlight talks
Details: (click to expand)Papers:(1) DeepSolar-3M: An AI-Enabled Solar PV Database Documenting 3 Million Systems Across the US (2) Paper HIVEX: A High-Impact Environment Suite for Multi-Agent Research (3) Dargana: fine-tuning EarthPT for dynamic tree canopy mapping from space (4) Wind Power in a Warming World: Diffusion-Based Downscaling of CMIP6 Climate Projections for Future Energy Planning |
||
Panel 1 - Challenges and Opportunities of ML for Climate Action in Asia
Details: (click to expand)Panelists: Karen Wang (Founder and CEO, Climind), James Askew (Chief Development Officer, NatureVerse, Inc.), Dona Mathew (Senior Research Associate, Digital Futures Lab)Topic: While climate change is a global problem, it manifests differently in different areas around the world. In this panel, we focus on climate change challenges in Asia and ask, how AI can support efforts towards climate action. |
||
Lunch break | ||
Panel 2 - Data-Centric Machine Learning in Climate Applications
Details: (click to expand)Panelists: David Robinson (Senior AI Research Scientist, Earth Species Project), Anuj Karpatne (Associate Professor, Virginia Tech), Chen Chen (Senior Research Scientist, Centre for Climate Research Singapore)Topic: Data-centric machine learning refers to the process of working on improving your data rather than designing the best model for some given dataset. In this panel we ask about data-centric ML challenges in the context of climate relevant applications. |
||
Spotlight talks
Details: (click to expand)Papers:(1) WeatherMesh-3: Fast and accurate operational global weather forecasting (2) Multi-resolution Score-Based Variational Graphical Diffusion for Causal Disaster System Modeling and Inference (3) Atlantes: A system of GPS transformers for global-scale real-time maritime intelligence (4) 5D Neural Surrogates for Nonlinear Gyrokinetic Simulations of Plasma Turbulence |
||
Poster session w/ coffee break | ||
Keynote Talk - Dr. Yisong Yue
Details: (click to expand)Title: AI for Adaptive Experiment DesignAbstract: Experiment design is a hallmark of virtually all research disciplines. In many settings, one important challenge is how to automatically design experiments over large action/design spaces. Furthermore, it is also important for such a procedure to be adaptive, i.e., to adapt to the outcomes of previous experiments. In this talk, I will describe recent progress from my group & collaborators in using data-driven algorithmic techniques for adaptive experiment design. I will close with some thoughts for the future. Bio: Yisong Yue is a Professor of Computing and Mathematical Sciences at the California Institute of Technology. He was previously a research scientist at Disney Research. Before that, he was a postdoctoral researcher in the Machine Learning Department and the iLab at Carnegie Mellon University. He received a Ph.D. from Cornell University and a B.S. from the University of Illinois at Urbana-Champaign. Yisong is also the General Chair of ICLR 2025 (International Conference on Learning Representations). Yisong's research interests are centered around machine learning, and in particular getting theory to work in practice. To that end, his research agenda spans both fundamental and applied pursuits, from novel learning-theoretic frameworks all the way to deployment in autonomous driving on public roads. His work has been recognized with multiple paper awards and nominations, including in robotics, computer vision, sports analytics, machine learning for health, and information retrieval. During his time in industry, Yisong worked on machine learning approaches to behavior modeling and motion planning for autonomous driving. |
||
Closing Remarks
Details: (click to expand)Closing remarks and awards announcement |
Speakers
Keynotes
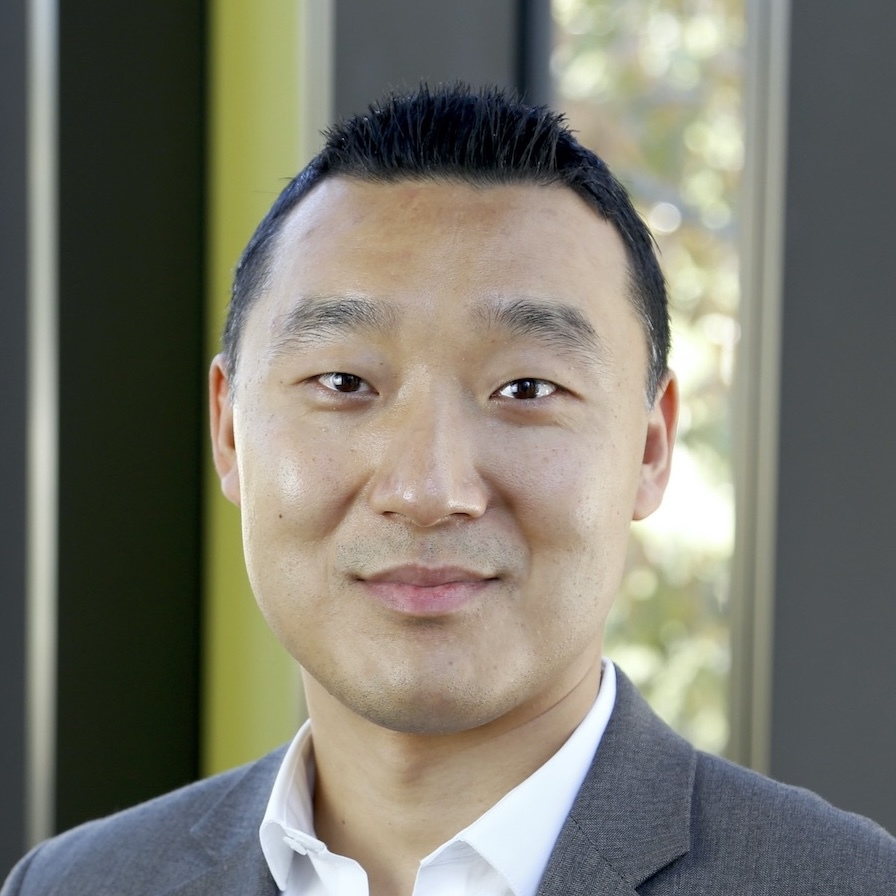
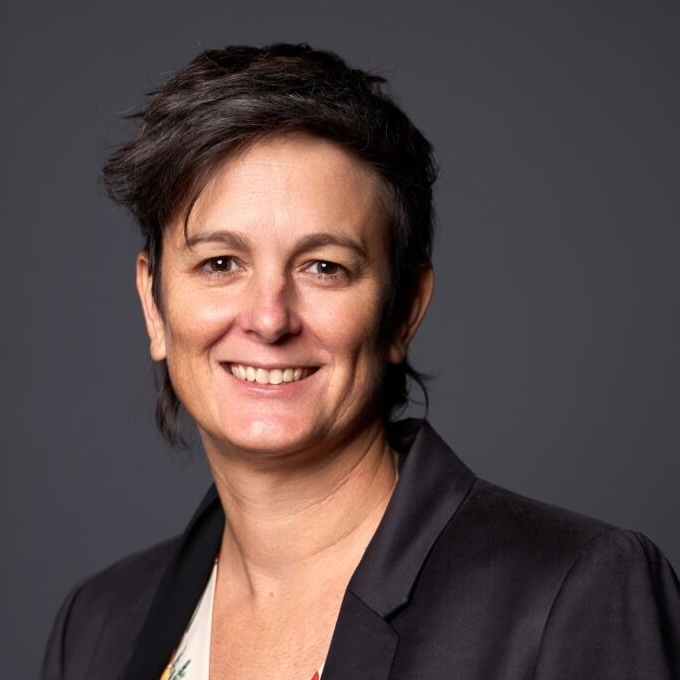
Panelists
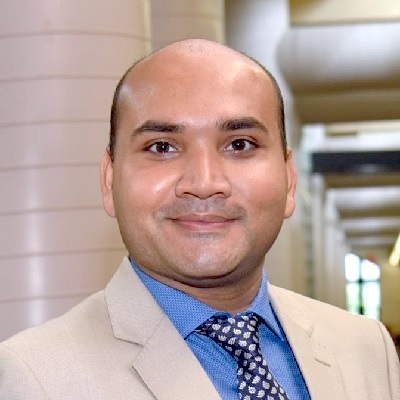
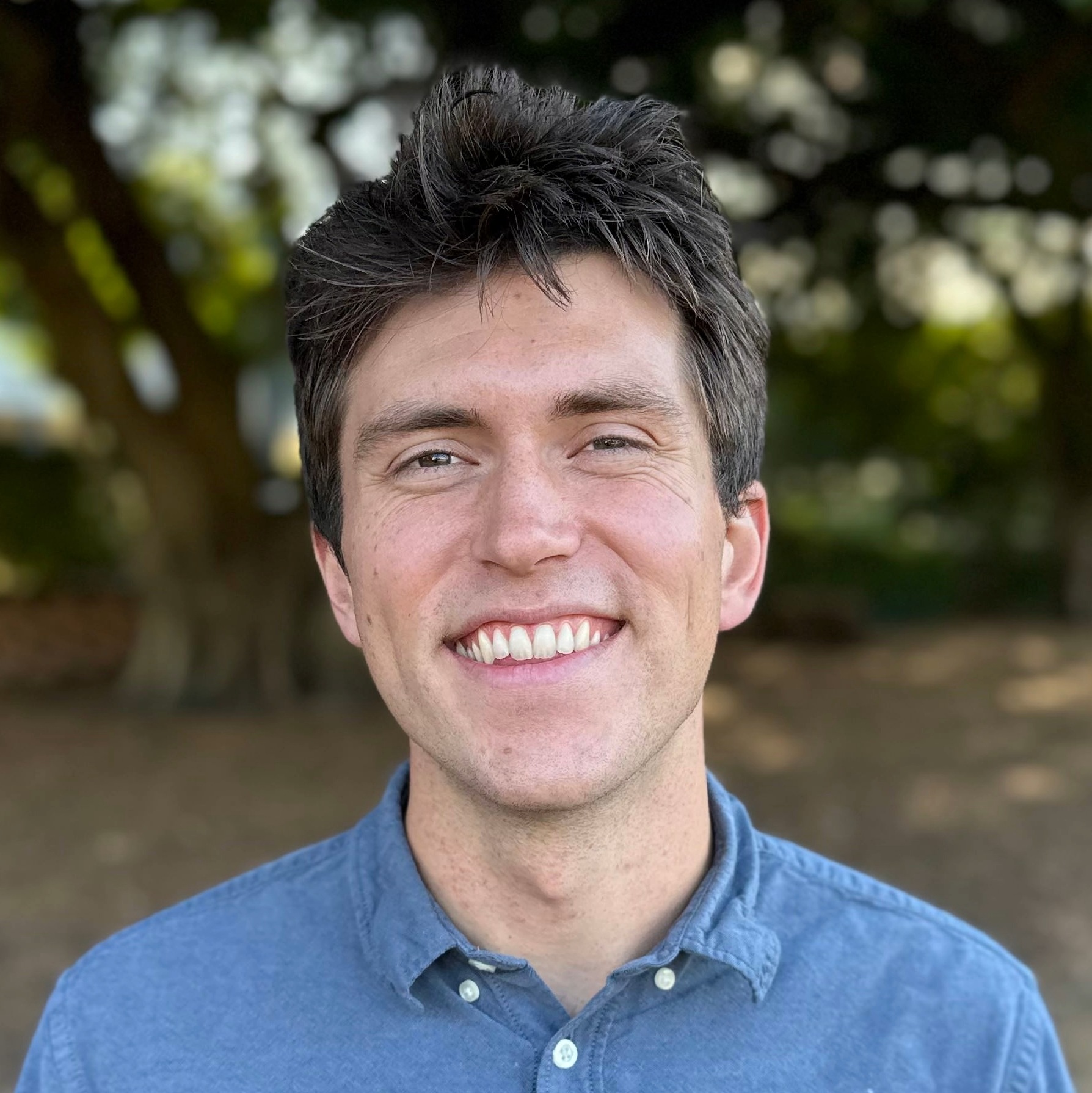
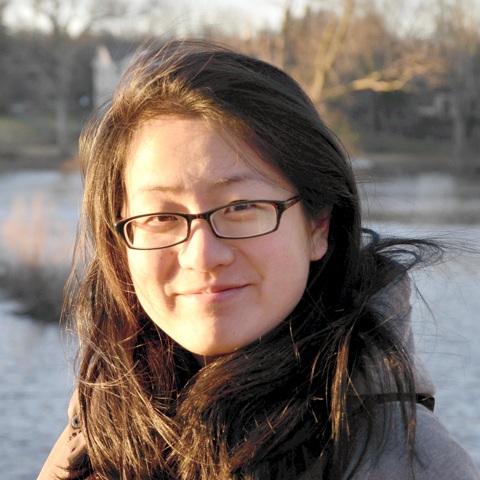
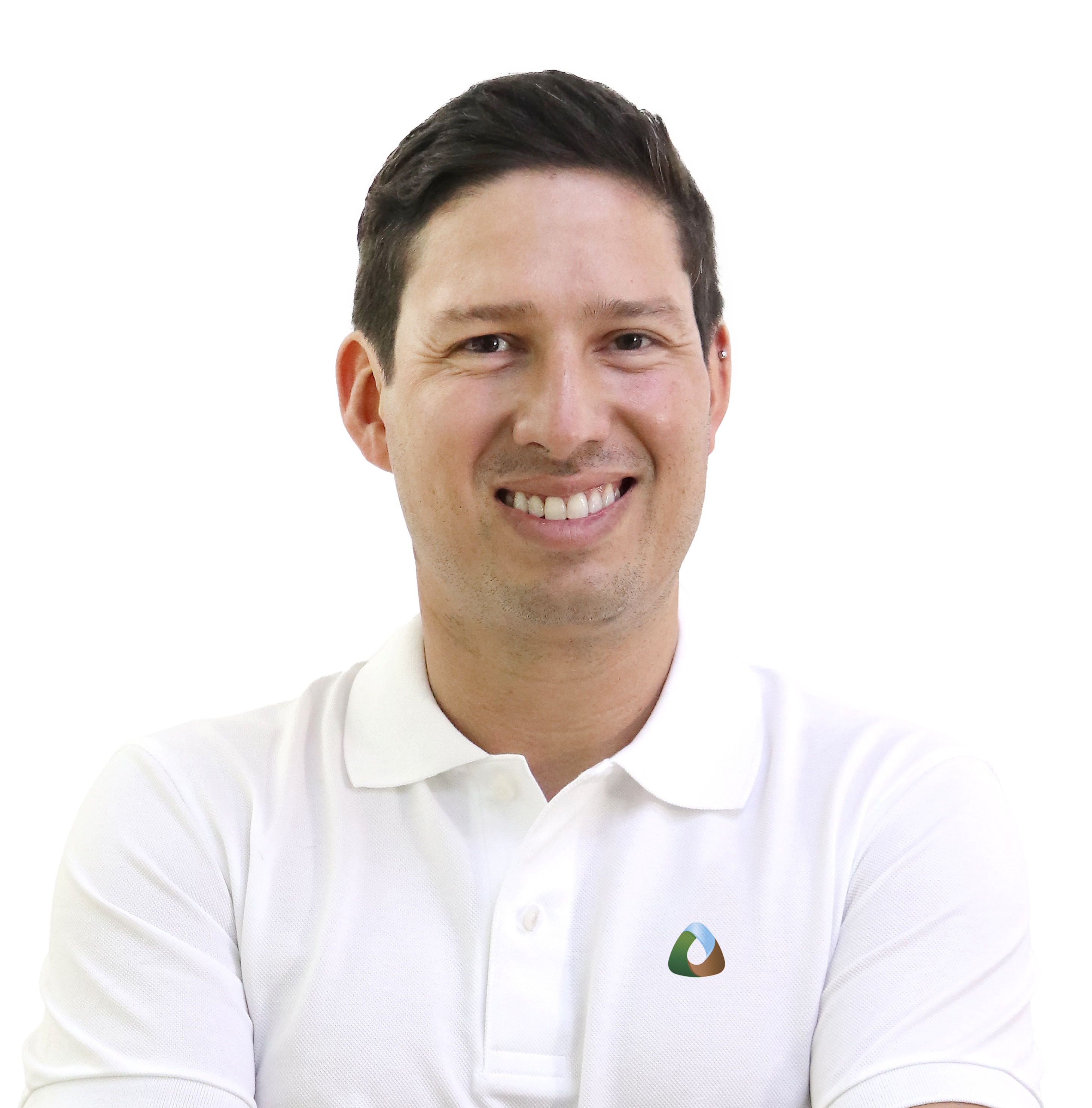
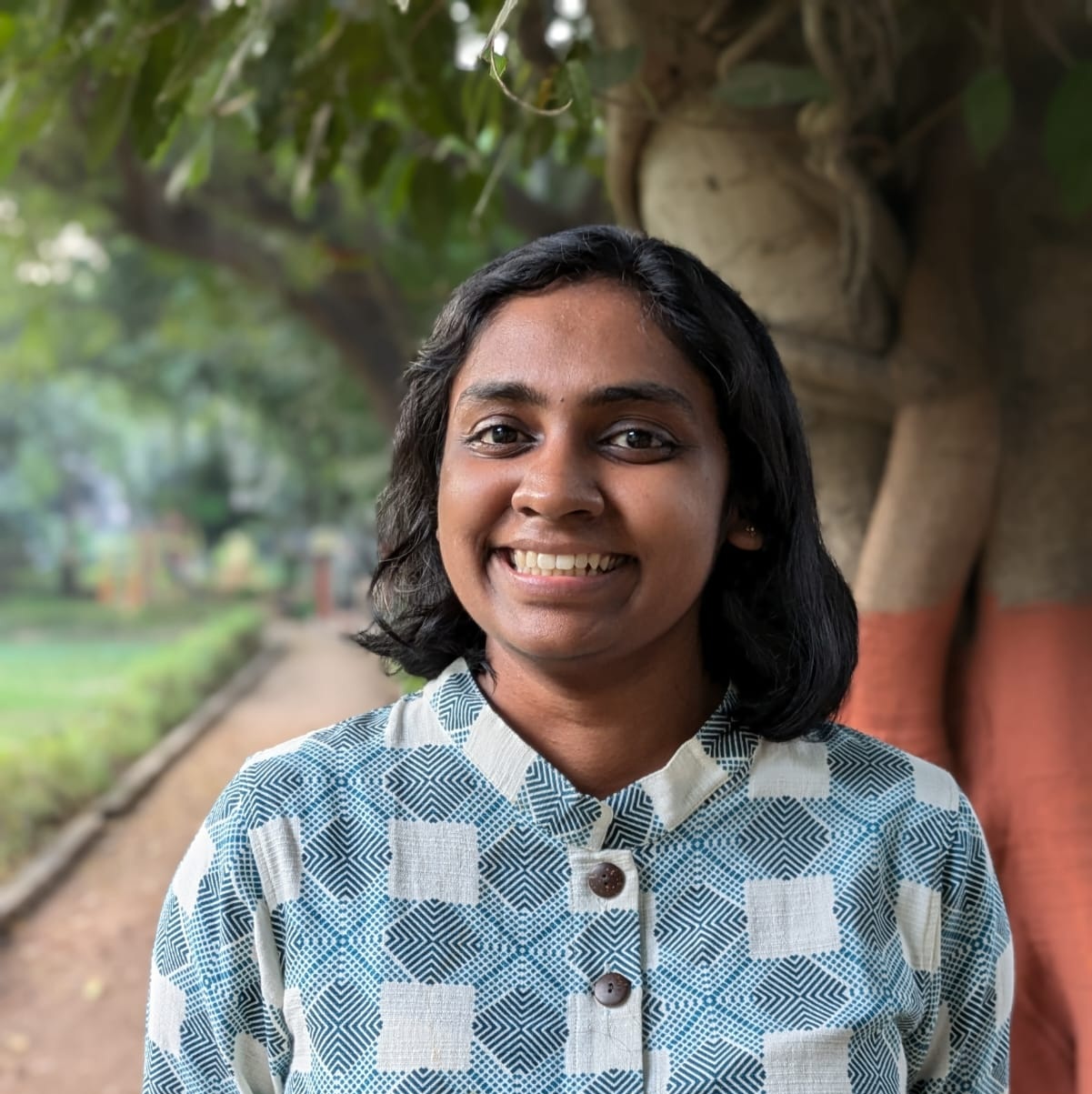
Accepted Works
Works were submitted to one of two tracks: Papers or Proposals.
Click the links below for information about each submission, including slides and papers.
Papers
Title | Authors |
---|---|
(1) Deep Reinforcement Learning for Power Grid Multi-Stage Cascading Failure Mitigation | Bo Meng (Sun Yat-sen University); Chenghao Xu (Sun Yat-sen University); Yongli Zhu (Sun Yat-sen University) |
(2) ClimateChat: Designing Data and Methods for Instruction Tuning LLMs to Answer Climate Change Queries | zhou chen (Tsinghua University); Xiao Wang (Tsinghua University); Liao Yuanhong (Tsinghua University); Ming Lin (Tsinghua University); Yuqi Bai (Tsinghua University) |
(3) ExioNAICS: Enterprises Level Emission Estimation Dataset with Large Language Models | Yanming Guo (University of Sydney); Jin Ma (University of Sydney); Qiao Xiao (Maynooth University); Kevin Credit (Maynooth University) |
(4) Improving Tropical Cyclone Forecasting With Video Diffusion Models | Zhibo Ren (Imperial College London); Pritthijit Nath (University Of Cambridge); Pancham Shukla (Imperial College London) |
(5) A hackathon for flood map prediction from geospatial data with parsimonious machine learning models | David Medernach (Capgemini); Cyril Lemaire (Capgemini); Eva Girousse (EDF); Julie Keisler (EDF); Julie Richon (Capgemini); Nicolas Brunel (ENSIIE) |
(6) Comparing Spatial Interpolation Methods for PM2.5 as Inputs to Urban Decision-Making in Greater Boston | SooMi Lee (Seoul National University); Stratis Ioannidis (Northeastern University); Amy Mueller (Northeastern University) |
(7) FabAgent: An LLM-based Agentic Optimization Framework for Design of Sustainable Fabrics | Anusha Narayan (The Nueva School) |
(8) Flood Detection Modeling: Leveraging The SEN1FLOOD11 Dataset For The Rio Colima River | Bilal Sardar (Anglia Ruskin University); Lakshmi Saheer (Anglia Ruskin University) |
(9) Causal Disaster System Modeling and Inference with Multi-resolution Score-Based Variational Graphical Diffusion | Xuechun Li (Johns Hopkins University); Shan Gao (Johns Hopkins University); Susu Xu (Johns Hopkins University) |
(10) Predicting extreme weather impacts on physical activity and sleep patterns using real-world data from wrist-worn accelerometers | Sara khalid (University of Oxford) |
(11) Dargana: fine-tuning EarthPT for dynamic tree canopy mapping from space | Michael Smith (Aspia Space); Luke Fleming (Aspia Space); James Geach (Aspia Space); Ryan Roberts (Aspia Space); Freddie Kalaitzis (Aspia Space); James Banister (Aspia Space) |
(12) Conditional Diffusion-Based Retrieval of Atmospheric CO2 from Earth Observing Spectroscopy | William Keely (The University of Oklahoma) |
(13) HIVEX: A High-Impact Environment Suite for Multi-Agent Research | Philipp Siedler (Aleph Alpha Research) |
(14) Learning Enhanced Structural Representations with Block-Based Uncertainties for Ocean Floor Mapping | Jose Marie Antonio Minoza (Center for AI Research) |
(15) Uncertainty-Aware Carbon Flux Estimation from Multispectral Landsat Imagery Using Mixture Density Networks | Anish Dulal (University of Oregon); Jake Searcy (University of Oregon) |
(16) A multimodal attention-based model for tree species classification using LiDAR and satellite imagery | Hadrien Sablon (PG&E); Rajen Bajgain (PG&E) |
(17) Balancing quantity and representativeness in constrained geospatial dataset design | Livia Betti (University of Colorado at Boulder); Esther Rolf (CU Boulder) |
(18) Generative Adversarial Networks for Unsupervised Anomaly Detection in Energy Time series data | Praveen Prasad Handigol (Indian Institute of Science, Bangalore); Pandarasamy Arjunan (Indian Institute of Science, Bangalore) |
(19) Improving Local Air Quality Predictions Using Transfer Learning on Satellite Data and Graph Neural Networks | Finn Gueterbock (University of Bristol); Raul Santos-Rodriguez (University of Bristol); Jeff Clark (University of Bristol) |
(20) Predicting out-of-domain performance under geographic distribution shifts | Haoran Zhang (Harvard University); Konstantin Klemmer (Microsoft Research); Esther Rolf (University of Colorado, Boulder); David Alvarez-Melis (Harvard University) |
(21) 5D Neural Surrogates for Nonlinear Gyrokinetic Simulations of Plasma Turbulence | Gianluca Galletti (ELLIS Unit Linz, LIT AI Lab, Institute for Machine Learning, Johannes Kepler University Linz, Austria); Fabian Paischer (ELLIS Unit Linz, LIT AI Lab, Institute for Machine Learning, Johannes Kepler University Linz, Austria); Paul Setinek (ELLIS Unit Linz, LIT AI Lab, Institute for Machine Learning, Johannes Kepler University Linz, Austria); William Hornsby (UKAEA, Culham Centre for Fusion Energy, Abingdon, UK); Lotenzo Zanisi (UKAEA, Culham Centre for Fusion Energy, Abingdon, UK); Naomi Carey (UKAEA, Culham Centre for Fusion Energy, Abingdon, UK); Stanislas Pamela (UKAEA, Culham Centre for Fusion Energy, Abingdon, UK); Johannes Brandstetter (ELLIS Unit Linz, LIT AI Lab, Institute for Machine Learning, Johannes Kepler University Linz, NXAI GmbH, Austria) |
(22) Learning Extreme Temperature Regimes | Shirin Goshtasbpour (SDSC); Maxim Samarin (SDSC, ETH Zurich and EPFL); Michele Volpi (SDSC, ETH Zurich and EPFL) |
(23) Deep Neural Network Framework for Inverting Remotely Sensed CO2 Measurements | Garvit Agarwal (IISER, Pune); shailesh deshpande (Tata Research Development and Design Centre, Tata Consultancy Services) |
(24) AI-Driven Sub-seasonal Landslide Forecasting in Nepal for Disaster Preparedness | Kelsey Doerksen (University of Oxford); Sihan Li (Sheffield University); Yarin Gal (University of Oxford); Freddie Kalaitzis (Aspia Space); Alexander Densmore (Durham University); Alexandre Dunant (Eurac Research); Nick Rosser (Durham University); Simon Dadson (University of Oxford) |
(25) A Joint Space-Time Encoder for Geographic Time-Series Data | David Mickisch (Mila); David Rolnick (McGill, Mila); Konstantin Klemmer (Microsoft Research); Mélisande Teng (Université de Montréal, Mila) |
(26) DiffScale: Continuous Downscaling and Bias Correction in Subseasonal Wind Forecasts | Maximilian Springenberg (Fraunhofer HHI); Noelia Otero Felipe (Fraunhofer HHI); Yuxin Xue (Fraunhofer HHI); Jackie Ma (Fraunhofer HHI) |
(27) A Synthetic Dataset of French Electric Load Curves With Temperature Conditioning | Tahar Nabil (EDF R&D); Ghislain Agoua (EDF R&D); Pierre Cauchois (Enedis); Anne de Moliner (Enedis); Benoît Grossin (EDF R&D) |
(28) GreenScreen: Automatic Accessible Presentation Generation from IPCC Reports | Alice Heiman (Stanford University); Komal Vij (Stanford University); Anjali Sreenivas (Stanford University) |
(29) What can large language models do for sustainable food? | Anna Thomas (Stanford University); Adam Yee (Umai Works); Andrew Mayne (Stanford); Maya Mathur (Stanford); Dan Jurafsky (Stanford); Kristina Gligorić (Stanford) |
(30) Large Language Models as a New Modality for Generalizable Earth Data Monitoring | Tong Nie (Tongji University); Junlin He (The Hong Kong Polytechnic University); Wei Ma (The Hong Kong Polytechnic University) |
(31) Drought forecasting using a hybrid neural architecture for integrating time series and static data | Julian Agudelo (AgroParisTech); Vincent Guigue (AgroParisTech); Cristina Manfredotti (AgroParisTech); Hadrien Piot (Agrial) |
(32) Alberta Wells Dataset: Pinpointing Oil and Gas Wells from Satellite Imagery | Pratinav Seth (Arya.ai); Michelle Lin (Mila - Quebec Artificial Intelligence Institute); Brefo Dwamena Yaw (Aya data); Jade Boutot (McGill University); Mary Kang (McGill University); David Rolnick (McGill University) |
(33) Improving Contrail Detection via Diffusion-Based Data Augmentation Framework | Yejun Lee (UNIST); Jaejun Yoo (UNIST) |
(34) Generalizable Implicit Neural Representations via Parameterized Latent Dynamics for Baroclinic Ocean Forecasting | Guang Zhao (Brookhaven National Lab); Xihaier Luo (Brookhaven National Lab); Seungjun Lee (Brookhaven National Lab); Yihui Ren (Brookhaven National Lab); Shinjae Yoo (Brookhaven National Lab); Luke Van Roekel (Los Alamos National Laboratory); Balu Nadiga (Los Alamos National Laboratory); Sri Hari Krishna Narayanan (Argonne National Laboratory); Yixuan Sun (Argonne National Laboratory); Wei Xu (Brookhaven National Lab) |
(35) XAI4Extremes: An interpretable machine learning framework for understanding extreme-weather precursors under climate change | Jiawen Wei (National University of Singapore); Aniruddha Bora (Brown University); Vivek Oommen (Brown University); Chenyu Dong (National University of Singapore); Juntao Yang (NVIDIA AI Technology Centre); Jeff Adie (NVIDIA AI Technology Centre); Chen Chen (Centre for Climate Research Singapore); Simon See (NVIDIA AI Technology Centre); George Karniadakis (Brown University); Gianmarco Mengaldo (National University of Singapore) |
(36) Diffusion-LAM: Probabilistic Limited Area Weather Forecasting with Diffusion | Erik Larsson (Linköping University); Joel Oskarsson (Linköping University); Tomas Landelius (SMHI); Fredrik Lindsten (Linköping University) |
(37) Towards the Curation of Environment-related Knowledge Graphs: Fine-tuning General-domain Language Models for Biodiversity Named Entity Recognition | Geilah Tabanao (University of the Philippines Diliman); Andrew Miguel Pagdanganan (University of the Philippines Diliman); Riza Batista-Navarro (University of Manchester); Roselyn Gabud (University of the Philippines Diliman) |
(38) Graph Neural Networks for Enhancing Ensemble Forecasts of Extreme Rainfall | Christopher Bülte (LMU Munich); Sohir Maskey (LMU Munich); Philipp Scholl (LMU Munich); Jonas von Berg (LMU Munich); Gitta Kutyniok (LMU Munich) |
(39) Offline Reinforcement Learning for Microgrid Voltage Regulation | Shan Yang (Sun Yat-sen University); Yongli Zhu (Sun Yat-sen University) |
(40) Real-Time Risky Fault-Chain Search using Time-Varying Graph RNNs | Anmol Dwivedi (RPI); Ali Tajer (RPI) |
(41) Machine Learning and Bayesian Method For Monitoring and Forecasting Jayawijaya’s Tropical Glacier Change | Muhamad Iqbal Januadi Putra (Universitas Siber Asia); Stuart Phinn (University of Queensland) |
(42) Earth Observation Foundation Models for region-specific flood segmentation | Helen Tamura-Wicks (IBM Research); Geoffrey Dawson (IBM Research); Andrew Taylor (Science and Technology Facilities Council); Chris Dearden (Science and Technology Facilities Council); Anne Jones (IBM Research); Paolo Fraccaro (IBM Research) |
(43) Prototype enhanced prediction in graph neural networks for climate applications | Nawid Keshtmand (University of Bristol); Elena Fillola Mayoral (University of Bristol); Jeffrey Clark (University of Bristol); Raul Santos-Rodriguez (University of Bristol); Matthew Rigby (University of Bristol) |
(44) Climplicit: Climatic Implicit Embeddings for Global Ecological Tasks | Johannes Dollinger (University of Zurich); Damien Robert (University of Zurich); Elena Plekhanova (Swiss Federal Research Institute WSL); Lukas Drees (University of Zurich); Jan Dirk Wegner (University of Zurich) |
(45) Deep Vision-Based Framework for Coastal Flood Prediction Under Climate Change Impacts and Shoreline Adaptations | Areg Karapetyan (NYU Abu Dhabi); Aaron C.H. Chow (NYUAD); Samer Madanat (NYUAD) |
(46) Towards Flood Extent Forecasting: Evaluating a Weather Foundation Model and U-Net for Flood Forecasting. | Eric Wanjau (UCL); Samuel Maina (Microsoft) |
(47) Palimpsest: Bill of Materials Prediction - A Case Study with Solid State Drives | Anran Wang (Amazon); Zaid Thanawala (Amazon); Harsh Gupta (Amazon); Jeremie Hakian (Amazon); Jared Kramer (Amazon); Kommy Weldemariam (Amazon); Bharathan Balaji (Amazon) |
(48) Lake Water Temperature Modeling Using Physics-Informed Neural Networks | Trieu Vo (Florida International University); Cuong Nguyen (Durham University); Dongsheng Luo (Florida International University); Leonardo Bobadilla (Florida International University) |
(49) Uncertainty-Aware Deep Learning Framework for Forecasting Coastal Water Level in Virginia Beach | Md Mahmudul Hasan (Thomas Jefferson National Accelerator Facility); Malachi Schram (Thomas Jefferson National Accelerator Facility); Sridhar Katragadda (City of Virginia Beach); Diana McSpadden (Thomas Jefferson National Accelerator Facility); Alisa N. Udomvisawakul (City of Virginia Beach); Heather Richter (Old Dominion University); Frank Liu (Old Dominion University) |
(50) Fine Flood Forecasts: Incorporating local data into global models through fine-tuning | Emil Ryd (University of Oxford); Grey Nearing (Google) |
(51) Learning to generate physical ocean states: Towards hybrid climate modeling | Etienne Meunier (Inria, Paris); David Kamm (IPSL); Guillaume Gachon (IPSL); Redouane Lguensat (IPSL); Julie Deshayes (IPSL) |
(52) CLIMGEN: Learning the Forcing-Response Relationship in Climate System | Tse-Chun Chen (Pacific Northwest National Laboratory); Parvathi Kooloth (Pacific Northwest National Laboratory); Jian Lu (Pacific Northwest National Laboratory); Jason Z. Hou (Pacific Northwest National Laboratory) |
(53) Geo-Semantics Analysis of Environmental Disasters in Nigeria Using National Print Media Data for Disaster Management | Benedict Ajanaku (Data Science Nigeria); Rashidat Sikiru (Data Science Nigeria); Anthony Soronnadi (Data Science Nigeria); Ife Adebara (Data Science Nigeria); Olubayo Adekanmbi (Data Science Nigeria AI (DSNai)) |
(54) Wind Power in a Warming World: Diffusion-Based Downscaling of CMIP6 Climate Projections for Future Energy Planning | Michael Sierks (Sust Global); Tristan Ballard (Sust Global); Gopal Erinjippurath (Sust Global) |
(55) DeepSolar-3M: An AI-Enabled Solar PV Database Documenting 3 Million Systems Across the US | Rajanie Prabha (Stanford University); Zhecheng Wang (Stanford University); Chad Zanocco (Stanford University); June Flora (Stanford University); Ram Rajagopal (Stanford University) |
(56) Exploring Design Choices for Autoregressive Deep Learning Climate Models | Florian Gallusser (Universität Würzburg); Simon Hentschel (Universität Würzburg); Anna Krause (Universität Würzburg); Andreas Hotho (Universität Würzburg) |
(57) Multivariate LSTM-Based Forecasting for Renewable Energy: Enhancing Climate Change Mitigation | Farshid Kamrani (Carleton University); Kristen Schell (Carleton University) |
(58) Atlantes: A system of GPS transformers for global-scale real-time maritime intelligence | Henry Herzog (Allen Institute for AI) |
(59) End-to-end electricity system forecasting via approximate message passing | Anthony Degleris (Stanford University); Akshay Sreekumar (Stanford University); Kamran Tehranchi (Stanford University); Ram Rajagopal (Stanford University) |
(60) Adaptive Dice Loss for Extremely Imbalanced Segmentation in Wetland Delineation | Sipeng Chen (Florida International University); Xu Zheng (Florida International University); Zeda Yin (Florida International University); Qiang Chen (Florida International University); Yuepeng Li (Florida International University); Jason Liu (Florida International University); Dongsheng Luo (Florida International University) |
(61) Populous: A Multimodal Geospatial AI Model for Understanding the Climate-Driven Insurance Crisis in the U.S. | Tristan Ballard (Sust Global); Gopal Erinjippurath (Sust Global); Michael Sierks (Sust Global); Peter Sousounis (Sust Global) |
(62) Enhancing Grid Resilience: Probabilistic Modeling of Gas-Fired Generator Capacity during Extreme Winter Weather | Sajjad Uddin Mahmud (Washington State University); Anamika Dubey (Washington State University) |
(63) Large Language Models for Monitoring Dataset Mentions in Climate Research | Aivin Solatorio (The World Bank); Rafael Macalaba (The World Bank); James Liounis (The World Bank) |
(64) WeatherMesh-3: Fast and accurate operational global weather forecasting | Lyna Kim (WindBorne Systems); Haoxing Du (WindBorne Systems) |
(65) Extending Two Explainable Artificial Intelligence Methods for Deep Climate Emulators | Wei Xu (Brookhaven National Laboratory); Rui Qiu (The Ohio State University); Xihaier Luo (Brookhaven National Laboratory); Yihui Ren (Brookhaven National Laboratory); Balu Nadiga (Los Alamos National Laboratory); Luke Van Roekel (Los Alamos National Laboratory); Han-Wei Shen (The Ohio State University); Shinjae Yoo (Brookhaven National Laboratory) |
(66) Heterogenous graph neural networks for species distribution modeling | Lauren Harrell (Google Research); Christine Kaeser-Chen (Google DeepMind); Burcu Karagol Ayan (Google DeepMind); Keith Anderson (Google DeepMind); Michelangelo Conserva (Google Research); Elise Kleeman (Google Research); Maxim Neumann (Google DeepMind); Matt Overlan (Google DeepMind); Melissa Chapman (Google Research); Drew Purves (Google DeepMind) |
(67) PF∆: A Benchmark Dataset for Power Flow With Load, Generator, & Topology Variations | Anvita Bhagavathula (Massachusetts Institute of Technology); Alvaro Carbonero (Massachusetts Institute of Technology); Ana Rivera (Massachusetts Institute of Technology); Priya Donti (Massachusetts Institute of Technology) |
(68) Segregation and Context Aggregation Network for Real-time Cloud Segmentation | Yijie Li (Carnegie Mellon University); Hewei Wang (Carnegie Mellon University); Jiayi Zhang (University of Nottingham); Jinjiang You (Carnegie Mellon University); Jinfeng Xu (The University of Hong Kong); Puzhen Wu (Cornell University); Yunzhong Xiao (Carnegie Mellon University); Soumyabrata Dev (University College Dublin) |
(69) Using multiple input modalities can improve data-efficiency for ML with satellite imagery | Arjun Rao (The University of Colorado Boulder); Esther Rolf (The University of Colorado Boulder) |
Proposals
Title | Authors |
---|---|
(70) Using Reinforcement Learning to Integrate Subjective Wellbeing into Climate Adaptation Decision Making | Arthur Vandervoort (Technical University of Denmark); Miguel Costa (Technical University of Denmark); Morten W. Petersen (Technical University of Denmark); Martin Drews (Technical University of Denmark); Sonja Haustein (Technical University of Denmark); Karyn Morrissey (University of Galway); Francisco C. Pereira (Technical University of Denmark) |
(71) Predicting Concurrence of Heatwaves, Droughts, and Wildfires with Spatiotemporal Deep Learning | Ana Trisovic (MIT) |
(72) Tracking ESG Disclosures of European Companies with Retrieval-Augmented Generation | Kerstin Forster (LMU Munich & Munich Center for Machine Learning); Victor Wagner (LMU Munich & Sustainability Reporting Navigator); Lucas Elias Keil (University of Cologne & Sustainability Reporting Navigator); Maximilian A. Müller (University of Cologne & Sustainability Reporting Navigator); Thorsten Sellhorn (LMU Munich & Sustainability Reporting Navigator); Stefan Feuerriegel (LMU Munich & Munich Center for Machine Learning) |
(73) Leveraging Geospatial Foundation Model to estimate Aboveground Biomass and studying it's effect on forest temperature | Arnav Goel (Purdue University); Gaia Cervini (Purdue University); Jinha Jung (Purdue University); Songlin Fei (Purdue University) |
(74) Evaluating the Environmental Impact of Language Models with Life Cycle Assessment | Jared Fernandez (Carnegie Mellon University); Clara Na (Carnegie Mellon University); Yonatan Bisk (Carnegie Mellon University); Emma Strubell (Carnegie Mellon University) |
(75) SuoiAI: Building a Dataset for Aquatic Invertebrates in Vietnam | Minh Teu Vo Thanh (NUOC SOLUTIONS); Lakshay Sharma (Microsoft); Tuan Dinh (NUOC SOLUTIONS); Khuong Dinh (University of Oslo); Trang Nguyen (Bowdoin); Trung Phan (Fulbright University Vietnam); Minh Do (NUOC SOLUTIONS); Duong Vu (Royal Netherlands Academy of Arts and Sciences) |
(76) Using street view imagery and deep generative modeling for estimating the health of urban forests | Akshit Gupta (Delft University of Technology); Remko Uijlenhoet (TU Delft) |
(77) From Rumors to Risk: Mapping and Modeling Climate-Disaster Misinformation | Tristan Ballard (Independent) |
(78) Dynamic Ensemble Models for Climate-Driven Epidemic Prediction | Jinpyo Hong (Brown University); Rachel Baker (Brown University) |
(79) Developing an urban temperature emulator using physics-based climate models and machine learning | Minn Lin Wong (Singapore-ETH Centre); Manmeet Singh (Jackson School of Geosciences, The University of Texas) |
(80) Modelling the Doughnut of social and planetary boundaries with machine learning | Stefano Vrizzi (Ecole Normale Superieure); Daniel O'Neill (Universitat de Barcelona) |
(81) Learning Subseasonal-to-Seasonal Global Ocean Forecasting on a Hierarchical Triangle Mesh | Stefano Campanella (OGS); Stefano Piani (OGS); Stefano Querin (OGS); Stefano Salon (OGS); Luca Bortolussi (University of Trieste); Jason Stock (Argonne National Laboratory) |
CCAIxFCL Networking Event
Are you interested in further connecting with the AI for climate action community? Together with FCL, we are organizing a networking event on Friday, Apr 25 in Singapore. Learn more about the event and register (for free) here.
Call for Submissions
We invite submissions of short papers using machine learning to address problems in climate mitigation, adaptation, or science, including but not limited to the following topics:
- Agriculture and food
- Behavioural and social science
- Buildings
- Carbon capture and sequestration
- Cities and urban planning
- Climate finance and economics
- Climate justice
- Climate science and climate modeling
- Disaster management and relief
- Earth observations and monitoring
- Earth science
- Ecosystems and biodiversity
- Extreme weather
- Forestry and other land use
- Health
- Heavy industry and manufacturing
- Local and indigenous knowledge systems
- Materials science and discovery
- Oceans and marine systems
- Power and energy systems
- Public policy
- Societal adaptation and resilience
- Supply chains
- Transportation
All machine learning techniques are welcome, from kernel methods to deep learning. Each submission should make clear why the application has (or could have) a pathway to positive impacts regarding climate change. We highly encourage submissions which make their data and code publicly available. Accepted submissions will be invited to give poster presentations, of which some will be selected for spotlight talks.
The workshop does not publish proceedings, and submissions are non-archival. Submission to this workshop does not preclude future publication. Previously published work may be submitted under certain circumstances (see the FAQ). While we encourage in-person attendance, we also aim to provide accomodations for virtual attendance, such as a virtual poster session.
All papers and proposals submissions must be through the submission website. Submissions will be reviewed double-blind; do your best to anonymize your submission, and do not include identifying information for authors in the PDF. Authors are required to use the workshop style template (based on the ICLR style files), available for LaTeX.
Please see the Tips for Submissions and FAQ, and contact climatechangeai.iclr2025@gmail.com with questions.
Submission Tracks
There are two tracks for submissions: (i) Papers and (ii) Proposals. Submissions are limited to 4 pages for the Papers track, and 3 pages for the Proposals track, in PDF format (see examples from previous workshops here). References do not count towards this total. Supplementary appendices are allowed but will be read at the discretion of the reviewers. All submissions must explain why the proposed work has (or could have) positive impacts regarding climate change.
PAPERS Track
Work that is in progress, published, and/or deployed.
Submissions for the Papers track should describe projects relevant to climate change that involve machine learning. These may include (but are not limited to) academic research; deployed results from startups, industry, public institutions, etc.; and climate-relevant datasets.
Submissions should provide experimental or theoretical validation of the method presented, as well as specifying what gap the method fills. Authors should clearly illustrate a pathway to climate impact, i.e., identify the way in which this work fits into broader efforts to address climate change. Algorithms need not be novel from a machine learning perspective if they are applied in a novel setting. Details of methodology need not be revealed if they are proprietary, though transparency is highly encouraged.
Submissions creating novel datasets are welcome. Datasets should be properly documented with regards to their provenance and contents and designed to permit machine learning research (e.g. formatted with clear benchmarks for evaluation).
PROPOSALS Track
Early-stage work and detailed descriptions of ideas for future work.
Submissions for the Proposals track should describe detailed ideas for how machine learning can be used to solve climate-relevant problems. While less constrained than the Papers track, Proposals will be subject to a very high standard of review. Ideas should be justified as extensively as possible, including motivation for why the problem being solved is important in tackling climate change, discussion of why current methods are inadequate, explanation of the proposed method, and discussion of the pathway to climate impact. Preliminary results are optional.
The Proposals track is integrated with ICLR’s “Tiny Papers” track (see here for more details). Authors of these papers will be earmarked for potential funding from ICLR, but need to submit a separate application for Financial Assistance that evaluates their eligibility. This application for Financial Assistance to attend ICLR 2025 is available on the ICLR website and closes on March 2nd.
Tips for Submissions
- For examples of typical formatting and content, see submissions from our previous workshops.
- Be explicit: Describe how your proposed approach addresses climate change, demonstrating an understanding of the application area.
- Frame your work: The specific problem and/or data proposed should be contextualized in terms of prior work.
- Address the impact: Describe the practical implications of your method in addressing the problem you identify, as well as any relevant societal impacts or potential side-effects. We recommend reading our further guidelines on this aspect here.
- Explain the ML: Readers may not be familiar with the exact techniques you are using or may desire further detail.
- Justify the ML: Describe why the ML method involved is needed, and why it is a good match for the problem.
- Avoid jargon: Jargon is sometimes unavoidable but should be minimized. Ideal submissions will be accessible both to an ML audience and to experts in other relevant fields, without the need for field-specific knowledge. Feel free to direct readers to accessible overviews or review articles for background, where it is impossible to include context directly.
Addressing Impact
Tackling climate change requires translating ideas into action. The guidelines below will help you clearly present the importance of your work to a broad audience, hopefully including relevant decision-makers in industry, government, nonprofits, and other areas.
- Illustrate the link: Many types of work, from highly theoretical to deeply applied, can have clear pathways to climate impact. Some links may be direct, such as improving solar forecasting to increase utilization within existing electric grids. Others may take several steps to explain, such as improving computer vision techniques for classifying clouds, which could help climate scientists seeking to understand fundamental climate dynamics.
- Consider your target audience: Try to convey with relative specificity why and to whom solving the problem at hand will be useful. If studying extreme weather prediction, consider how you would communicate your key findings to a government disaster response agency. If analyzing a supply chain optimization pilot program, what are the main takeaways for industries who might adopt this technology? To ensure your work will be impactful, where possible we recommend co-developing projects with relevant stakeholders or reaching out to them early in the process for feedback. We encourage you to use this opportunity to do so!
- Outline key metrics: Quantitative or qualitative assessments of how well your results (or for proposals, anticipated results) compare to existing methods are encouraged. Try to give a sense of the importance of your problem and your findings. We encourage you to convey why the particular metrics you choose are relevant from a climate change perspective. For instance, if you are evaluating your machine learning model on the basis of accuracy, how does improved accuracy on a machine learning model translate to climate impact, and why is accuracy the best metric to use in this context?
- Be clear and concise: The discussion of impact does not need to be lengthy, just clear.
- Convey the big picture: Ultimately, the goal of Climate Change AI is to “empower work that meaningfully addresses the climate crisis.” Try to make sure that from the beginning, you contextualize your method and its impacts in terms of this objective.
Q&A
If you have further questions on how to participate in the workshop, you can also contact us via email at climatechangeai.iclr2025@gmail.com. For recordings of informational webinars of previous editions of our workshop, please see here.
Organizers
Konstantin Klemmer (Microsoft Research)
Melissa Chapman (ETH Zürich)
Lily Xu (University of Oxford)
Ivan Poon (National University of Singapore)
Mélisande Teng (Université de Montréal, Mila)
Patrick Emami (National Renewable Energy Lab)
Binyu Lei (National University of Singapore)
Yoshua Bengio (Mila, Université de Montréal)
Mentorship Program
We are hosting a mentorship program to facilitate exchange between potential workshop submitters and experts working in topic areas relevant to the workshop. The goal of this program is to foster cross-disciplinary collaborations and ultimately increase the quality and potential impact of submitted work.
Expectations
Mentors are expected to guide mentees during the CCAI mentorship program as they prepare submissions for this workshop.
Examples of mentor-mentee interactions may include:
- In-depth discussion of relevant related work in the area of the Paper or Proposal, to ensure submissions are well-framed and contextualized in terms of prior work.
- Iterating on the core idea of a Proposal to ensure that the climate change application is well-posed and the ML techniques used are well-suited.
- Giving feedback on the writing or presentation of a Paper or Proposal to bring it to the right level of maturity for submission.
Mentees are expected to initiate contact with their assigned mentor and put in the work and effort necessary to prepare a Paper or Proposal submission Feb 3.
We suggest that after the mentor-mentee matching is made, a first (physical or digital) meeting should take place within the first week (Jan 10-14) to discuss the Paper or Proposal and set expectations for the mentorship period. Subsequent interactions can take place either through meetings or via email discussions, following the expectations set during the initial meeting, culminating in a final version of a Paper or Proposal submitted via the CMT portal by Feb 3.
Mentors and mentees must abide by the Climate Change AI Code of Conduct: https://www.climatechange.ai/code_of_conduct.
Application
Applications are due by Dec 27, 2024.
-
Application to be a mentee -
Application to be a mentor
Sponsors
Bronze Sponsors
Frequently Asked Questions
Mentorship Program FAQ
Q: Are mentors allowed to be authors on the paper for which they provided mentorship?
A: Yes, mentors can be co-authors but not reviewers.
Q: What happens if the mentor/mentee does not fulfill their duties, or if major issues come up?
A: Please email us at climatechangeai.iclr2025@gmail.com and we will do our best to help resolve the situation. Potential breaches of the Code of Conduct will be responded to promptly as detailed therein.
Q: What happens if I apply to be a mentee but do not get paired with a mentor?
A: While we will do our best, we cannot guarantee pairings for everyone. Even if you do not get paired with a mentor, we encourage you to submit a Paper or Proposal to the workshop, and our reviewers will provide you with guidance and feedback on how to improve it.
Q: What happens if my submission does not get accepted to the workshop?
A: While the mentorship program is meant to give early-career researchers and students the opportunity to improve the quality of their work, sometimes submissions will need further polishing and elaboration before being ready for presentation at a CCAI workshop. If this is the case, we invite you to take into account the comments made by the reviewers and to resubmit again to a subsequent CCAI workshop.
Q: I cannot guarantee that I can commit at least 4 hours to the program over the time period. Should I still apply as a mentor?
A: No. While the 4 hour time commitment is a suggestion, we do believe that it is necessary to ensure that all mentees receive the help and guidance they need.
Q: I do not have a background in machine learning; can I still apply to be a mentor/mentee?
A: Yes! We welcome applications from domains that are complementary to machine learning to solve the problems that we are targeting.
Q: What happens if my mentor/mentee wants to continue meeting after the workshop?
A: We welcome and encourage continued interactions after the official mentorship period. That said, neither the mentor nor the mentee should feel obligated to maintain contact.
Submission FAQ
Q: How can I keep up to date on this kind of stuff?
A: Sign up for our newsletter!
Q: I’m not in machine learning. Can I still submit?
A: Yes, absolutely! We welcome submissions from many fields. Do bear in mind, however, that the majority of attendees of the workshop will have a machine learning background; therefore, other fields should be introduced sufficiently to provide context for the work.
Q: What if my submission is accepted but I can’t attend the workshop?
A: You may ask someone else to present your work in your stead.
Q: It’s hard for me to fit my submission on 3 or 4 pages. What should I do?
A: Feel free to include appendices with additional material (these should be part of the same PDF file as the main submission). Do not, however, put essential material in an appendix, as it will be read at the discretion of the reviewers.
Q: Can I send submissions directly by email?
A: No, please use the CMT website to make submissions.
Q: The submission website is asking for my name. Is this a problem for anonymization?
A: You should fill out your name and other info when asked on the submission website; CMT will keep your submission anonymous to reviewers.
Q: Do submissions for the Proposals track need to have experimental validation?
A: No, although some initial experiments or citation of published results would strengthen your submission.
Q: The submission website never sent me a confirmation email. Is this a problem?
A: No, the CMT system does not send automatic confirmation emails after a submission, though the submission should show up on the CMT page once submitted. If in any doubt regarding the submission process, please contact the organizers. Also please avoid making multiple submissions of the same article to CMT.
Q: Can I submit previously published work to this workshop?
A: Yes, though under limited circumstances. In particular, work that has previously been published at non-machine learning venues may be eligible for submission; however, work that has been published in conferences on machine learning or related fields is likely not eligible. If your work was previously accepted to a Climate Change AI workshop, this work should have changed or matured substantively to be eligible for resubmission. Please contact climatechangeai.iclr2025@gmail.com with any questions.
Q: Can I submit work to this workshop if I am also submitting to another ICLR 2025 workshop?
A: Yes. We cannot, however, guarantee that you will not be expected to present the material at a time that conflicts with the other workshop.
Q: Do I have to attend the workshop in-person?
A: While we encourage in-person attendance, we aim to provide accomodations for virtual attendance, such as a virtual poster session.
Q: Is there financial assistance available?
A: We hope to provide some limited financial assistance to participants from traditionally underserved background. Further financial assistance will be available through ICLR’s “Tiny Papers” initiative (see here).